An Indicator-Based Multi-Objective Variable Neighborhood Search Approach for Query-Focused Summarization
Swarm and Evolutionary Computation(2024)
摘要
Currently, automatic multi-document summarization is an interesting subject in numerous fields of study. As a part of it, query-focused summarization is becoming increasingly important in recent times. These methods can automatically produce a summary based on a query given by the user, including the most relevant information from the query at the same time as the redundancy among sentences is reduced. This can be achieved by developing and applying a multi-objective optimization approach. In this paper, an Indicator-based Multi-Objective Variable Neighborhood Search (IMOVNS) algorithm has been designed, implemented, and tested for the query-focused extractive multi-document summarization problem. Experiments have been carried out with datasets from Text Analysis Conference (TAC). The results were evaluated using the Recall-Oriented Understudy for Gisting Evaluation (ROUGE) metrics. IMOVNS has greatly improved the results presented in the scientific literature, providing improvement percentages in ROUGE metric reaching up to 69.24% in ROUGE-1, up to 57.70% in ROUGE-2, and up to 77.37% in ROUGE-SU4 scores. Hence, the proposed IMOVNS offers a promising solution to the query-focused summarization problem, thus highlighting its efficacy and potential for enhancing automatic summarization techniques.
更多查看译文
关键词
Evolutionary computation,Variable neighborhood search,Multi-objective optimization,Indicator-based optimization,Query-focused summarization
AI 理解论文
溯源树
样例
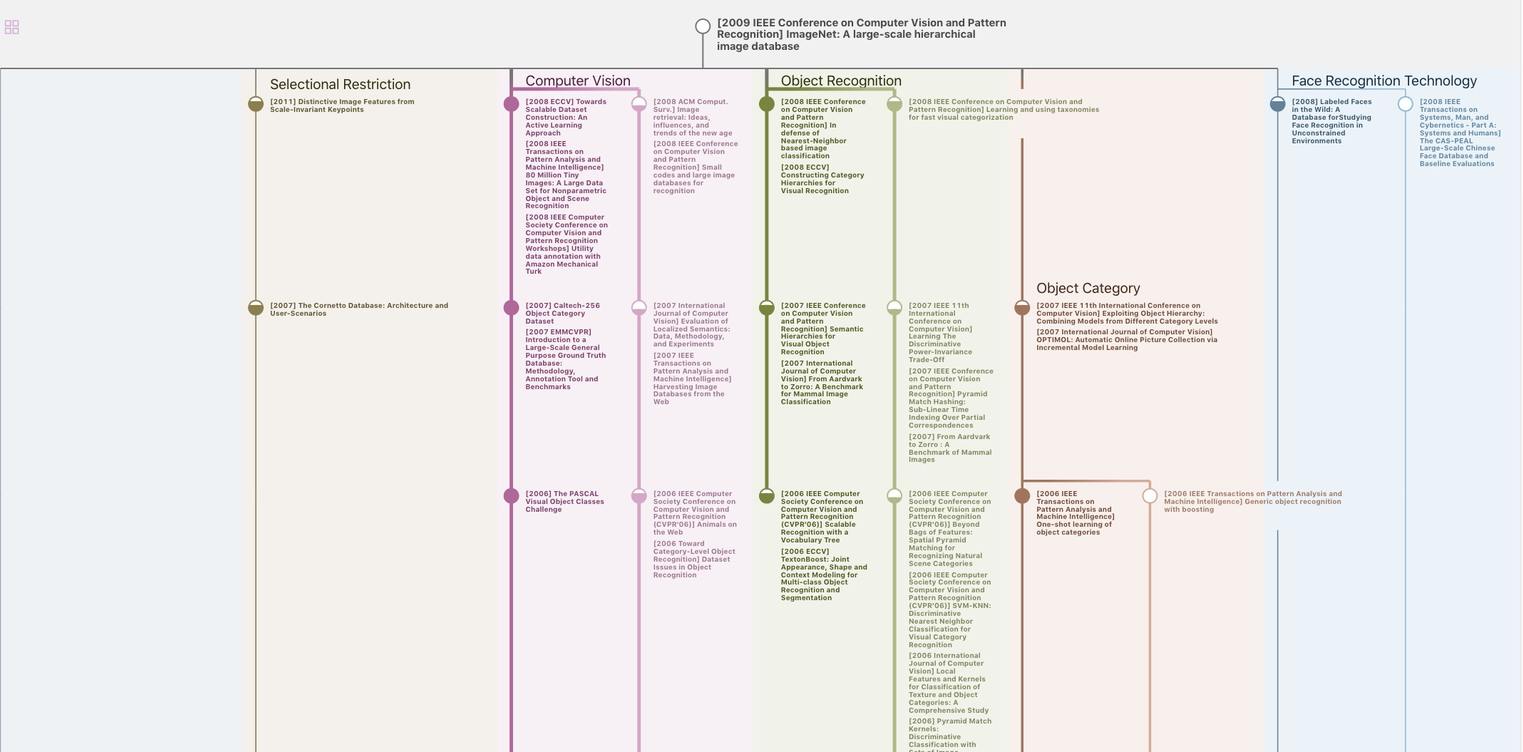
生成溯源树,研究论文发展脉络
Chat Paper
正在生成论文摘要