High-Performance, Accurate Large-Scale Quantum Chemistry Calculations on GPU Supercomputers using Coulomb-Perturbed Fragmentation.
International Conference on Parallel Processing(2024)
摘要
Predicting the chemico-physical properties of large molecular systems is a formidable challenge in chemistry and materials science. Traditional quantum mechanical methods, while accurate, have impractical scaling for large molecules with thousands of atoms, which are crucial in the development of novel therapeutics, catalysts, and nanomaterials. To address this, molecular fragmentation algorithms have been proposed to improve scalability and enable extensive parallelism. In this article, we introduce a significant enhancement to the Fragment Molecular Orbital (FMO) method, termed the Coulomb-Perturbed Fragmentation (CPF) method. CPF incorporates algorithmic improvements and implementation enhancements to optimize performance on heterogeneous computing systems equipped with a large number of GPUs. Key developments include a significant simplification of iteratitve self-consistent field (SCF) algorithm, advanced data management through a one-sided communication model, topology-aware optimizations, and a hybrid communication strategy for intra-group exchanges. Moreover, CPF integrates a distributed dynamic multi-layer load balancing scheme to optimise fragment distribution and workload management across nodes and GPUs. Performance evaluations on a 420-atom benzene molecule system comprising 35 fragments reveal that CPF outperforms existing GPU/CPU-based FMO algorithms in both efficiency and accuracy. When deployed on the Gadi supercomputer, CPF achieves over 97% parallel efficiency on 20 nodes, with scalability maintaining above 98% and 90% efficiency in weak-scaling tests for smaller and larger systems, respectively. Notably, CPF matches or exceeds the computational accuracy of conventional FMO methods, marking a substantial progress in the field of computational chemistry for fragmentation-based large-scale molecular modelling.
更多查看译文
AI 理解论文
溯源树
样例
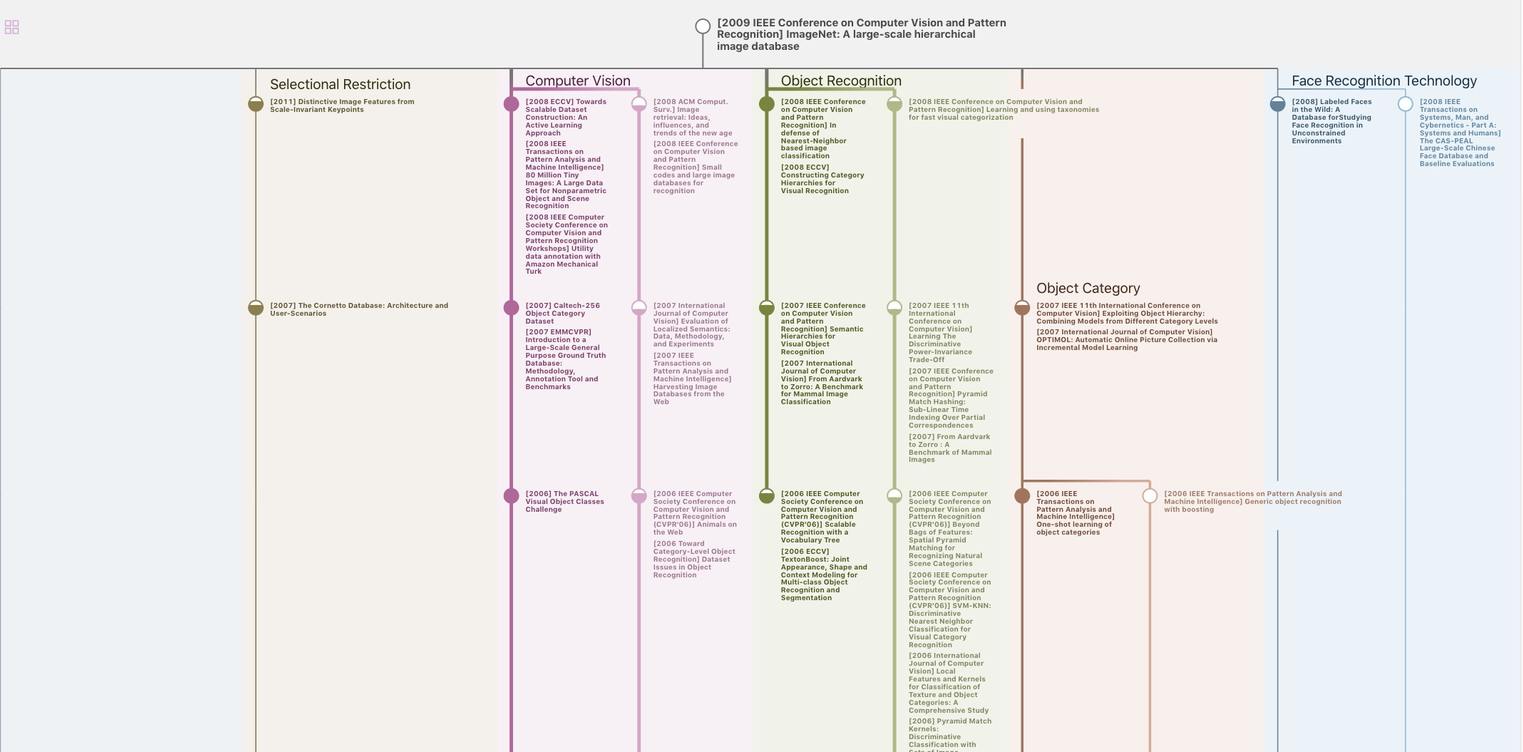
生成溯源树,研究论文发展脉络
Chat Paper
正在生成论文摘要