NeCo: Improving DINOv2's spatial representations in 19 GPU hours with Patch Neighbor Consistency
arxiv(2024)
摘要
We propose sorting patch representations across views as a novel self-supervised learning signal to improve pretrained representations. To this end, we introduce NeCo: Patch Neighbor Consistency, a novel training loss that enforces patch-level nearest neighbor consistency across a student and teacher model, relative to reference batches. Our method leverages a differentiable sorting method applied on top of pretrained representations, such as DINOv2-registers to bootstrap the learning signal and further improve upon them. This dense post-pretraining leads to superior performance across various models and datasets, despite requiring only 19 hours on a single GPU. We demonstrate that this method generates high-quality dense feature encoders and establish several new state-of-the-art results: +5.5 non-parametric in-context semantic segmentation on ADE20k and Pascal VOC, and +7.2
更多查看译文
AI 理解论文
溯源树
样例
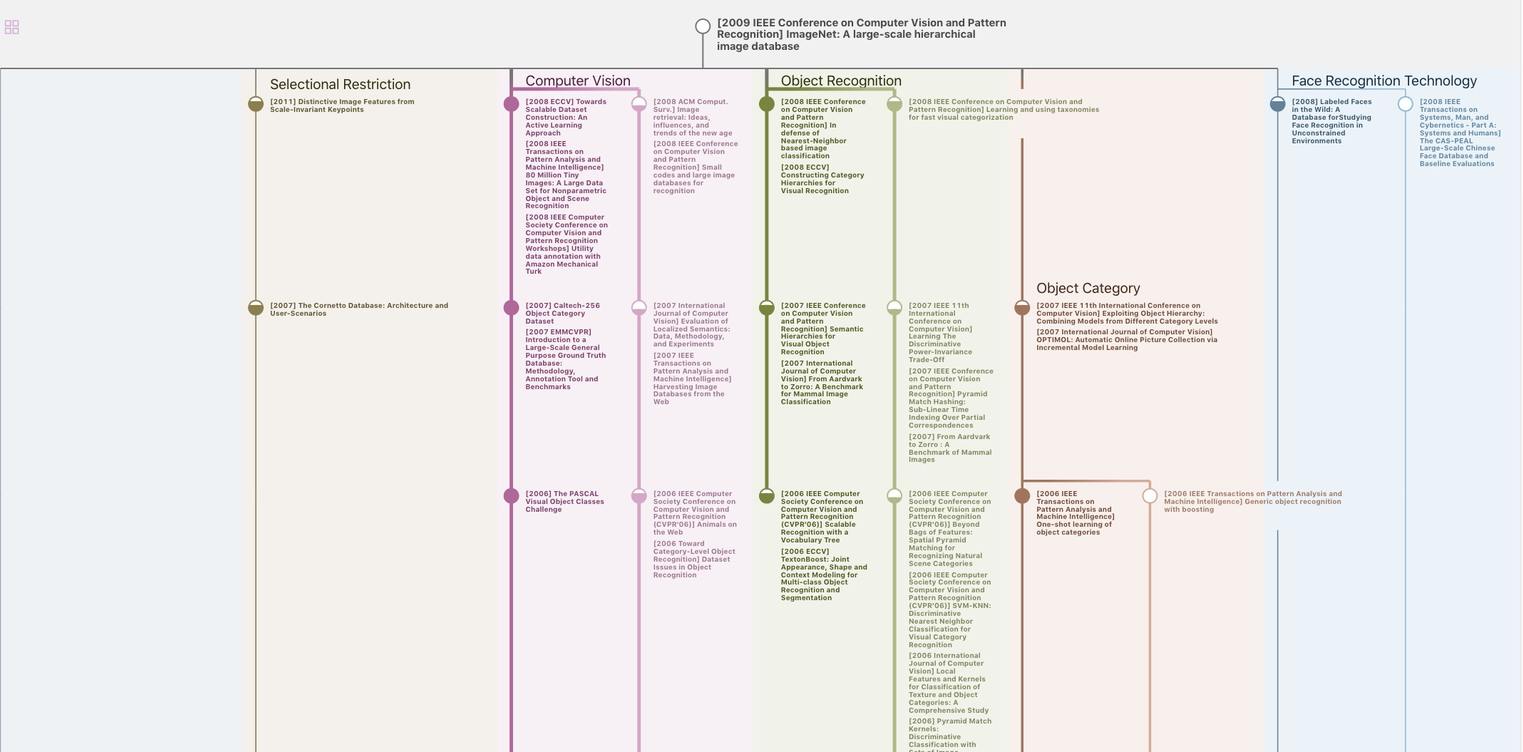
生成溯源树,研究论文发展脉络
Chat Paper
正在生成论文摘要