LSTM $+$ Transformer Real-Time Crash Risk Evaluation Using Traffic Flow and Risky Driving Behavior Data
IEEE TRANSACTIONS ON INTELLIGENT TRANSPORTATION SYSTEMS(2024)
摘要
Crash risk evaluation studies mainly established the relationship between the macro traffic status and crashes. However, the impact of risky driving behavior, a significant factor in crashes, has not been thoroughly investigated due to the data collection limitations of fixed detectors. In this study, the risky driving behavior data generated by Connected Vehicle (CV) techniques was introduced along with traffic flow data to develop the crash risk evaluation model. An LSTM $+$ Transformer approach was developed, in which the Transformer could extract the non-aggregated spatial-temporal features of risky driving behaviors and LSTM learn the temporal patterns of traffic flow. An ensemble layer was proposed to integrate the macro traffic status features and micro driving behavior, and automatically fit their weights to optimize crash risk evaluation performance. Data from a Chinese freeway was used for empirical analysis. The results show that the proposed LSTM $+$ Transformer model achieved high model accuracy (77.7%), recall (68.6%), and AUC (0.785), with average improvement of between 5.34%, 15.69%, and 5.97%, respectively, compared to existing LSTM, XGBoost, SVM and Logistic Regression (LR) models. Moreover, utilizing risky driving behavior data by incorporating the macro traffic status has proved to capture the pre-crash traffic flow turbulence more precisely. The model results explained by SHapley Additive exPlanations (SHAP) reveal that higher frequency, longer duration and greater acceleration of risky braking behavior increase the number of road vehicles affected, thereby heightening the crash risks. These findings could help the deployment of proactive traffic management and target CV control strategies to reduce crashes.
更多查看译文
关键词
Accidents,Detectors,Transformers,Traffic control,Long short term memory,Data models,Roads,Crash risk evaluation,risky driving behavior,Transformer,proactive traffic management
AI 理解论文
溯源树
样例
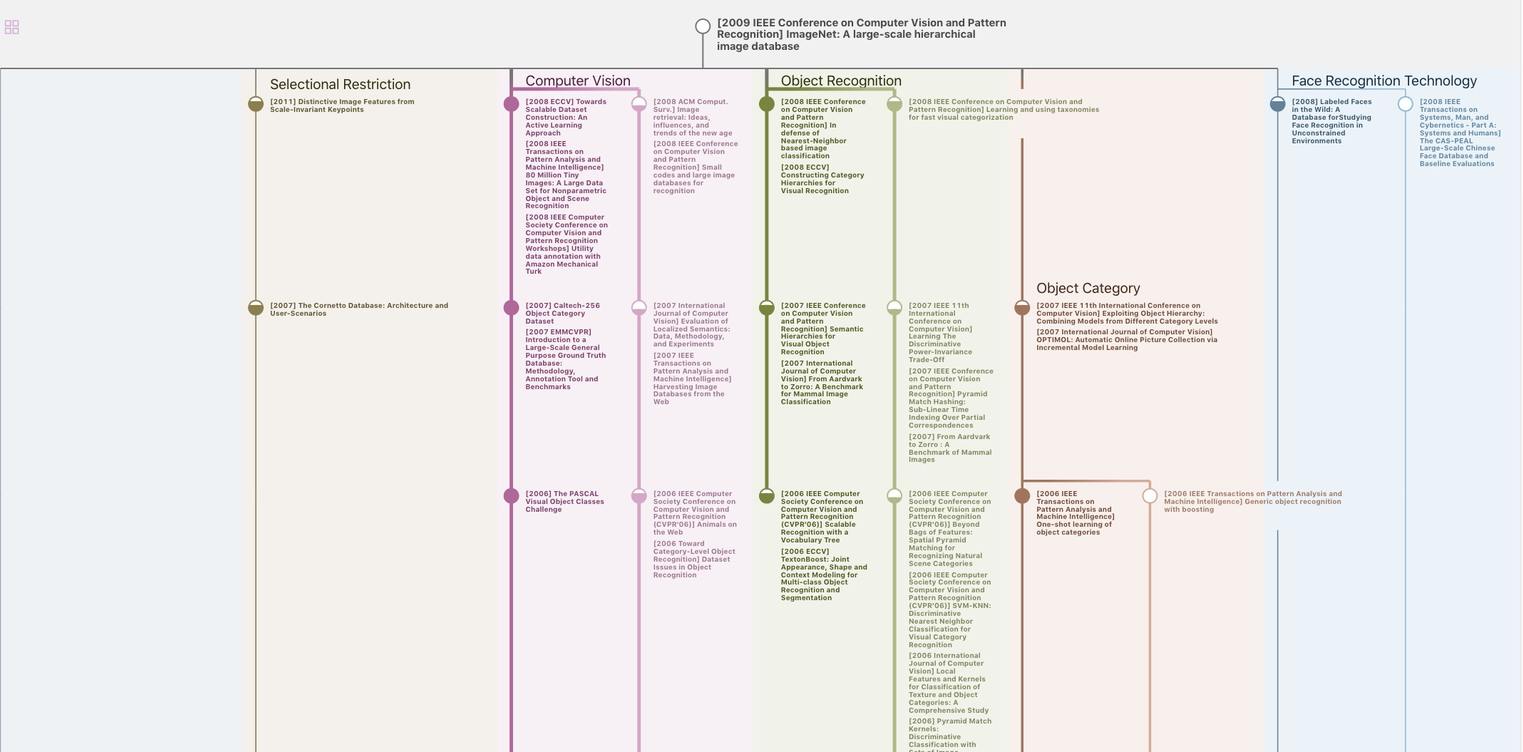
生成溯源树,研究论文发展脉络
Chat Paper
正在生成论文摘要