Bayesian Prototype Learning for Few-Shot Radar Signal Intra-Pulse Modulation Recognition
IEEE Communications Letters(2024)
Abstract
In an open electromagnetic environment, few-shot learning (FSL) has been widely used to recognize new classes of radar signals with few labeled data, which is needed in spectrum management or electronic reconnaissance systems for saving time and human resources. However, suffering from the distribution shift, existing methods minimizing the empirical risk on few labeled data may lead to model degradation on unseen data. We propose a Bayesian prototype learning (BPL) method for few-shot radar signal modulation recognition. Specifically, we design a Bayesian prototype (BP) learner that enhances generalization to unseen data, regularizing the prototype embedding space by modeling prototype learning as a variational inference problem. Furthermore, to transfer base class knowledge efficiently, we design a shallow-deep feature map fusion (SDF) block, combining feature maps from shallow and deep layers. Additionally, a class-covariance metric (CCM) is introduced to refine classification boundaries by considering intra-class distributions. Extensive experiments show the superiority of our method, achieving a recognition accuracy of 97.91% with 5 labeled data per class.
MoreTranslated text
Key words
Few-shot learning,radar signal intra-pulse modulation recognition,variational inference,feature fusion
AI Read Science
Must-Reading Tree
Example
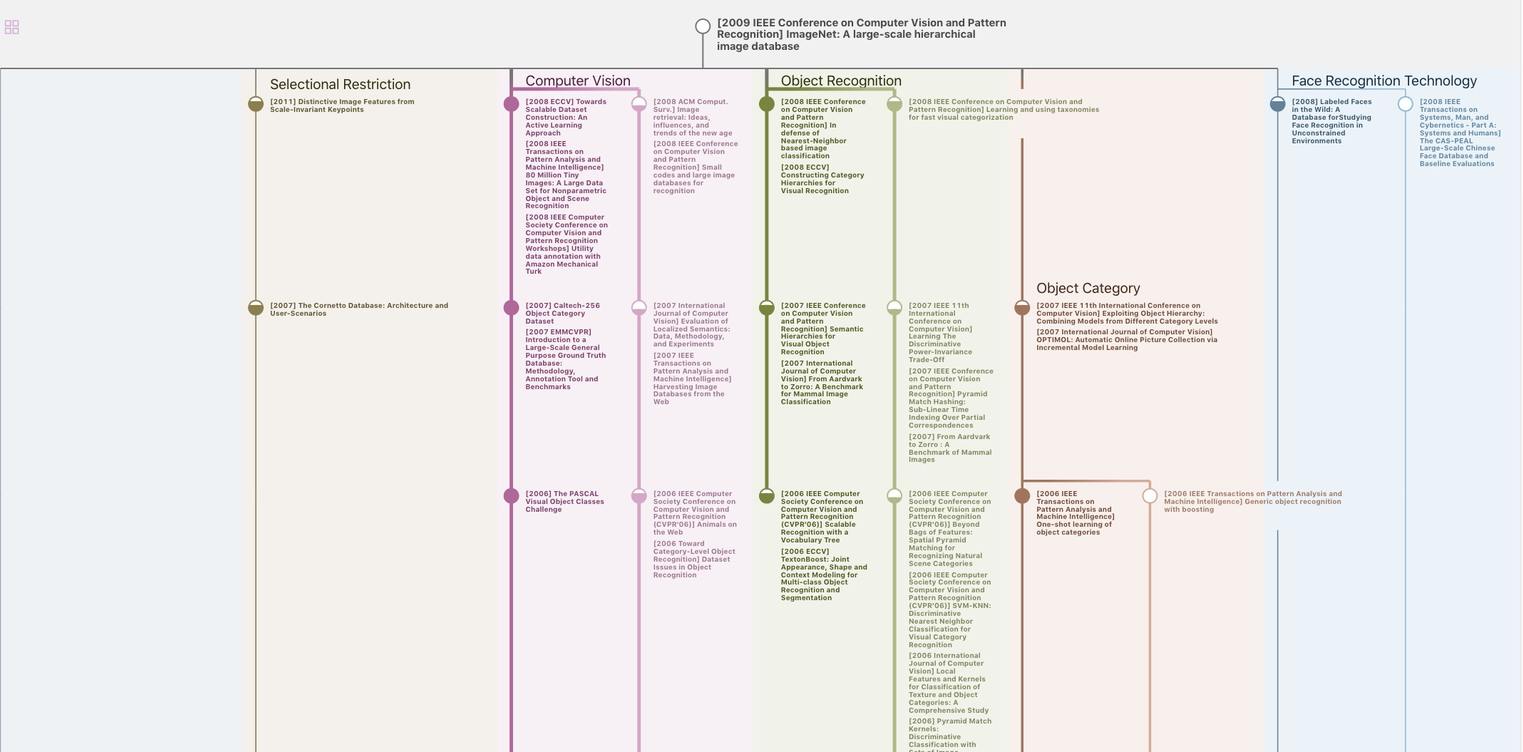
Generate MRT to find the research sequence of this paper
Chat Paper
Summary is being generated by the instructions you defined