Integrated Study of Prediction and Optimization Performance of PBI-HTPEM Fuel Cell Using Deep Learning, Machine Learning and Statistical Correlation
Renewable Energy(2024)
摘要
This paper uses 3D modeling and artificial intelligence methods to predict, and find optimal point in high-temperature proton exchange membrane (HTPEM) fuel cells. The main objective is to obtain maximum power and current density at the optimum node of the HTPEM fuel cell. The response surface method (RSM) is used to prevent excessive duplication and ensure adequate data coverage for determining input parameters. Also, For the first time, the correlation presented was compared with AI-based metaheuristic optimization methods i.e., including support vector regression (SVR), Gaussian process regression (GPR), and deep neural networks (DNN) with a dropout layer, alongside metaheuristic algorithms such as whale optimization algorithm (WOA), Grasshopper optimization algorithm (GOA), firefly algorithm (FF), and the genetic algorithm (GA). The results show that SVR, GPR, and DNN methods have excellent performance, with mean absolute percentage error (MAPE) of 0.81% for DNN, 0.83% for SVR, and 2.24% for GPR. Most optimization algorithms exhibit errors below 8%. The DNN-GOA, SVR-WOA, SVR-GA, and GPR-GOA algorithms have the lowest errors among them. Correlations have a lower computational cost for obtaining maximum power and current density at the optimum node compared to optimization algorithms, with a relative error of less than 6% in most cases.
更多查看译文
关键词
High-temperature PEM fuel cell,COMSOL Multiphysics,Artificial Neural Network,Deep Neural Network,Optimization,Correlation
AI 理解论文
溯源树
样例
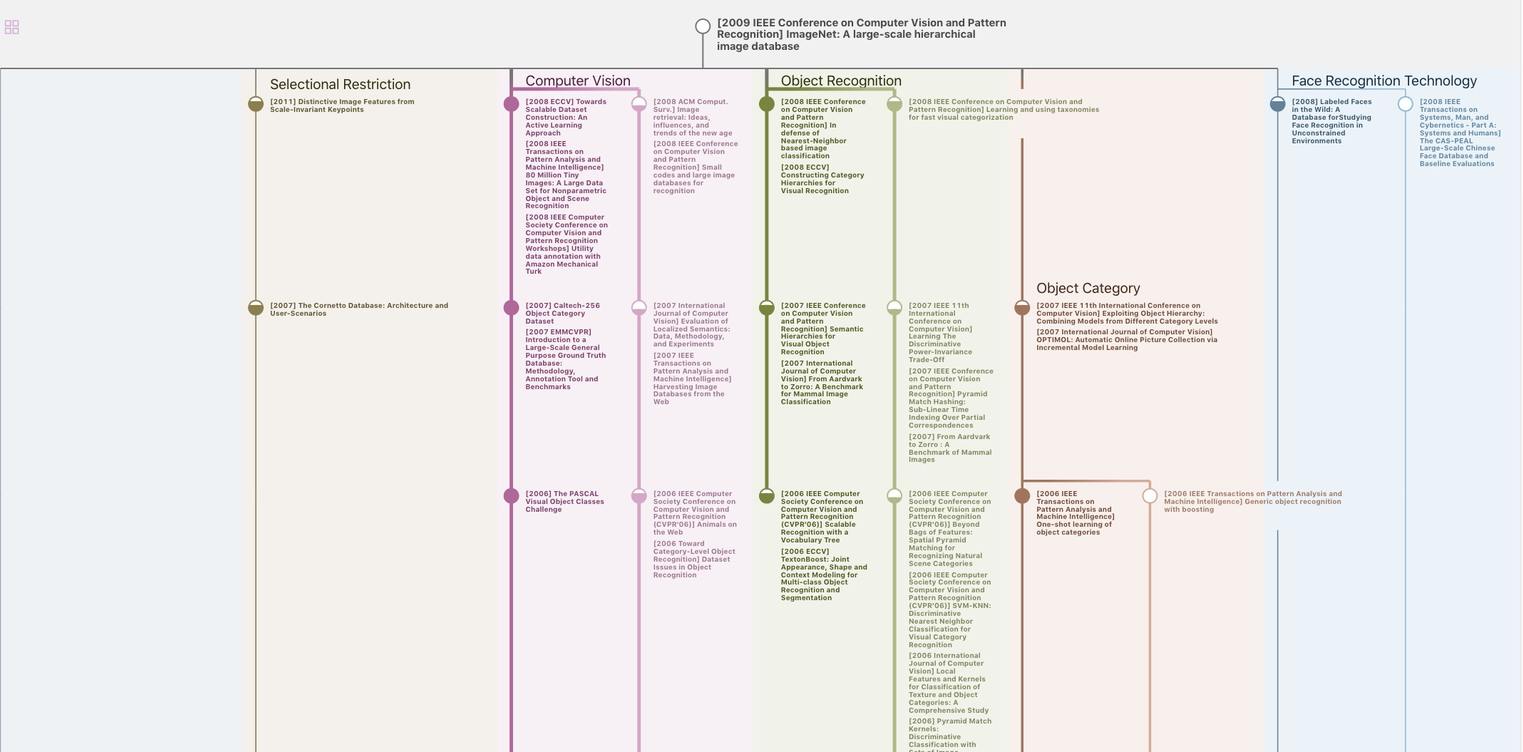
生成溯源树,研究论文发展脉络
Chat Paper
正在生成论文摘要