The Blind and the Elephant: A Preference-aware Edge Video Analytics Scheduler for Maximizing System Benefit.
International Conference on Parallel Processing(2024)
Abstract
Video analytics is the killer workload in edge computing, which involves the scheduler’s complex decisions to balance analysis performance (latency and accuracy) and resource consumption (network, computation, and energy). Traditional schedulers address this as a single-objective optimization problem with fixed weights, unable to precisely capture unknown system preferences due to intricate pricing rules across various service levels and resource costs, consequently leading to suboptimal system benefit like monetary gain. In this paper, we propose a Bayesian optimization-driven multi-objective scheduler, PaMO, that can proactively explore the system pricing preference by pairwise comparing outcome vectors of all objectives. Moreover, PaMO designs a heuristic scheduling algorithm with a zero-delay jitter guarantee to avoid performance degradation caused by resource contention and uses a revised Bayesian optimization algorithm to make video configuration and scheduling decisions. Experiments on real video analytics workloads show that PaMO can achieve up to 53.9% benefit gain compared to state-of-the-art scheduling methods.
MoreTranslated text
AI Read Science
Must-Reading Tree
Example
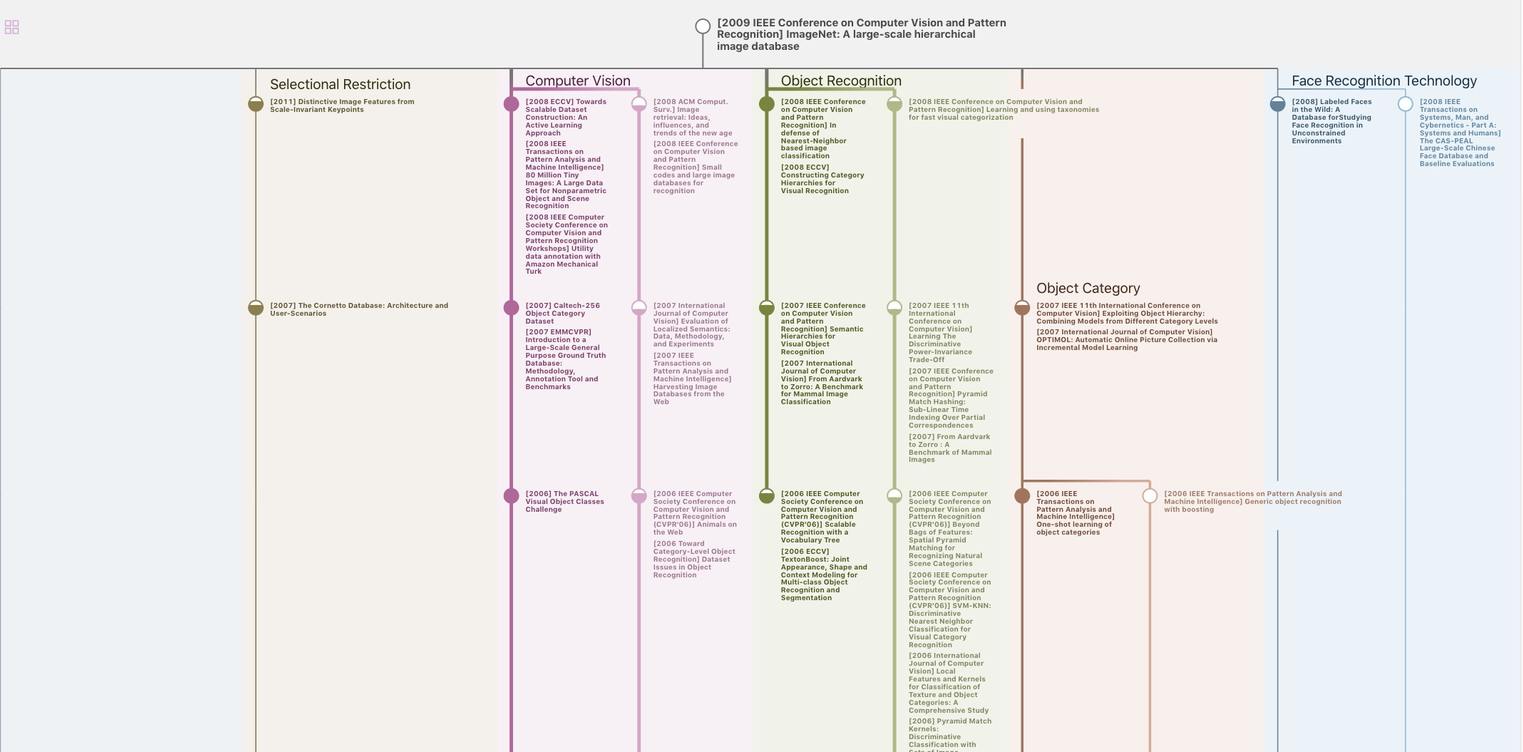
Generate MRT to find the research sequence of this paper
Chat Paper
Summary is being generated by the instructions you defined