Enhancing Soil Particle Content Prediction Accuracy: Advanced Hyperspectral Analysis and Machine Learning Models
Journal of Soils and Sediments(2024)
摘要
Prediction of soil particle content is essential for soil texture classification, soil management and agricultural production. This study aimed to achieve high-accuracy predictions of soil particle content in the Ogan-Kucha River Oasis using hyperspectral data and environmental variables. We collected 62 representative surface soil samples (depth: 0–10 cm), and conducting indoor soil particle content and spectral measurements. The relationship between environmental variables and soil particle content was analyzed using the Boruta algorithm, and seven three-band spectral indices (TBIs) were constructed using an optimal band algorithm. By integrating environmental covariates and TBIs, soil particle inversion models were developed using the extreme learning machine (ELM), backpropagation neural networks (BP), neural networks optimized with the sparrow search algorithm (SSA-BP), and neural networks optimized with the sparrow search algorithm enhanced by Sine chaos mapping (Sine-SSA-BP). The results demonstrated that (1) the Boruta algorithm identified key environmental covariates that affect specific soil particle components; (2) there was significant variation in the correlation between different TBIs and soil particle content, with absolute correlation coefficients ranging from 0.225 to 0.852; (3) the estimation models established by the four machine learning algorithms performed well in predicting soil particle content, particularly for silt (R2: 0.664–0.858, RMSE: 11.107–17.128) and clay (R2: 0.444–0.857, RMSE: 0.550–1.405), for which higher accuracy was achieved; and (4) compared with the traditional ELM (R2: 0.422–0.664), BP (R2: 0.487–0.673) and SSA-BP models (R2: 0.625–0.777), the Sine-SSA-BP model showed a significant improvement in prediction accuracy, with the highest R2 reaching 0.858. Compared to the traditional ELM, BP and SSA-BP models, the Sine-SSA-BP model significantly excelled in predicting soil particle content, offering innovative insights and robust support for soil texture classification and management.
更多查看译文
关键词
Soil particle content,Hyperspectral,Backpropagation neural network,Chaos mapping
AI 理解论文
溯源树
样例
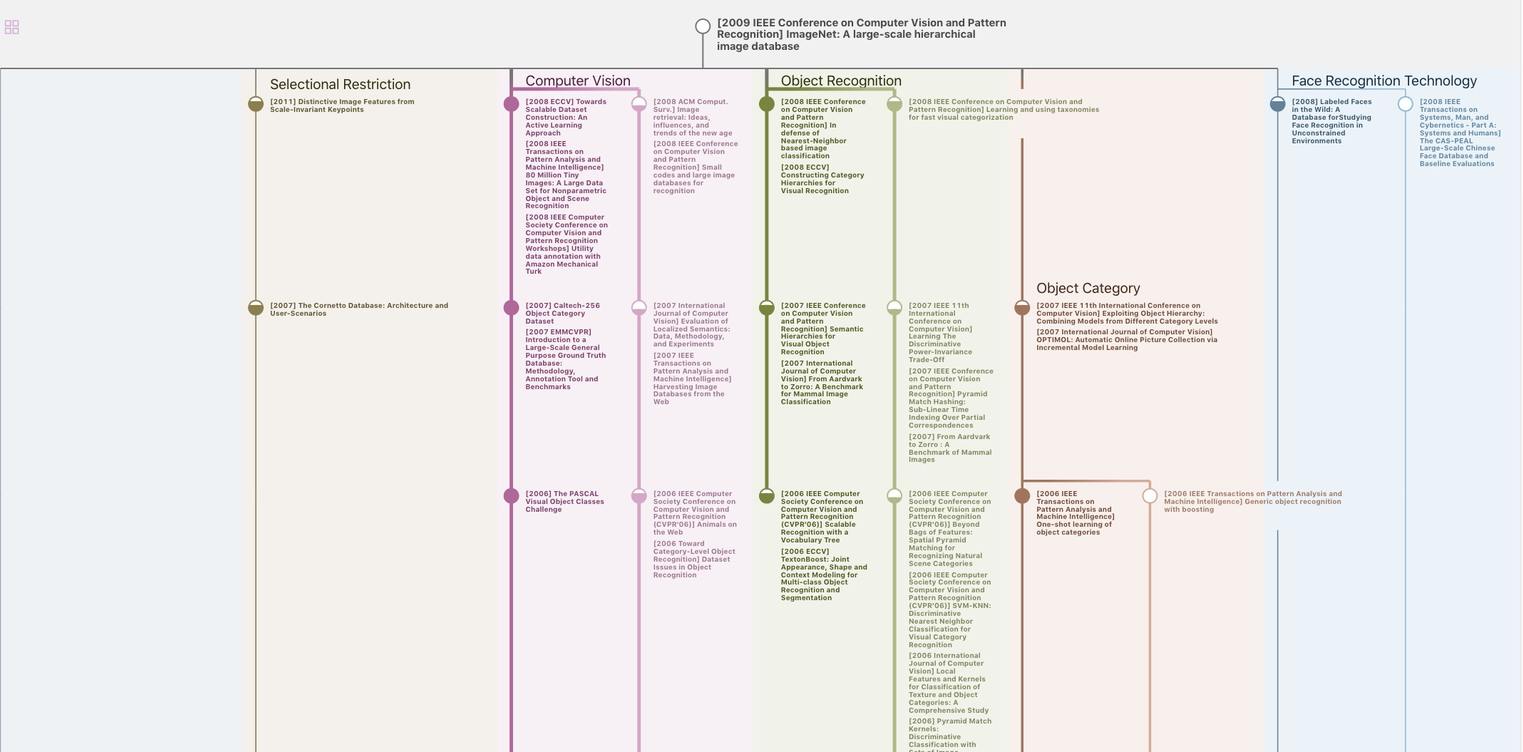
生成溯源树,研究论文发展脉络
Chat Paper
正在生成论文摘要