Class-balanced Open-set Semi-supervised Object Detection for Medical Images
arxiv(2024)
Abstract
Medical image datasets in the real world are often unlabeled and imbalanced, and Semi-Supervised Object Detection (SSOD) can utilize unlabeled data to improve an object detector. However, existing approaches predominantly assumed that the unlabeled data and test data do not contain out-of-distribution (OOD) classes. The few open-set semi-supervised object detection methods have two weaknesses: first, the class imbalance is not considered; second, the OOD instances are distinguished and simply discarded during pseudo-labeling. In this paper, we consider the open-set semi-supervised object detection problem which leverages unlabeled data that contain OOD classes to improve object detection for medical images. Our study incorporates two key innovations: Category Control Embed (CCE) and out-of-distribution Detection Fusion Classifier (OODFC). CCE is designed to tackle dataset imbalance by constructing a Foreground information Library, while OODFC tackles open-set challenges by integrating the “unknown” information into basic pseudo-labels. Our method outperforms the state-of-the-art SSOD performance, achieving a 4.25 mAP improvement on the public Parasite dataset.
MoreTranslated text
AI Read Science
Must-Reading Tree
Example
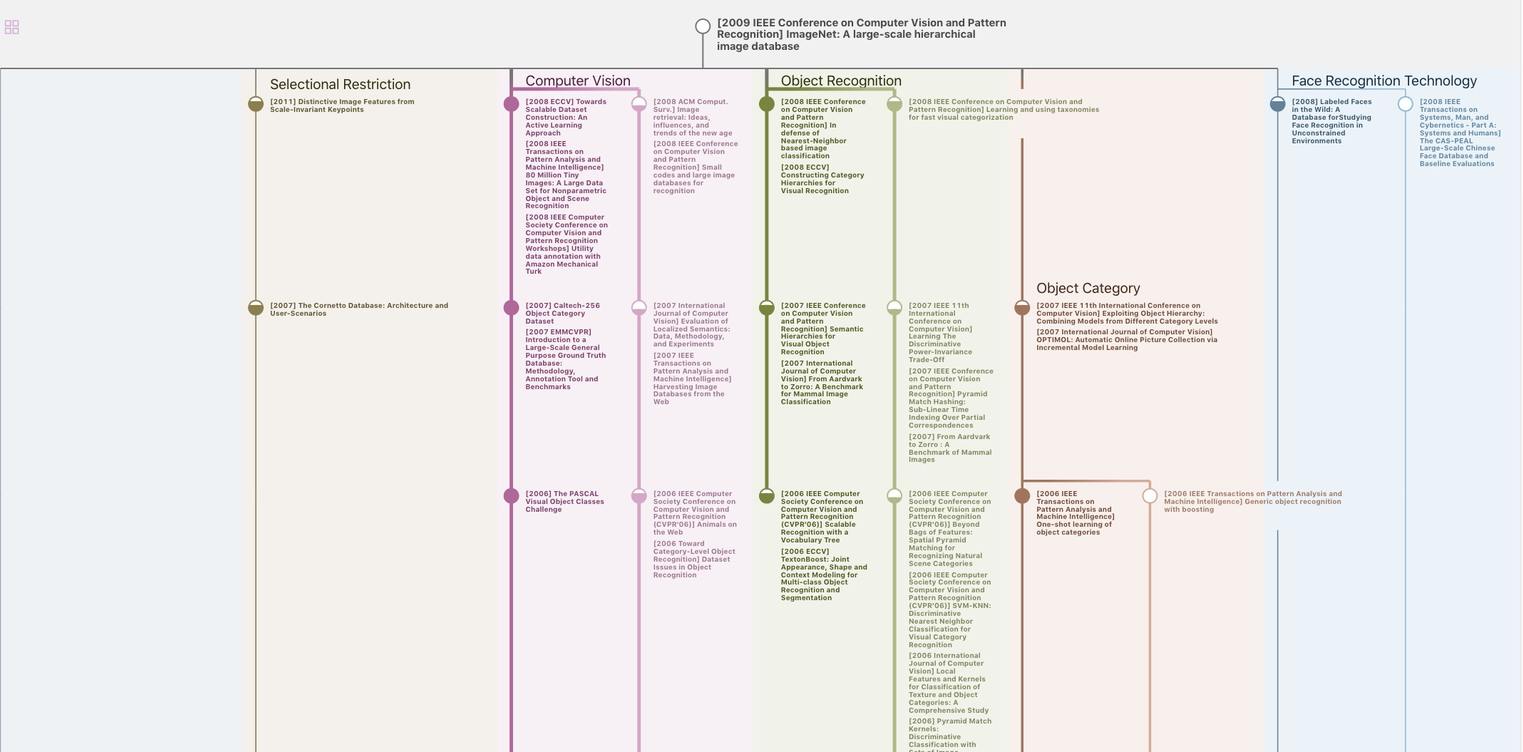
Generate MRT to find the research sequence of this paper
Chat Paper
Summary is being generated by the instructions you defined