High Precision ECG Digitization Using Artificial Intelligence
medrxiv(2024)
摘要
Background The digitization of electrocardiograms (ECGs) is an important process in modern healthcare, enabling the preservation, transmission, and advanced analysis of ECG data. Traditional methods for digitizing ECGs from paper formats face significant challenges, particularly in real-world scenarios with varying image quality, paper distortions, and overlapping signals. Existing solutions often require manual input and are limited by their dependence on high-quality images and standardized layouts. Methods This study introduces a fully automated, deep learning-based approach for high-precision ECG digitization, implementing a two-stage process. In the ECG normalization phase, image distortions are corrected, axes are calibrated, and a standardized grid structure is generated. The ECG reconstruction phase uses deep learning techniques to extract and digitize the leads, with subsequent post-processing to refine the digital signal. The tool was evaluated using a custom-built PMcardio ECG Image Database (PM-ECG-ID) comprising 6,000 ECG images generated from 100 unique ECGs, subjected to various augmentations to simulate real-world challenges. Performance was assessed using Pearson's correlation coefficient (PCC), root mean squared error (RMSE), and signal-to-noise ratio (SNR). Results The digitization tool demonstrated an average PCC consistently exceeding 91% across all leads, SNR above 12.5 dB and an RMSE below 0.10 mV. The time to ECG digitization was consistently less than 10 seconds. The failure rate was low, averaging 6.62%, with most failures occurring under extreme conditions such as severe blurring or significant image degradation. The tool maintained robust performance even under challenging scenarios, such as low-resolution images, distorted grids, and overlapping signals. Performance metrics indicated slight variations across different leads, with leads V3-V5 showing marginally higher RMSE and lower PCC, reflecting the complexity of digitizing large amplitude signals. Conclusion Our deep learning-based approach for ECG digitization delivers high precision and reliability, effectively addressing real-world challenges such as image distortions, lighting variations, and overlapping signals. This fully automated method enhances the accessibility and utility of ECG data, supporting advanced AI-driven analysis in cardiac healthcare.
### Competing Interest Statement
V. Kresnakova, V. Boza, B. Vavrik, A. Iring, M. Hojcka, A. Rafajdus and A. Demolder are employees of Powerful Medical. T. Palus, S. Rovder, R. Herman, M. Herman, V. Jurasek, and F. Bauer are the Co-founders, and R. Hatala is a shareholder of Powerful Medical.
### Funding Statement
This study did not receive any funding.
### Author Declarations
I confirm all relevant ethical guidelines have been followed, and any necessary IRB and/or ethics committee approvals have been obtained.
Yes
The details of the IRB/oversight body that provided approval or exemption for the research described are given below:
https://physionet.org/content/ptb-xl/1.0.3/
I confirm that all necessary patient/participant consent has been obtained and the appropriate institutional forms have been archived, and that any patient/participant/sample identifiers included were not known to anyone (e.g., hospital staff, patients or participants themselves) outside the research group so cannot be used to identify individuals.
Yes
I understand that all clinical trials and any other prospective interventional studies must be registered with an ICMJE-approved registry, such as ClinicalTrials.gov. I confirm that any such study reported in the manuscript has been registered and the trial registration ID is provided (note: if posting a prospective study registered retrospectively, please provide a statement in the trial ID field explaining why the study was not registered in advance).
Yes
I have followed all appropriate research reporting guidelines, such as any relevant EQUATOR Network research reporting checklist(s) and other pertinent material, if applicable.
Yes
All data produced are available online at: https://doi.org/10.5281/zenodo.13617672
更多查看译文
AI 理解论文
溯源树
样例
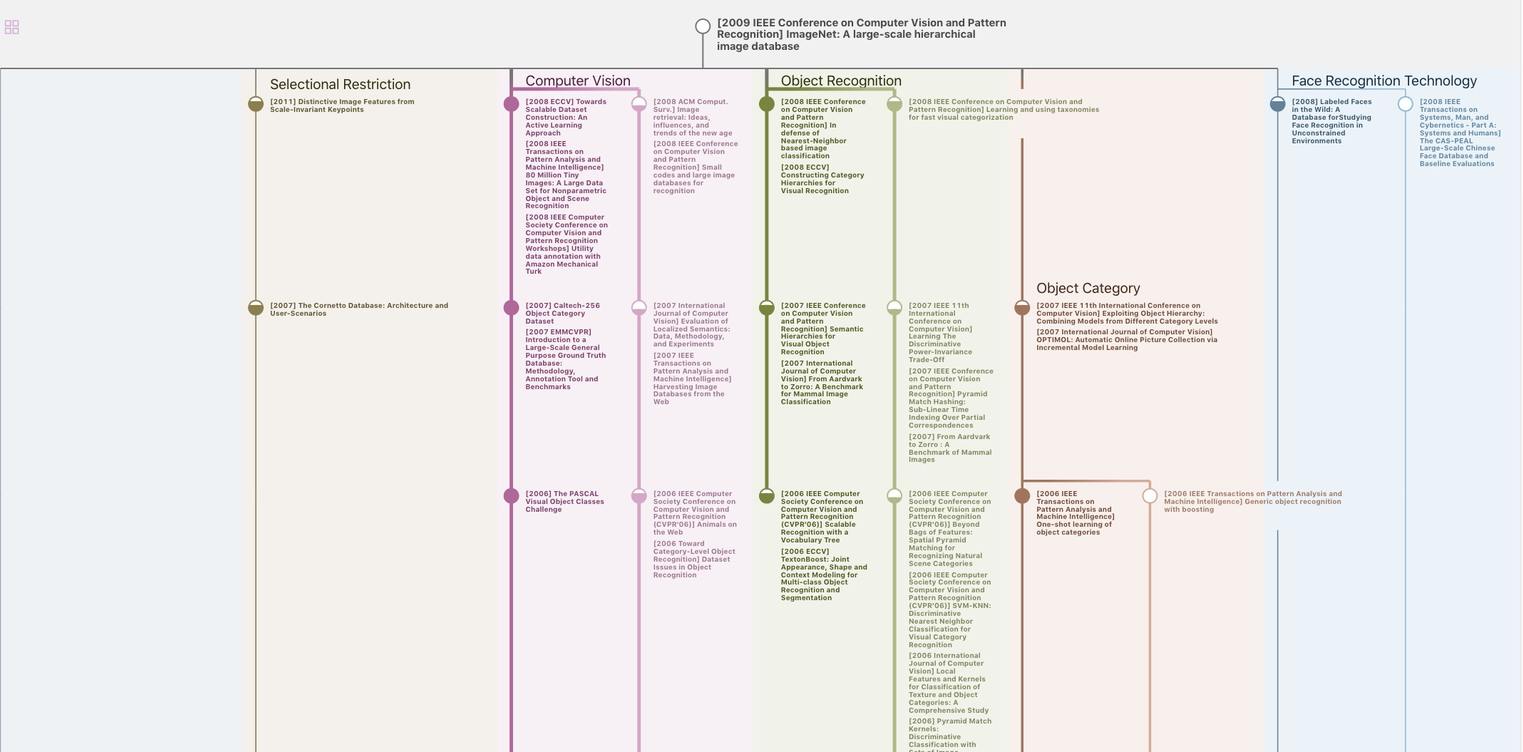
生成溯源树,研究论文发展脉络
Chat Paper
正在生成论文摘要