Global Context Relation-Guided Feature Aggregation Network for Salient Object Detection in Optical Remote Sensing Images
Remote Sensing(2024)
摘要
With the rapid development of deep neural networks, salient object detection has achieved great success in natural images. However, detecting salient objects from optical remote sensing images still remains a challenging task due to the diversity of object types, scale, shape and orientation variations, as well as cluttered backgrounds. Therefore, it is impractical to directly leverage methods designed for natural images to detect salient objects in optical remote sensing images. In this work, we present an end-to-end deep neural network for salient object detection in optical remote sensing images via global context relation-guided feature aggregation. Since the objects in remote sensing images often have a scattered distribution, we design a global context relation module to capture the global relationships between different spatial positions. In order to effectively integrate low-level appearance features as well as high-level semantic features for enhancing the final performance, we develop a feature aggregation module with the global context relation information as guidance and embed it into the backbone network to refine the deep features in a progressive manner. Instead of using traditional binary cross entropy as a training loss which treats all pixels equally, we design a weighted binary cross entropy to capture local surrounding information of different pixels. Extensive experiments on three public datasets are conducted to validate the efficiency of the proposed network and the results demonstrate that our proposed method consistently outperforms other competitors.
更多查看译文
关键词
salient object detection,optical remote sensing images,global context relation,multi-level feature aggregation
AI 理解论文
溯源树
样例
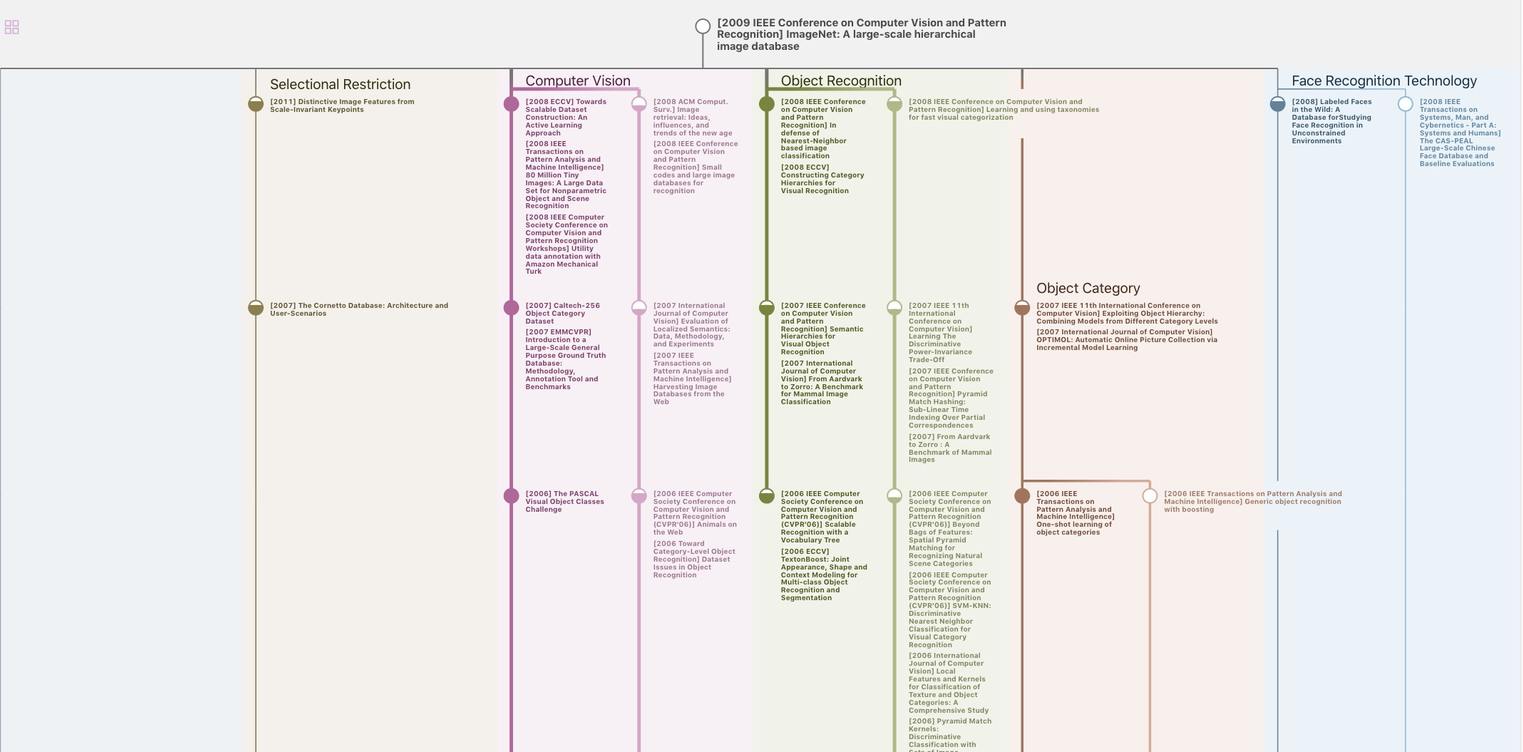
生成溯源树,研究论文发展脉络
Chat Paper
正在生成论文摘要