Drug Repositioning by Collaborative Learning Based on Graph Convolutional Inductive Network
Future Generation Computer Systems(2024)
摘要
Motivation: Computational drug repositioning is a vital path to improve efficiency of drug discovery, which aims to find potential Drug-Disease Associations (DDAs) to develop new effects of the existing drugs. Many approaches detected novel DDAs from heterogenous network which integrates similar drugs, similar diseases and the known DDAs. However, sparsity of the known DDAs and intrinsic synergic relations on representations of drugs and diseases in the heterogenous network are still the main challenges for DDAs prediction. Results: To address the problems, a novel drug repositioning approach is proposed here. Firstly, a drug similar network is constructed by Gaussian similarity kernel fusion of multisource drug similarities. Likewise, a disease similar network is generated by the same strategy. Secondly, the known DDAs network is extended by a bi-random walk algorithm from the above-mentioned similar networks. Meanwhile, representations of drugs and diseases are learned from their similar networks through graph convolutions and then a DDAs network is induced from the representations. Finally, to discover latent DDAs, the inductive DDAs network is refined iteratively by collaborating with the extended known DDAs network. Comprehensive experimental results show that our method outperforms several state-of-the-art methods for predicting DDAs on indicators including precision, recall, F1-score, MCC, ROC and AUPR. Moreover, case studies suggest that our method is highly effective in practices. The success of our method may be attributed to three aspects: (1) reliable similar relationships of drugs and diseases; (2) enhanced connectivity of the heterogenous network; (3) reasonable collaborative induction on DDAs network. Our method is freely available at https://github.com/BioMLab/DRCLN.
更多查看译文
关键词
Drug-disease associations,Graph convolutional network,Biological network,Collaborative learning
AI 理解论文
溯源树
样例
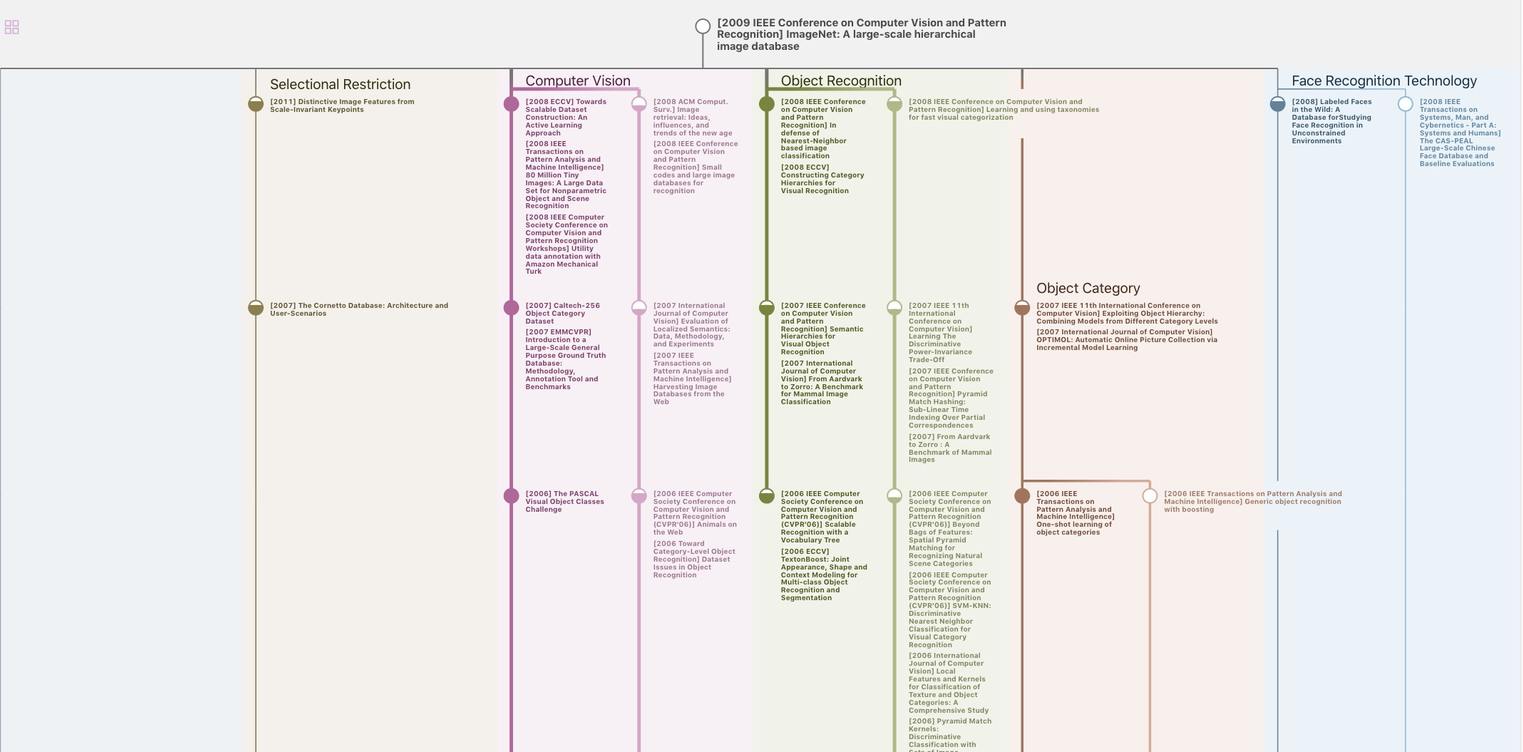
生成溯源树,研究论文发展脉络
Chat Paper
正在生成论文摘要