Brain Tumor Segmentation Using Generative Adversarial Networks
IEEE Access(2024)
摘要
Deep learning has played a vital role in advancing medical research, particularly in brain tumor segmentation. Despite using numerous deep learning algorithms for this purpose, accurately and reliably segmenting brain tumors remains a significant challenge. Segmentation of precise tumors is essential for the effective treatment of brain diseases. While deep learning offers a range of algorithms for segmentation, they still face limitations when analyzing medical images due to the variations in tumor shape, size, and location. This study proposes a deep learning approach combining a Generative Adversarial Network (GAN) with transfer learning and auto-encoder techniques to enhance brain tumor segmentation. The GAN incorporates a generator and discriminator to generate superior segmentation outcomes. In the generator, we applied downsampling and upsampling for tumor segmentation. In addition, an autoencoder is applied in which the encoder retains as much information as possible and then the decoder with those encodings reconstructs the image. At the bottleneck, the transfer learning technique is applied using the DenseNet model. By combining autoencoder techniques with transfer learning methodologies in GANs feature learning is enhanced, training time is reduced, and stability is increased. In this work, we enhanced the accuracy of brain tumor segmentation and even achieved better results for tumors having small size. We train and evaluate our proposed model using the publicly available BraTS 2021 dataset. The experimental result shows a dice score of 0.94 for the whole tumor, 0.86 for the tumor core, and 0.82 for the enhancing tumor. It is also shown that we achieve 2% to 4% higher accuracy as compared to other methods.
更多查看译文
关键词
Deep learning,GAN,auto-encoder,up sampling,down sampling,transfer learning,BraTS,DenseNet
AI 理解论文
溯源树
样例
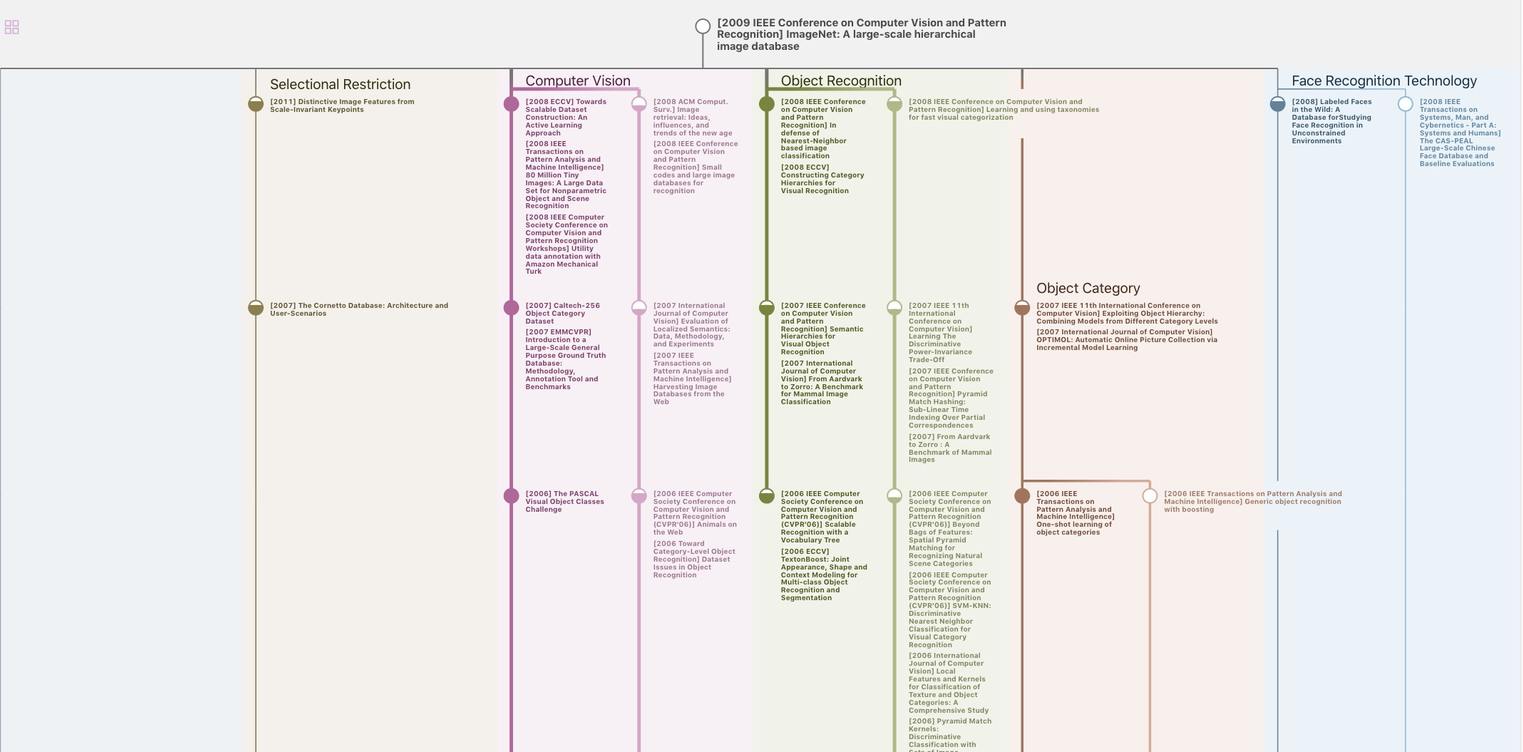
生成溯源树,研究论文发展脉络
Chat Paper
正在生成论文摘要