Federated Learning with Multi-Method Adaptive Aggregation for Enhanced Defect Detection in Power Systems
Big Data and Cognitive Computing(2024)
摘要
The detection and identification of defects in transmission lines using computer vision techniques is essential for maintaining the safety and reliability of power supply systems. However, existing training methods for transmission line defect detection models predominantly rely on single-node training, potentially limiting the enhancement of detection accuracy. To tackle this issue, this paper proposes a server-side adaptive parameter aggregation algorithm based on multi-method fusion (SAPAA-MMF) and formulates the corresponding objective function. Within the federated learning framework proposed in this paper, each client executes distributed synchronous training in alignment with the fundamental process of federated learning. The hierarchical difference between the global model, aggregated using the improved joint mean algorithm, and the global model from the previous iteration is computed and utilized as the pseudo-gradient for the adaptive aggregation algorithm. This enables the adaptive aggregation to produce a new global model with improved performance. To evaluate the potential of SAPAA-MMF, comprehensive experiments were conducted on five datasets, involving comparisons with several algorithms. The experimental results are analyzed independently for both the server and client sides. The findings indicate that SAPAA-MMF outperforms existing federated learning algorithms on both the server and client sides.
更多查看译文
关键词
federated learning,defect detection,multi-method fusion,attention mechanism
AI 理解论文
溯源树
样例
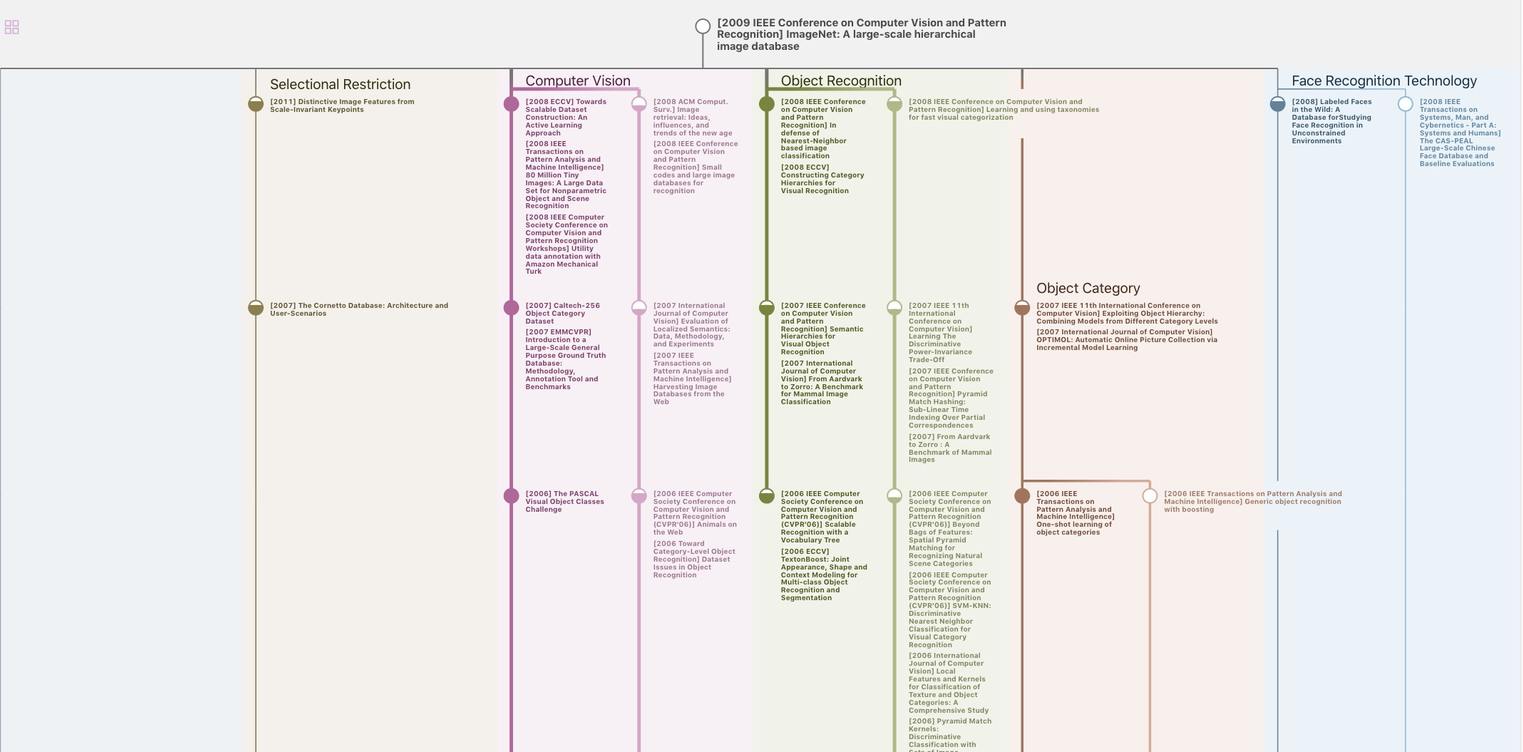
生成溯源树,研究论文发展脉络
Chat Paper
正在生成论文摘要