Deep-Transfer-Learning-Based Natural Language Processing of Serial Free-Text Computed Tomography Reports for Predicting Survival of Patients With Pancreatic Cancer.
JCO clinical cancer informatics(2024)
摘要
PURPOSE:To explore the predictive potential of serial computed tomography (CT) radiology reports for pancreatic cancer survival using natural language processing (NLP).
METHODS:Deep-transfer-learning-based NLP models were retrospectively trained and tested with serial, free-text CT reports, and survival information of consecutive patients diagnosed with pancreatic cancer in a Korean tertiary hospital was extracted. Randomly selected patients with pancreatic cancer and their serial CT reports from an independent tertiary hospital in the United States were included in the external testing data set. The concordance index (c-index) of predicted survival and actual survival, and area under the receiver operating characteristic curve (AUROC) for predicting 1-year survival were calculated.
RESULTS:Between January 2004 and June 2021, 2,677 patients with 12,255 CT reports and 670 patients with 3,058 CT reports were allocated to training and internal testing data sets, respectively. ClinicalBERT (Bidirectional Encoder Representations from Transformers) model trained on the single, first CT reports showed a c-index of 0.653 and AUROC of 0.722 in predicting the overall survival of patients with pancreatic cancer. ClinicalBERT trained on up to 15 consecutive reports from the initial report showed an improved c-index of 0.811 and AUROC of 0.911. On the external testing set with 273 patients with 1,947 CT reports, the AUROC was 0.888, indicating the generalizability of our model. Further analyses showed our model's contextual interpretation beyond specific phrases.
CONCLUSION:Deep-transfer-learning-based NLP model of serial CT reports can predict the survival of patients with pancreatic cancer. Clinical decisions can be supported by the developed model, with survival information extracted solely from serial radiology reports.
更多查看译文
AI 理解论文
溯源树
样例
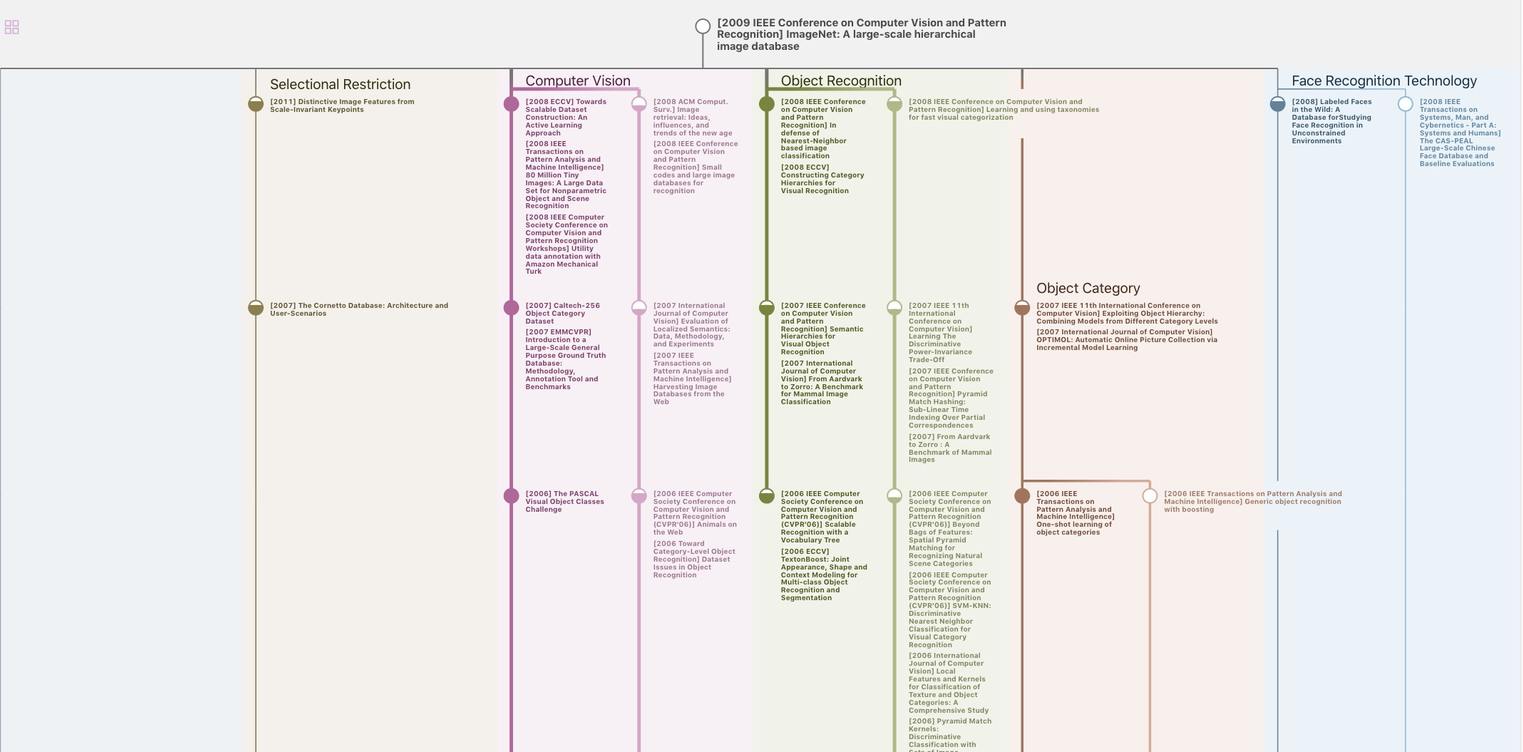
生成溯源树,研究论文发展脉络
Chat Paper
正在生成论文摘要