Deep Learning Helps Discriminating Autoimmune Hepatitis and Primary Biliary Cholangitis
JHEP Reports(2024)
摘要
Background and Aims Biliary abnormalities in Autoimmune Hepatitis (AIH) and interface hepatitis in Primary Biliary Cholangitis (PBC) occur frequently, and misinterpretation may lead to therapeutic mistakes with a negative impact on patients. This study investigates the use of a deep learning (DL)-based pipeline for the diagnosis of AIH and PBC to aid differential diagnosis. Method We conducted a multicenter study across six European referral centers, and built a library of digitized liver biopsy slides dating from 1997 to 2023. A training set of 354 cases (266 AIH and 102 PBC) and an external validation set of 92 cases (62 AIH and 30 PBC) were available for analysis. A novel DL model, the Autoimmune Liver Neural Estimator (ALNE), was trained on whole-slide images (WSIs) with haematoxylin and eosin staining, without human annotations. The ALNE model was evaluated against clinico-pathological diagnoses and tested for interobserver variability among general pathologists. Results The ALNE model demonstrated high accuracy in differentiating AIH from PBC, achieving an AUROC of 0.81 in external validation. Attention heatmaps showed that ALNE tends to focus more on areas with increased inflammation, associating such patterns predominantly with AIH. A multivariate explainable ML model revealed that PBC cases misclassified as AIH more often had ALP values between 1 x Upper Limit of Normal (ULN) and 2 x ULN, coupled with AST values above 1 x ULN . Inconsistency among general pathologists was noticed when evaluating a random sample of the same cases (Fleiss's kappa value 0.09). Conclusion The ALNE model is the first system generating a quantitative and accurate differential diagnosis among AIH and PBC cases.
更多查看译文
关键词
computational pathology,digital pathology,liver,rare liver diseases,autoimmunity,artificial intelligence
AI 理解论文
溯源树
样例
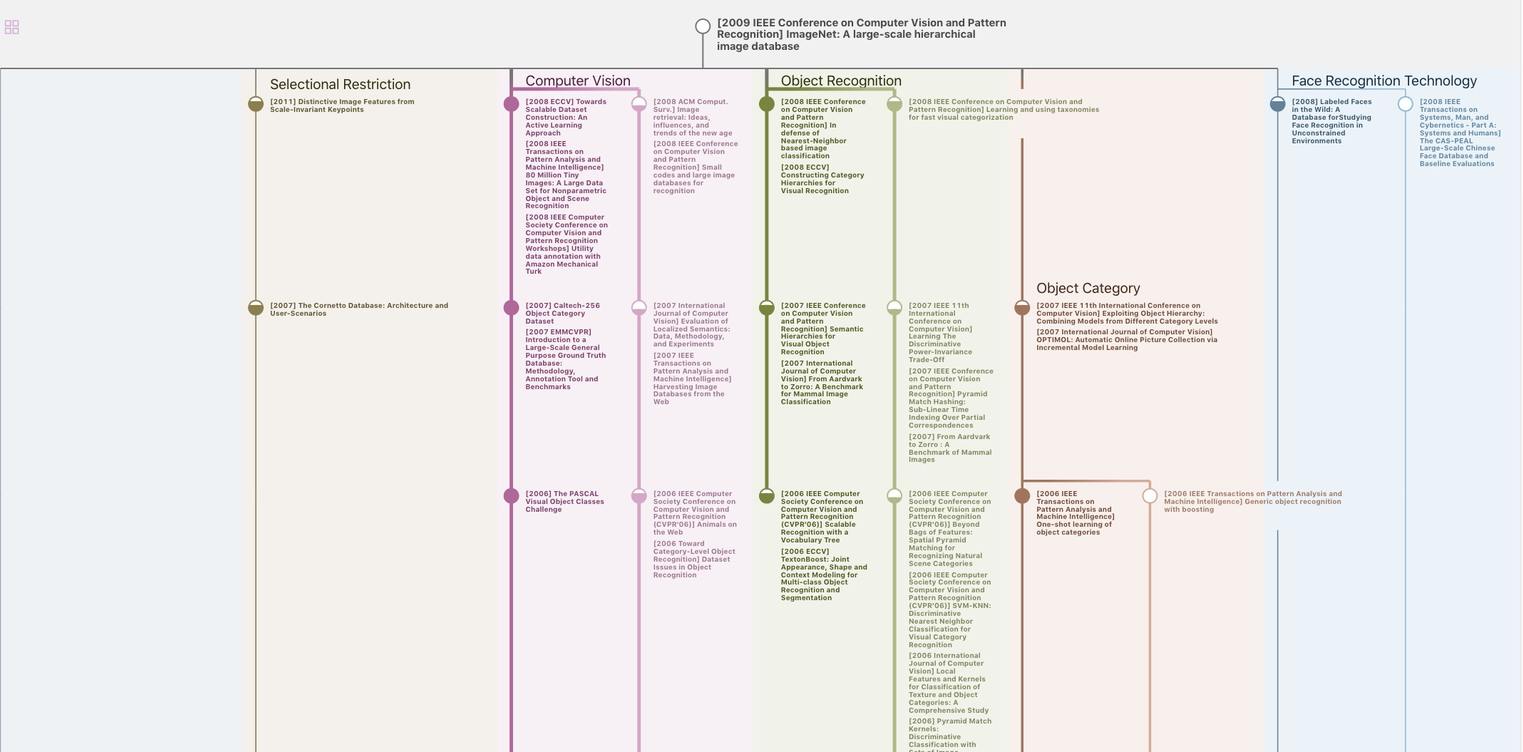
生成溯源树,研究论文发展脉络
Chat Paper
正在生成论文摘要