Explicit Background Endmember Learning for Hyperspectral Anomaly Detection
IEEE Transactions on Instrumentation and Measurement(2024)
Guizhou Univ | Natl Univ Def Technol | Beijing Inst Tracking & Telecommun Technol | Northwest Inst Nucl Technol
Abstract
Hyperspectral anomaly detection (HAD) is challenging especially when anomalies appear in the form of subpixels. Since the spectral signatures of anomalies in mixed pixels are mixed with those of other materials in the background, the anomalies are more difficult to be separated from the background. However, most existing deep learning-based methods neglect the negative effect of mixed pixels on HAD. Therefore, their applications are restricted. To handle this problem, an explicit background endmember learning (EBEL) detection framework is proposed for HAD. Specifically, a spectral-spatial reconstruction error-based background estimation (BE) strategy is employed to obtain the pseudobackground of hyperspectral images (HSIs). Then, the inherent relationships of cross attention mechanism in the Transformer with endmember learning and spectral linear mixing model (LMM) are constructed. Therefore, the cross-attention mechanism is introduced to learn the endmember features from the pseudobackground. Meanwhile, the HSI features can be linearly represented by the background endmember features through a cascaded cross-attention-based module. In this way, the anomalies are detected by the reconstruction errors since the background can be well represented by the background endmember features, while the anomalies cannot. Experimental results on one synthetic dataset and four real datasets demonstrate the superior detection performance of our method to existing state-of-the-art HAD methods.
MoreTranslated text
Key words
Feature extraction,Anomaly detection,Attention mechanisms,Hyperspectral imaging,Transformers,Training,Learning systems,cross attention,endmember learning,hyperspectral image (HSI),mixed pixel
求助PDF
上传PDF
View via Publisher
AI Read Science
AI Summary
AI Summary is the key point extracted automatically understanding the full text of the paper, including the background, methods, results, conclusions, icons and other key content, so that you can get the outline of the paper at a glance.
Example
Background
Key content
Introduction
Methods
Results
Related work
Fund
Key content
- Pretraining has recently greatly promoted the development of natural language processing (NLP)
- We show that M6 outperforms the baselines in multimodal downstream tasks, and the large M6 with 10 parameters can reach a better performance
- We propose a method called M6 that is able to process information of multiple modalities and perform both single-modal and cross-modal understanding and generation
- The model is scaled to large model with 10 billion parameters with sophisticated deployment, and the 10 -parameter M6-large is the largest pretrained model in Chinese
- Experimental results show that our proposed M6 outperforms the baseline in a number of downstream tasks concerning both single modality and multiple modalities We will continue the pretraining of extremely large models by increasing data to explore the limit of its performance
Upload PDF to Generate Summary
Must-Reading Tree
Example
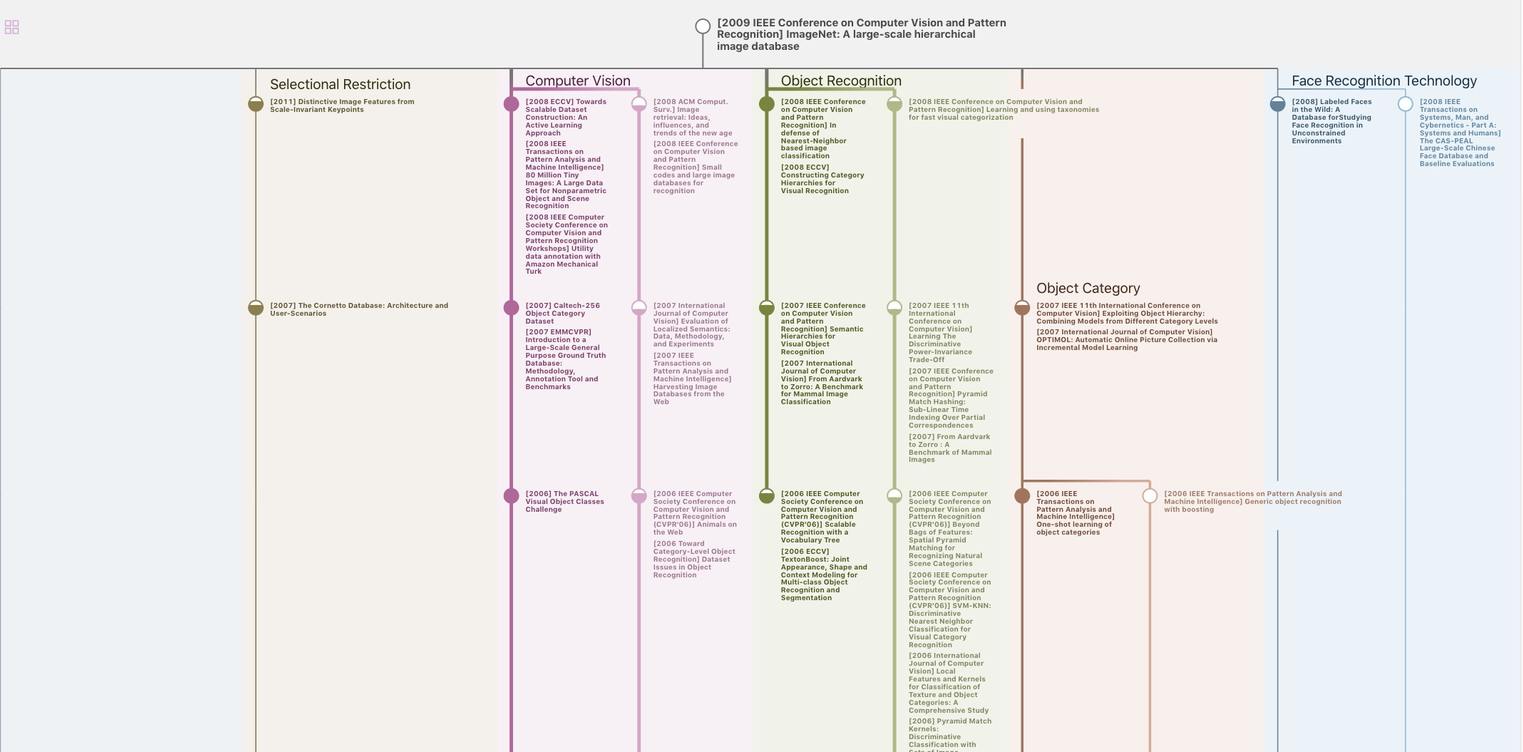
Generate MRT to find the research sequence of this paper
Data Disclaimer
The page data are from open Internet sources, cooperative publishers and automatic analysis results through AI technology. We do not make any commitments and guarantees for the validity, accuracy, correctness, reliability, completeness and timeliness of the page data. If you have any questions, please contact us by email: report@aminer.cn
Chat Paper