Xerostomia Prediction in Patients with Nasopharyngeal Carcinoma During Radiotherapy Using Segmental Dose Distribution in Dosiomics and Radiomics Models
Oral oncology(2024)
摘要
Objectives This study aimed to integrate radiomics and dosiomics features to develop a predictive model for xerostomia (XM) in nasopharyngeal carcinoma after radiotherapy. It explores the influence of distinct feature extraction methods and dose ranges on the performance. Materials and methods Data from 363 patients with nasopharyngeal carcinoma were retrospectively analyzed. We pioneered a dose-segmentation strategy, where the overall dose distribution (OD) was divided into four segmental dose distributions (SDs) at intervals of 15 Gy. Features were extracted using manual definition and deep learning, applying OD or SD and integrating radiomics and dosiomics, yielding corresponding feature scores (manually defined radiomics, MDR; manually defined dosiomics, MDD; deep learning-based radiomics, DLR; deep learning-based dosiomics, DLD). Subsequently, 18 models were developed by combining features and model types (random forest and support vector machine). Results and conclusion Under OD, O(DLR_DLD) demonstrated exceptional performance, with an optimal area under the curve (AUC) of 0.81 and an average AUC of 0.71. Within SD, S(DLR_DLD) surpassed the other models, achieving an optimal AUC of 0.90 and an average AUC of 0.85. Therefore, the integration of dosiomics into radiomics can augment predictive efficacy. The dose-segmentation strategy can facilitate the extraction of more profound information. This indicates that ScoreDLR and ScoreMDR were negatively associated with XM, whereas ScoreDLD, derived from SD exceeding 15 Gy, displayed a positive association with XM. For feature extraction, deep learning was superior to manual definition.
更多查看译文
关键词
Xerostomia,Dose-segmentation strategy,Dosiomics,Radiomics,Nasopharyngeal carcinoma
AI 理解论文
溯源树
样例
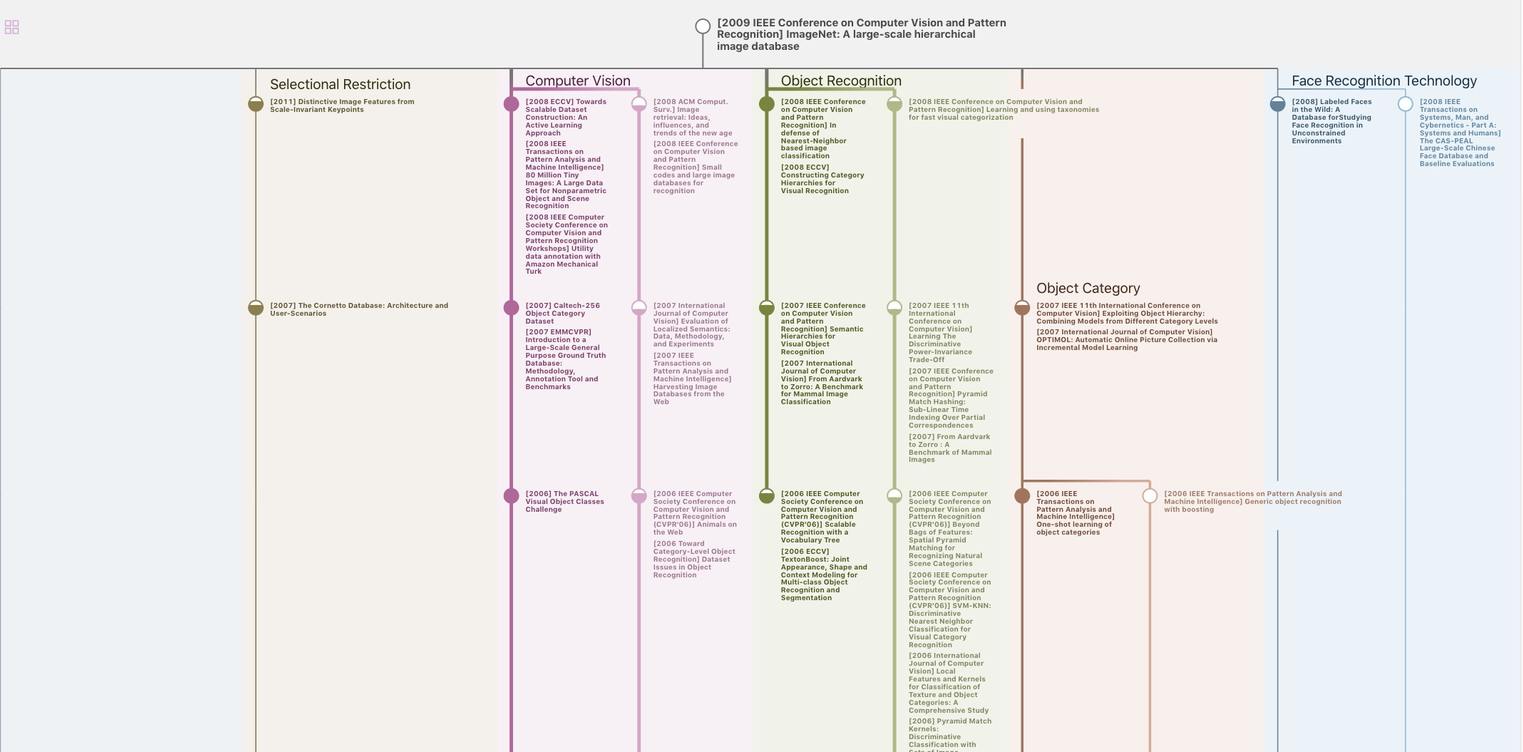
生成溯源树,研究论文发展脉络
Chat Paper
正在生成论文摘要