Short-Term Wind Power Prediction Based on Encoder–Decoder Network and Multi-Point Focused Linear Attention Mechanism
Sensors (Basel, Switzerland)(2024)
摘要
Wind energy is a clean energy source that is characterised by significant uncertainty. The electricity generated from wind power also exhibits strong unpredictability, which when integrated can have a substantial impact on the security of the power grid. In the context of integrating wind power into the grid, accurate prediction of wind power generation is crucial in order to minimise damage to the grid system. This paper proposes a novel composite model (MLL-MPFLA) that combines a multilayer perceptron (MLP) and an LSTM-based encoder–decoder network for short-term prediction of wind power generation. In this model, the MLP first extracts multidimensional features from wind power data. Subsequently, an LSTM-based encoder-decoder network explores the temporal characteristics of the data in depth, combining multidimensional features and temporal features for effective prediction. During decoding, an improved focused linear attention mechanism called multi-point focused linear attention is employed. This mechanism enhances prediction accuracy by weighting predictions from different subspaces. A comparative analysis against the MLP, LSTM, LSTM–Attention–LSTM, LSTM–Self_Attention–LSTM, and CNN–LSTM–Attention models demonstrates that the proposed MLL-MPFLA model outperforms the others in terms of MAE, RMSE, MAPE, and R2, thereby validating its predictive performance.
更多查看译文
关键词
short-term wind power prediction,encoder–decoder network,LSTM network,multi-point focused linear attention
AI 理解论文
溯源树
样例
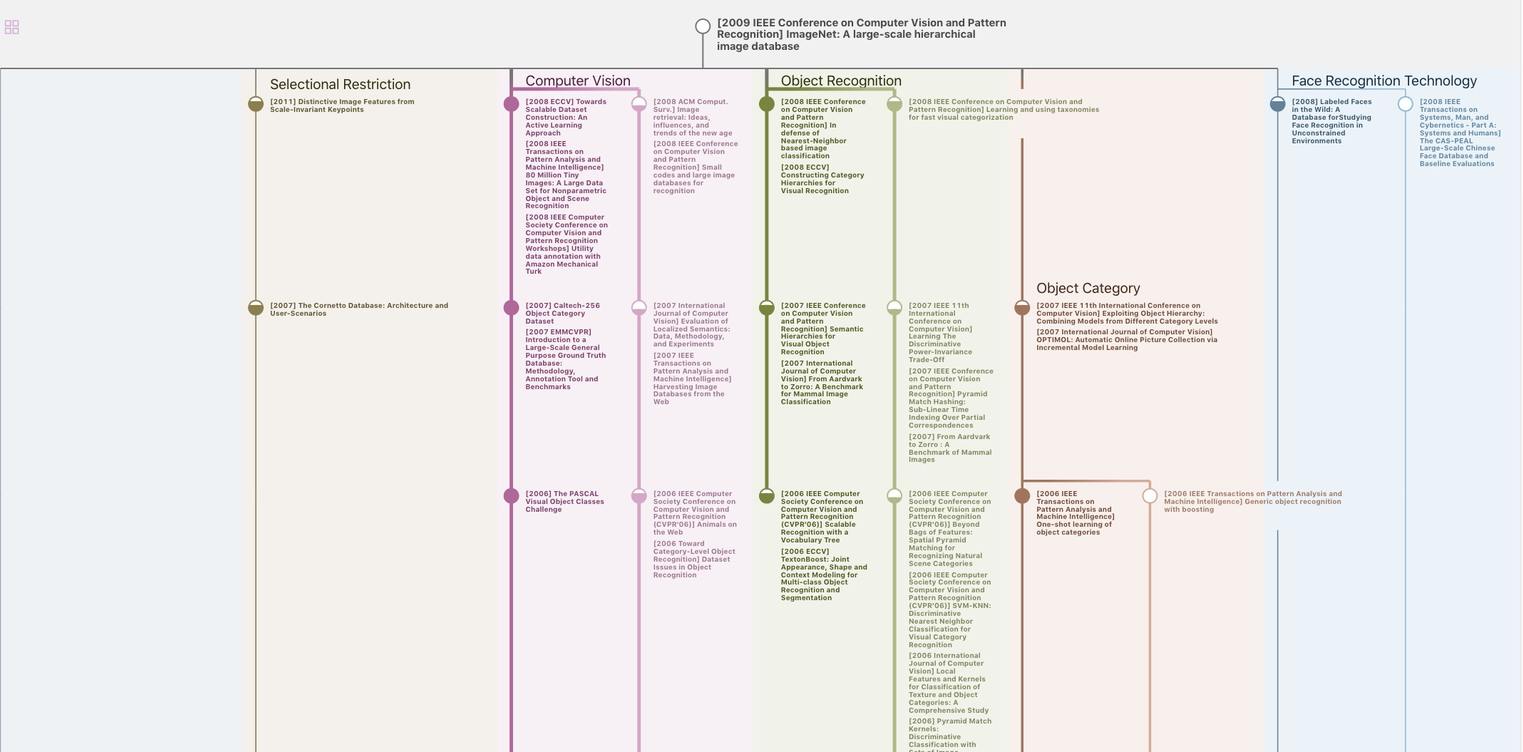
生成溯源树,研究论文发展脉络
Chat Paper
正在生成论文摘要