Graph Deep Active Learning Framework for Data Deduplication
Big Data Mining and Analytics(2024)
摘要
With the advent of the era of big data, an increasing amount of duplicate data are expressed in different forms. In order to reduce redundant data storage and improve data quality, data deduplication technology has never become more significant than nowadays. It is usually necessary to connect multiple data tables and identify different records pointing to the same entity, especially in the case of multi-source data deduplication. Active learning trains the model by selecting the data items with the maximum information divergence and reduces the data to be annotated, which has unique advantages in dealing with big data annotations. However, most of the current active learning methods only employ classical entity matching and are rarely applied to data deduplication tasks. To fill this research gap, we propose a novel graph deep active learning framework for data deduplication, which is based on similarity algorithms combined with the bidirectional encoder representations from transformers (BERT) model to extract the deep similarity features of multi-source data records, and first introduce the graph active learning strategy to build a clean graph to filter the data that needs to be labeled, which is used to delete the duplicate data that retain the most information. Experimental results on real-world datasets demonstrate that the proposed method outperforms state-of-the-art active learning models on data deduplication tasks.
更多查看译文
关键词
data deduplication,active learning,similarity calculation
AI 理解论文
溯源树
样例
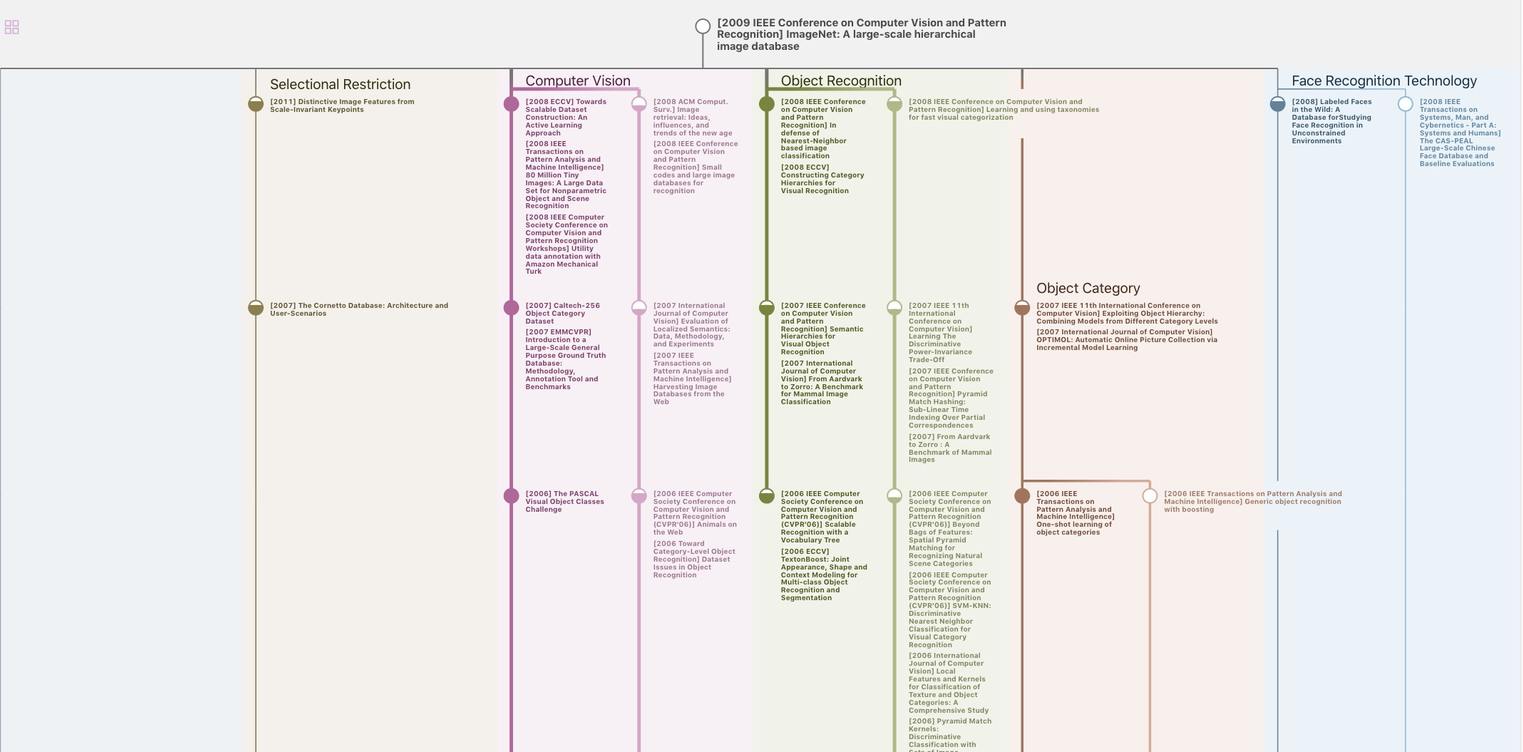
生成溯源树,研究论文发展脉络
Chat Paper
正在生成论文摘要