Performance of Machine Learning-Based Models to Screen Obstructive Sleep Apnea in Pregnancy
npj Women's Health(2024)
摘要
The purpose of this study is to improve the performance of existing OSA screening tools for pregnant women with machine learning algorithms. A total of 296 pregnant women who complained of snoring OSA were recruited to complete four traditional OSA screening questionnaires: Berlin, STOP, STOP-Bang questionnaires, and Epworth Sleepiness Scale. OSA status was confirmed using an overnight type III home sleep test. 76 of the participants repeated the procedure at different trimesters, generating a total of 402 records. The participants were randomly split into a training set (n = 207) and a test set (n = 89) in a 7:3 ratio. We applied a logistic regression model to build Mixture of Models for OSA screen (MoMOSA) based on demographic data and selected questions from all the questionnaires. Finally, we transformed the MoMOSA into a new questionnaire with a nomogram. MoMOSA, with 13 features, achieved the highest performance among the traditional questionnaires and built models.
更多查看译文
AI 理解论文
溯源树
样例
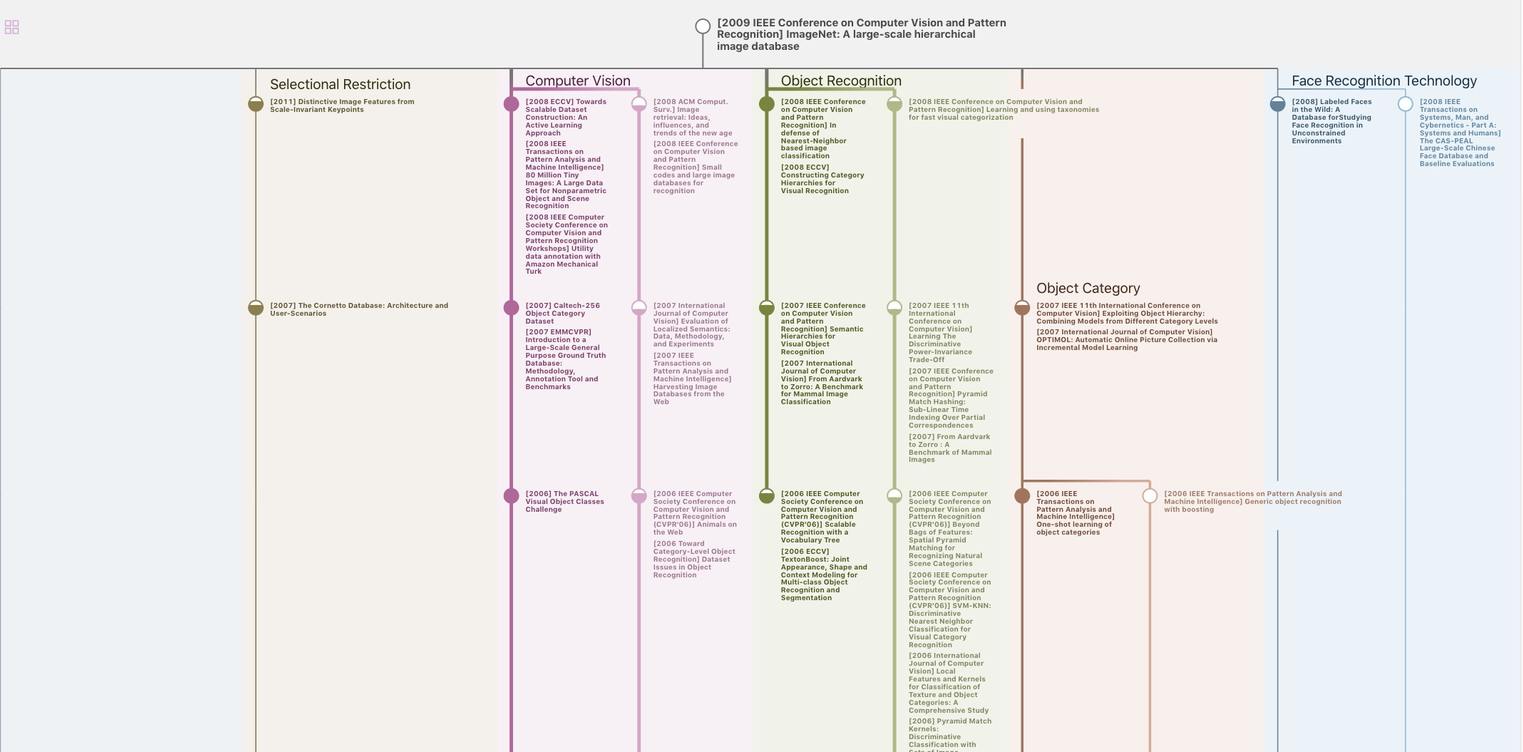
生成溯源树,研究论文发展脉络
Chat Paper
正在生成论文摘要