Classification of High-dimensional Time Series in Spectral Domain using Explainable Features
arxiv(2024)
Abstract
Interpretable classification of time series presents significant challenges in high dimensions. Traditional feature selection methods in the frequency domain often assume sparsity in spectral density matrices (SDMs) or their inverses, which can be restrictive for real-world applications. In this article, we propose a model-based approach for classifying high-dimensional stationary time series by assuming sparsity in the difference between inverse SDMs. Our approach emphasizes the interpretability of model parameters, making it especially suitable for fields like neuroscience, where understanding differences in brain network connectivity across various states is crucial. The estimators for model parameters demonstrate consistency under appropriate conditions. We further propose using standard deep learning optimizers for parameter estimation, employing techniques such as mini-batching and learning rate scheduling. Additionally, we introduce a method to screen the most discriminatory frequencies for classification, which exhibits the sure screening property under general conditions. The flexibility of the proposed model allows the significance of covariates to vary across frequencies, enabling nuanced inferences and deeper insights into the underlying problem. The novelty of our method lies in the interpretability of the model parameters, addressing critical needs in neuroscience. The proposed approaches have been evaluated on simulated examples and the `Alert-vs-Drowsy' EEG dataset.
MoreTranslated text
AI Read Science
Must-Reading Tree
Example
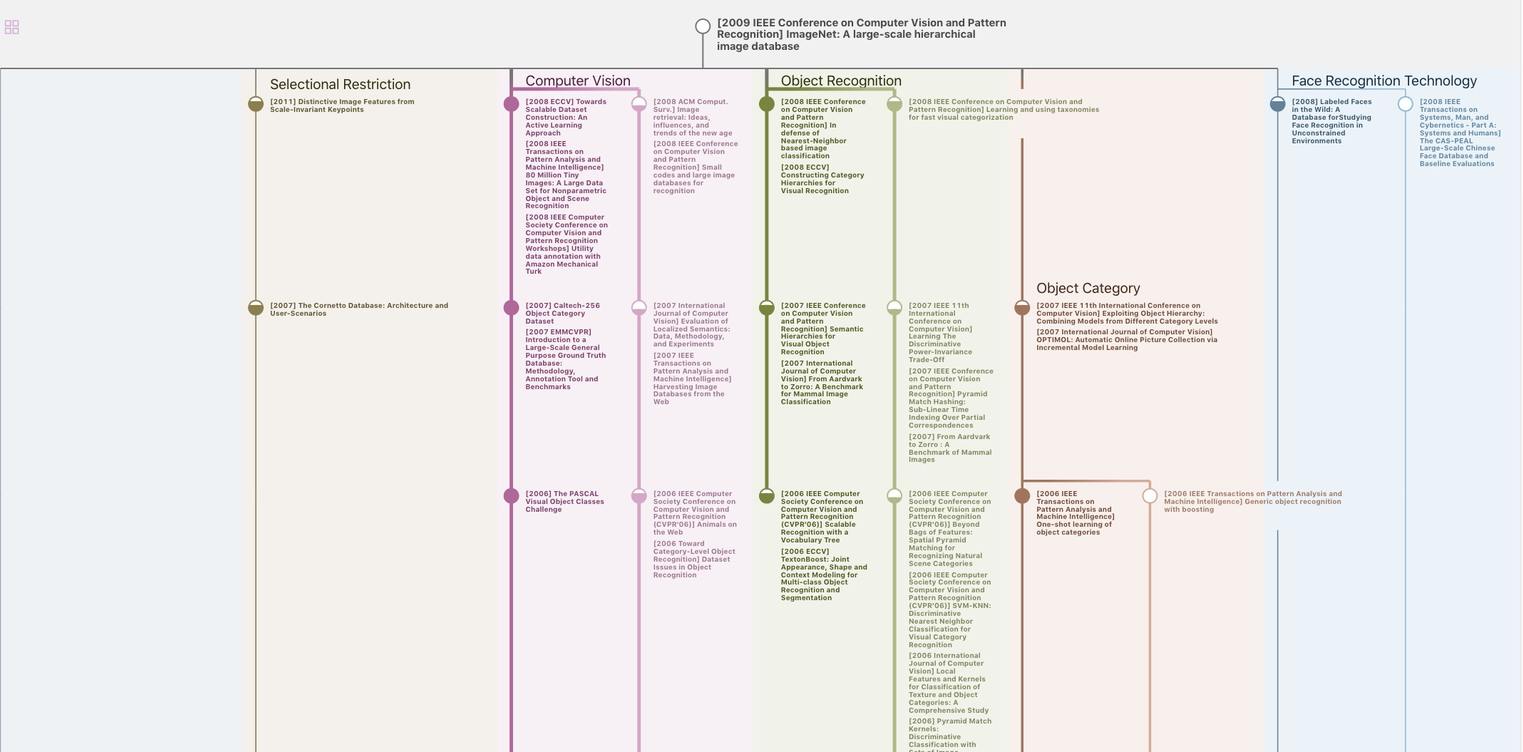
Generate MRT to find the research sequence of this paper
Chat Paper
Summary is being generated by the instructions you defined