MSI-UNet: A Flexible UNet-Based Multi-Scale Interactive Framework for 3D Gastric Tumor Segmentation on CT Scans
2024 IEEE International Symposium on Biomedical Imaging (ISBI)(2024)
摘要
Accurate segmentation of gastric tumors is critical yet presents a formidable challenge in medical imaging, where conventional UNet-based frameworks, despite their prevalence, falter on intricate tumor samples due to their limited interactive capacities. The SAM-based segmentation methods address this shortcoming yet with insufficient accuracy. By ingeniously blending images with mask inputs, our MSI-UNet leverages a U-shaped design to deliver pixel-perfect segmentation accuracy, while a novel multi-scale attention module adeptly harnesses interaction points for refined information extraction. When benchmarked on gastric tumor segmentation tasks, MSI-UNet surpasses existing state-of-the-art methods, elevating the Dice Similarity Coefficient (DSC) from 74.82% to 79.3% and minimizing Average Surface Distance (ASD) from 6.46 to 1.98, achieving a comparable accuracy with inter-radiologist consistency of 79.7% DSC. Furthermore, our framework demonstrates superior predictive prowess in survival analysis, enhancing the C-index from 61.7% to 68.68%. Ample experimental comparisons have substantiated that MSI-UNet holds the potential to offer considerable assistance to healthcare professionals in managing and decoding subsequent medical procedures.
更多查看译文
关键词
Gastric tumor segmentation,interactive segmentation,deep learning
AI 理解论文
溯源树
样例
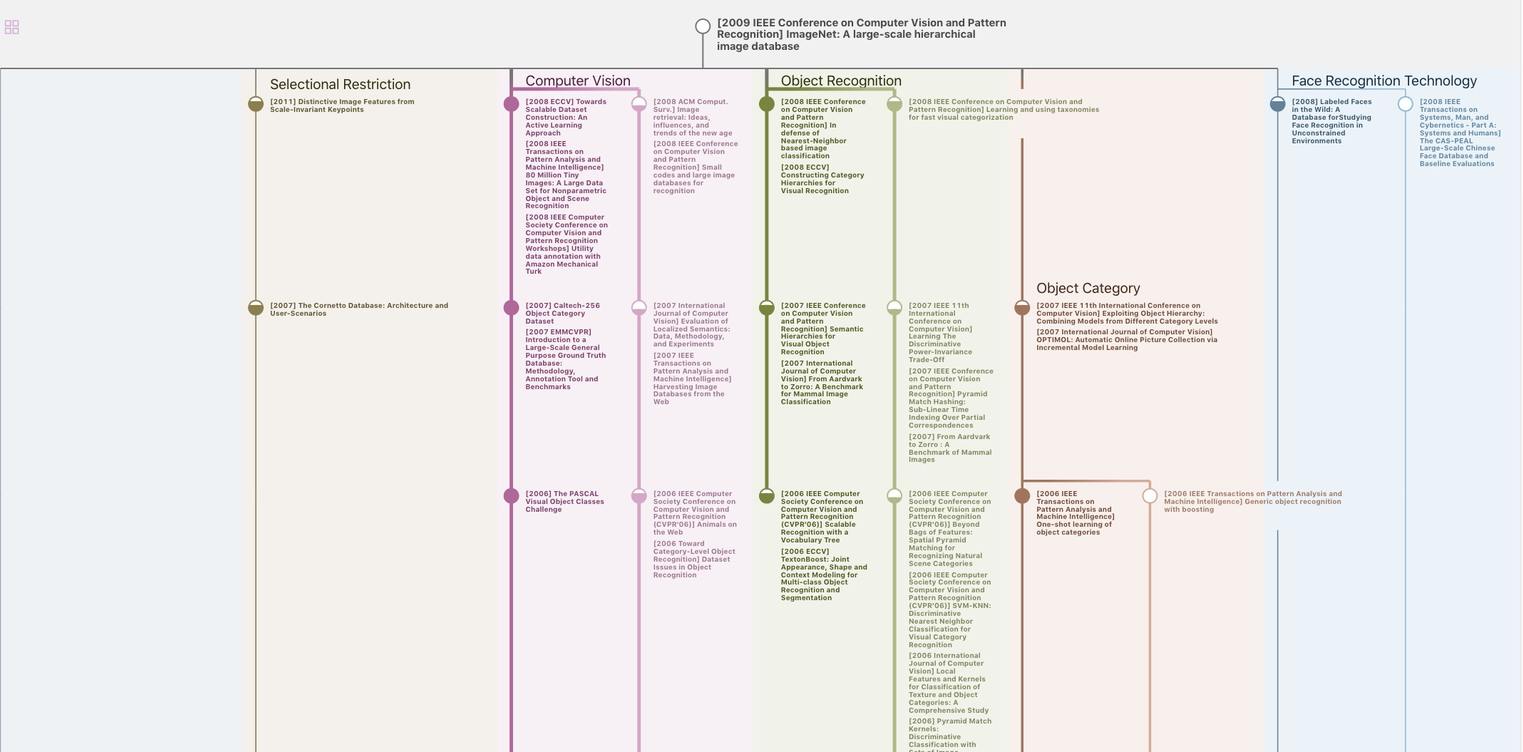
生成溯源树,研究论文发展脉络
Chat Paper
正在生成论文摘要