Use of explainable AI on slit-lamp images of anterior surface of eyes to diagnose allergic conjunctival diseases
Allergology International(2024)
摘要
Background
Artificial intelligence (AI) is a promising new technology that has the potential of diagnosing allergic conjunctival diseases (ACDs). However, its development is slowed by the absence of a tailored image database and explainable AI models. Thus, the purpose of this study was to develop an explainable AI model that can not only diagnose ACDs but also present the basis for the diagnosis.
Methods
A dataset of 4942 slit-lamp images from 10 ophthalmological institutions across Japan were used as the image database. A sequential pipeline of segmentation AI was constructed to identify 12 clinical findings in 1038 images of seasonal and perennial allergic conjunctivitis (AC), atopic keratoconjunctivitis (AKC), vernal keratoconjunctivitis (VKC), giant papillary conjunctivitis (GPC), and normal subjects. The performance of the pipeline was evaluated by determining its ability to obtain explainable results through the extraction of the findings. Its diagnostic accuracy was determined for 4 severity-based diagnosis classification of AC, AKC/VKC, GPC, and normal.
Results
Segmentation AI pipeline efficiently extracted crucial ACD indicators including conjunctival hyperemia, giant papillae, and shield ulcer, and offered interpretable insights. The AI pipeline diagnosis had a high diagnostic accuracy of 86.2%, and that of the board-certified ophthalmologists was 60.0%. The pipeline had a high classification performance, and the area under the curve (AUC) was 0.959 for AC, 0.905 for normal subjects, 0.847 for GPC, 0.829 for VKC, and 0.790 for AKC.
Conclusions
An explainable AI model created by a comprehensive image database can be used for diagnosing ACDs with high degree of accuracy.
更多查看译文
关键词
Allergic conjunctivitis,Artificial intelligence,Atopic keratoconjunctivitis,Deep learning,Vernal keratoconjunctivitis
AI 理解论文
溯源树
样例
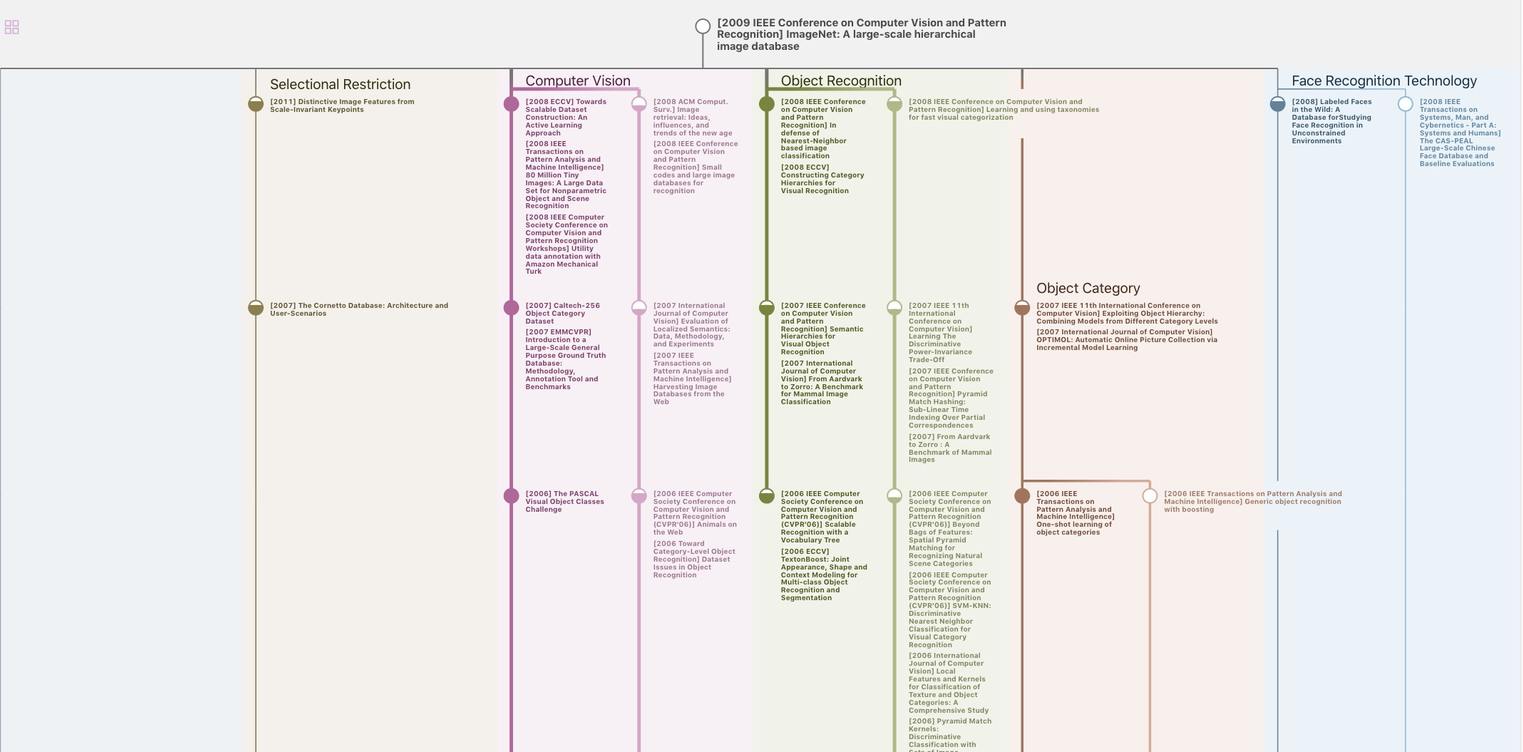
生成溯源树,研究论文发展脉络
Chat Paper
正在生成论文摘要