Large Language Model (LLM) Comparison Between GPT-3 and PaLM-2 to Produce Indonesian Cultural Content
Eastern-European Journal of Enterprise Technologies Information technology Industry control systems(2024)
摘要
Large language models can help to compile content with a cultural theme. However, any information generated by large language models needs to be evaluated to see the truth/fact of the information generated. With many studies discussing the comparison of the capabilities of large language models, there is not much research that directly discusses the comparison of the performance of large language models in producing Indonesian cultural content. This research compares the correctness of the information generated by the large language model using the expert judgment method when creating Indonesian cultural content and its fine-tuning capabilities evaluated using BERTScore. The evaluation method was successfully applied and the results show that in this case, PaLM-2 included less misinformation while GPT-3 excelled in fine-tuning. Using the combination of expert judgment and BERTScore makes it possible to evaluate large language models and obtain additional valid training data to correct deficiencies. The results showed that PaLM-2 produced more valid content with a score of 27 points, while GPT-3 scored 8 points. For training on new datasets/fine-tuning, it was found that the GPT-3 language model was able to learn the dataset more quickly, with a time of 50 minutes and a cost of IDR 27,000, while PaLM-2 took 2 hours 10 minutes and a cost of IDR 1,377,204. For the training dataset evaluation results, GPT-3 is superior with an average of all scores reaching 0.85205. Meanwhile, the PaLM-2 Tuned Model got an average overall score of 0.78942. In this case, the GPT-3 Tuned Model is superior by 8 %. In practice, this method can be used if the assessment is descriptive and requires direct assessment from experts
更多查看译文
AI 理解论文
溯源树
样例
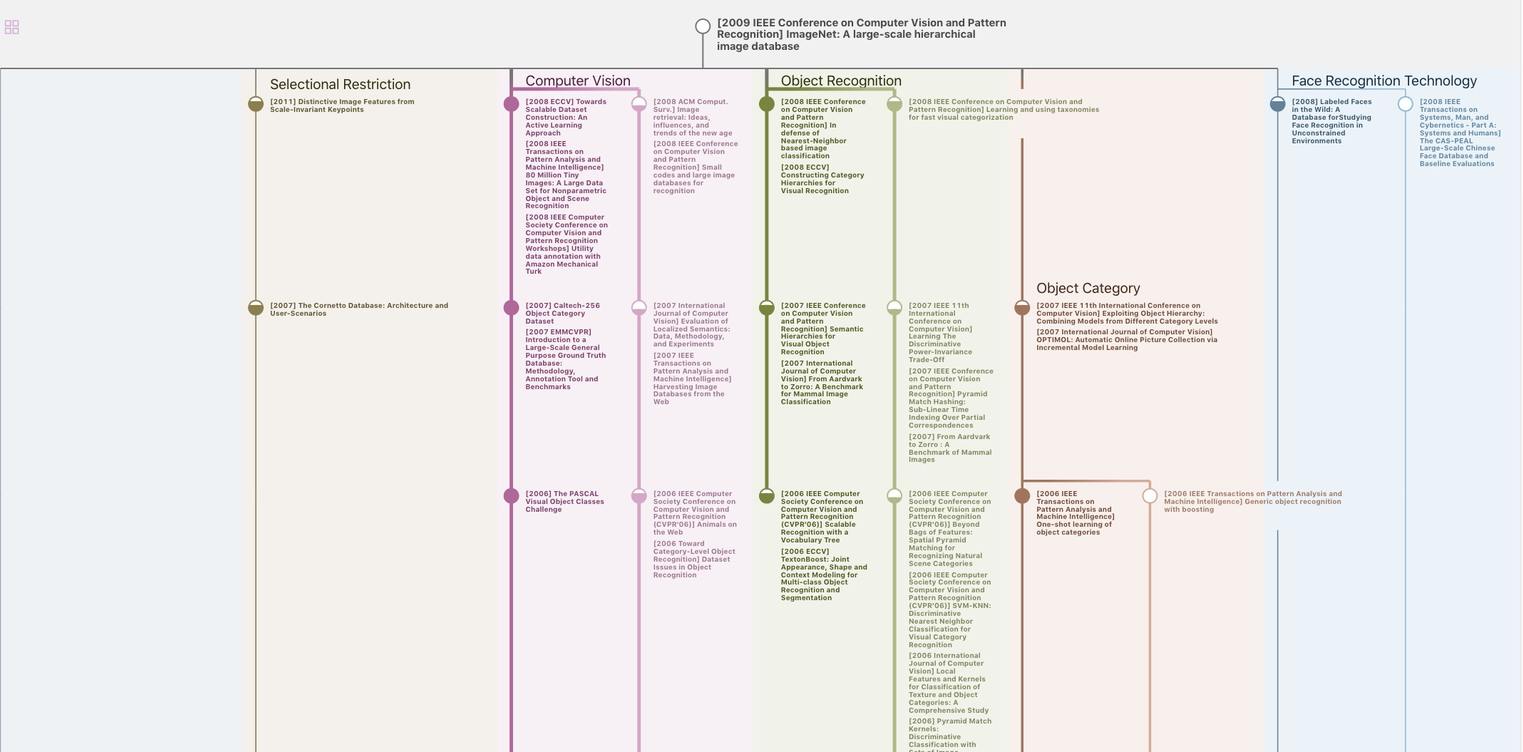
生成溯源树,研究论文发展脉络
Chat Paper
正在生成论文摘要