Forensic discrimination of fiber microspectrophotometry data by resampling and repeating two-sample hypothesis testing
Microchemical Journal(2024)
摘要
Textile fibers are important forensic trace evidence in criminal investigations. Colored textile fibers are often analyzed using non-destructive microspectrophotometry. However, when the spectra of questioned and known samples are very similar, it is difficult to compare the spectra visually. Chemometric methods, such as principal component analysis and agglomerative hierarchical clustering, have been applied to microspectrophotometry data. In this study, we used two-sample hypothesis testing as a chemometric approach for discrimination of fiber samples without reducing or eliminating the dimensionality of the data. Cotton fibers dyed with vat dyes were analyzed by microspectrophotometry. We examined suitable data types and statistical methods for test fiber samples by resampling and repeating hypothesis testing. A combination of spectral data type and statistical method was identified that provided a good balance of type I (false exclusion) and type II (false inclusion) error rates in two-sample hypothesis testing of the fibers.The main advantage of our chemometric approach is that an appropriate method for samples can be selected by using the type I and II error rates. Furthermore, our method can use all the measured data for the calculation without reducing or eliminating the dimensionality of the data, so it is not necessary to reduce variables or smooth the spectrum. This statistical method also requires no training set. Therefore, two-sample hypothesis testing could be applied to fibers of different types, colors, or materials from those used in this study. Resampling and repeating two-sample hypothesis testing technique is promising as a new chemometric approach for comparing microspectrophotometry spectra.
更多查看译文
关键词
Trace evidence,Bootstrap method,High-dimensional data,Error type,First derivative spectrum
AI 理解论文
溯源树
样例
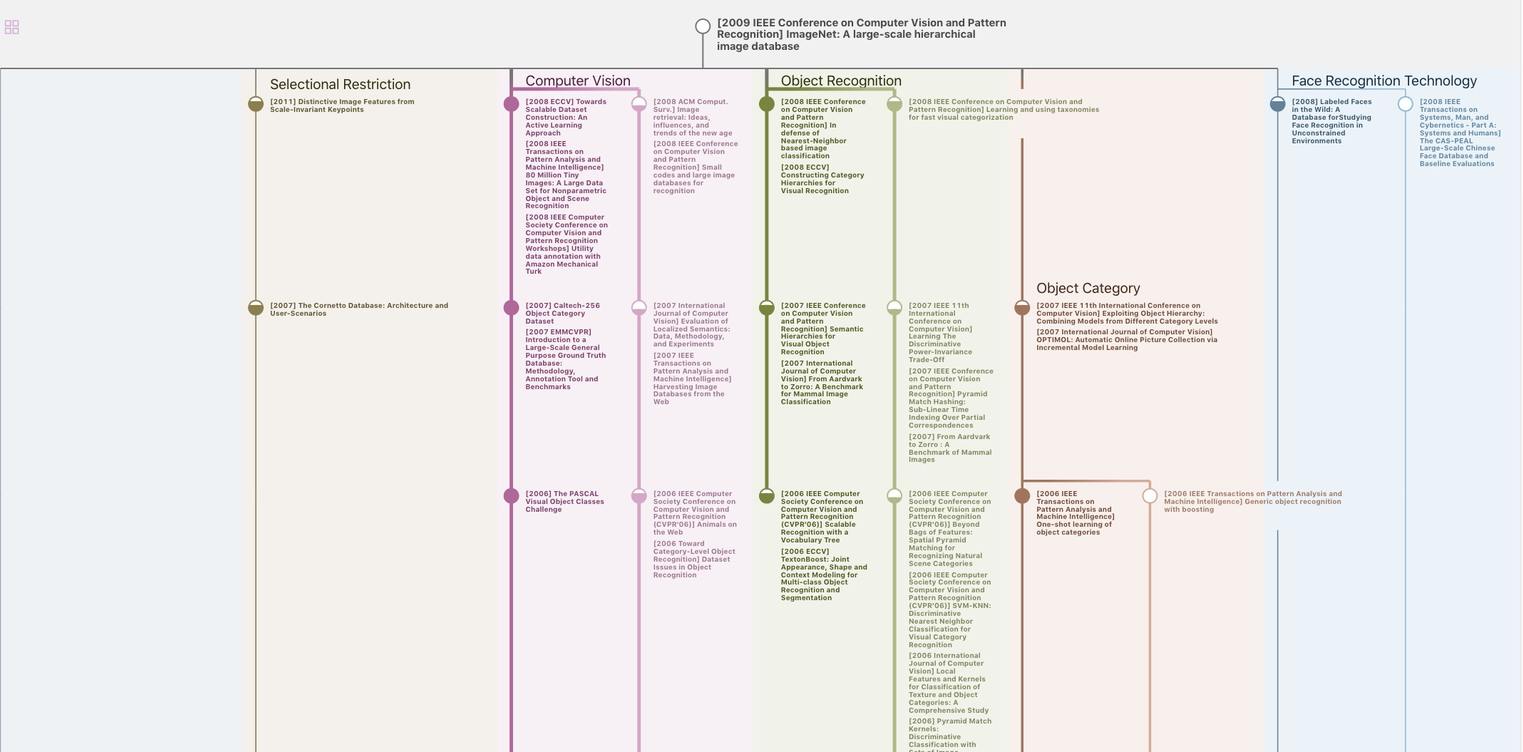
生成溯源树,研究论文发展脉络
Chat Paper
正在生成论文摘要