Exploiting XAI Maps to Improve MS Lesion Segmentation and Detection in MRI
biorxiv(2024)
摘要
To date, several methods have been developed to explain deep learning algorithms for classification tasks. Recently, an adaptation of two of such methods has been proposed to generate instance-level explainable maps in a semantic segmentation scenario, such as multiple sclerosis (MS) lesion segmentation. In the mentioned work, a 3D U-Net was trained and tested for MS lesion segmentation, yielding an F1 score of 0.7006, and a positive predictive value (PPV) of 0.6265. The distribution of values in explainable maps exposed some differences between maps of true and false positive (TP/FP) examples. Inspired by those results, we explore in this paper the use of characteristics of lesion-specific saliency maps to refine segmentation and detection scores. We generate around 21000 maps from as many TP/FP lesions in a batch of 72 patients (training set) and 4868 from the 37 patients in the test set. 93 radiomic features extracted from the first set of maps were used to train a logistic regression model and classify TP versus FP. On the test set, F1 score and PPV were improved by a large margin when compared to the initial model, reaching 0.7450 and 0.7817, with 95 and [0.7679, 0.7962], respectively. These results suggest that saliency maps can be used to refine prediction scores, boosting a model's performances.
更多查看译文
AI 理解论文
溯源树
样例
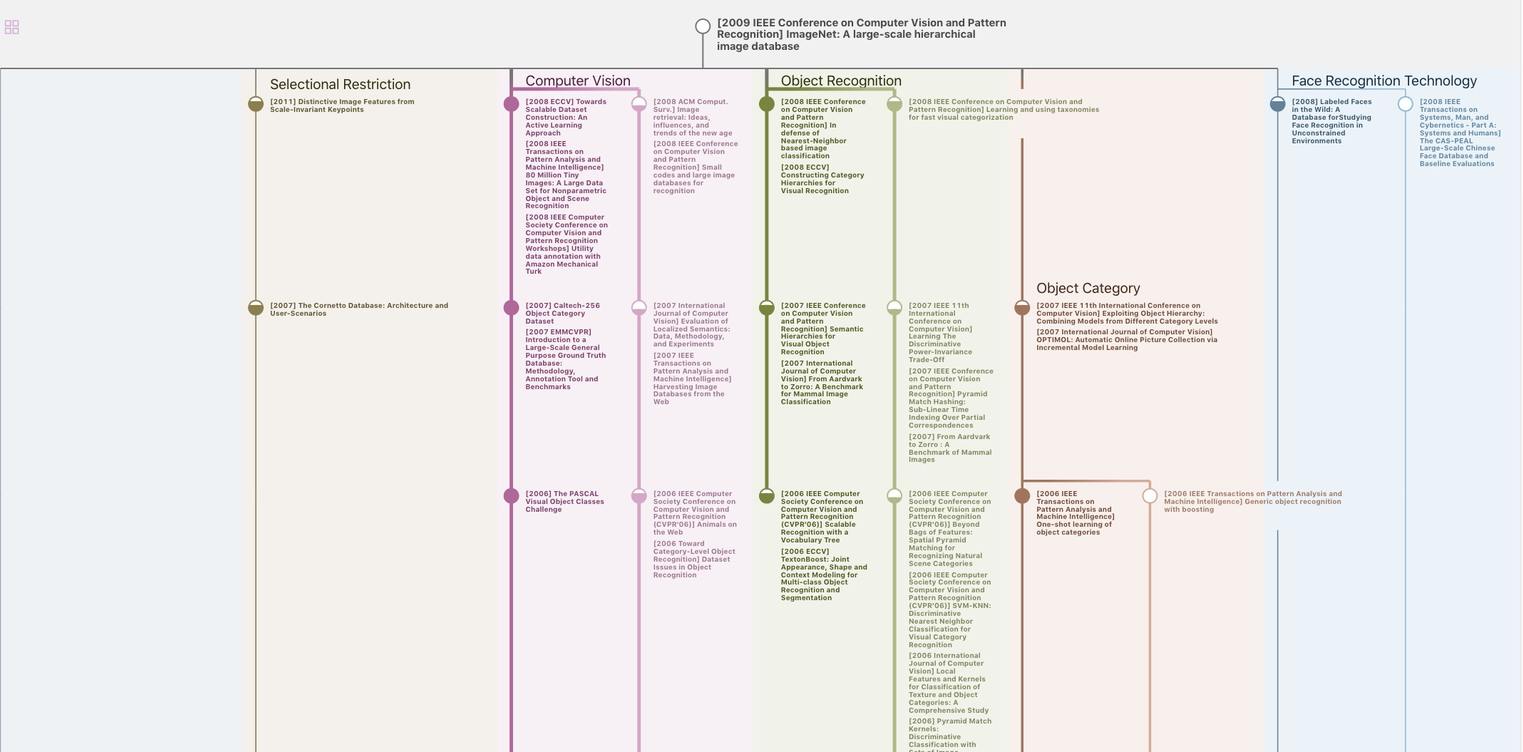
生成溯源树,研究论文发展脉络
Chat Paper
正在生成论文摘要