Customizing Graph Neural Network for CAD Assembly Recommendation
KDD 2024(2024)
关键词
CAD Assembly Recommendation,Graph Neural Network,Neural Architecture Search,Customized Recommender System
AI 理解论文
溯源树
样例
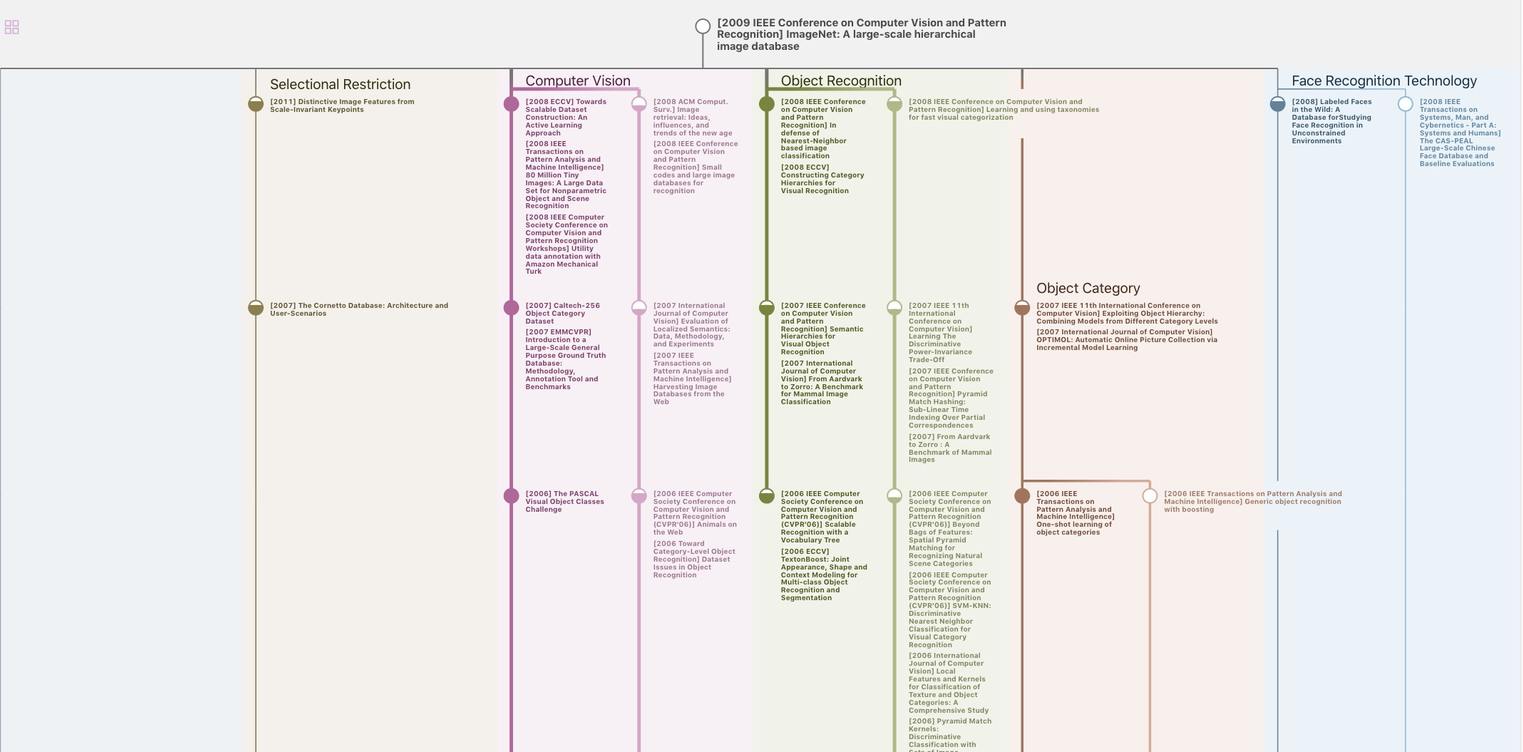
生成溯源树,研究论文发展脉络
Chat Paper
正在生成论文摘要
KDD 2024(2024)