Multi-omics Analysis Reveals Biomarkers That Contribute to Biological Age Rejuvenation in Response to Therapeutic Plasma Exchange
medrxiv(2024)
Buck Institute for Research on Aging | Global Apheresis
Abstract
The rapid increase in the proportion of older adults worldwide poses a huge challenge for healthcare systems. Currently, age-related chronic diseases account for over 90% of annual healthcare expenditures (more than $4.1 trillion) in the US alone. Thus, new therapies to improve healthspan and reduce the burden of chronic disease are needed. Herein, we conducted a phase 3 controlled clinical trial to evaluate the biological age (BA) effects of different therapeutic plasma exchange (TPE) modalities in ambulatory individuals. We longitudinally profiled the subjects to measure changes in the epigenome, proteome, metabolome, glycome, and shifts in immune cell composition (cytomics). We demonstrate that administering TPE supplemented with intravenous immunoglobulin (IVIG) (TPE-IVIG) on a biweekly regime (two sessions in the first week, followed by a three-week break) is a robust therapy for BA rejuvenation. This intervention induced coordinated cellular and molecular omics responses, reversed age-related immune decline, and modulated key cellular senescence-associated proteins. Integrative analysis revealed baseline biomarkers associated with successful responses, indicating that TPE-IVIG treatment benefits those with a poorer baseline health status. In summary, this is the first multi-omics study to examine the effectiveness of various TPE modalities, which demonstrate biological age rejuvenation and the molecular features associated with this rejuvenation. ### Competing Interest Statement The authors have declared no competing interest. ### Clinical Trial NCT06534450 ### Funding Statement This study was funded by Circulate, Inc ### Author Declarations I confirm all relevant ethical guidelines have been followed, and any necessary IRB and/or ethics committee approvals have been obtained. Yes The details of the IRB/oversight body that provided approval or exemption for the research described are given below: Ethics committee of Diagnostics IRB gave ethical approval for this work. I confirm that all necessary patient/participant consent has been obtained and the appropriate institutional forms have been archived, and that any patient/participant/sample identifiers included were not known to anyone (e.g., hospital staff, patients or participants themselves) outside the research group so cannot be used to identify individuals. Yes I understand that all clinical trials and any other prospective interventional studies must be registered with an ICMJE-approved registry, such as ClinicalTrials.gov. I confirm that any such study reported in the manuscript has been registered and the trial registration ID is provided (note: if posting a prospective study registered retrospectively, please provide a statement in the trial ID field explaining why the study was not registered in advance). Yes I have followed all appropriate research reporting guidelines, such as any relevant EQUATOR Network research reporting checklist(s) and other pertinent material, if applicable. Yes The raw data and complete mass spectrometry data sets have been uploaded to the Mass Spectrometry Interactive Virtual Environment (MassIVE) repository, which is maintained by the Center for Computational Mass Spectrometry at the University of California San Diego. Raw data and complete MS data sets have been uploaded to the MassIVE repository and can be downloaded using the following link: https://massive.ucsd.edu/ProteoSAFe/dataset.jsp?task=945e8acb15054ae895937c3b84d926b9 (MassIVE ID number: MSV000095275; ProteomeXchange ID: PXD053805).
MoreTranslated text
PDF
View via Publisher
AI Read Science
AI Summary
AI Summary is the key point extracted automatically understanding the full text of the paper, including the background, methods, results, conclusions, icons and other key content, so that you can get the outline of the paper at a glance.
Example
Background
Key content
Introduction
Methods
Results
Related work
Fund
Key content
- Pretraining has recently greatly promoted the development of natural language processing (NLP)
- We show that M6 outperforms the baselines in multimodal downstream tasks, and the large M6 with 10 parameters can reach a better performance
- We propose a method called M6 that is able to process information of multiple modalities and perform both single-modal and cross-modal understanding and generation
- The model is scaled to large model with 10 billion parameters with sophisticated deployment, and the 10 -parameter M6-large is the largest pretrained model in Chinese
- Experimental results show that our proposed M6 outperforms the baseline in a number of downstream tasks concerning both single modality and multiple modalities We will continue the pretraining of extremely large models by increasing data to explore the limit of its performance
Try using models to generate summary,it takes about 60s
Must-Reading Tree
Example
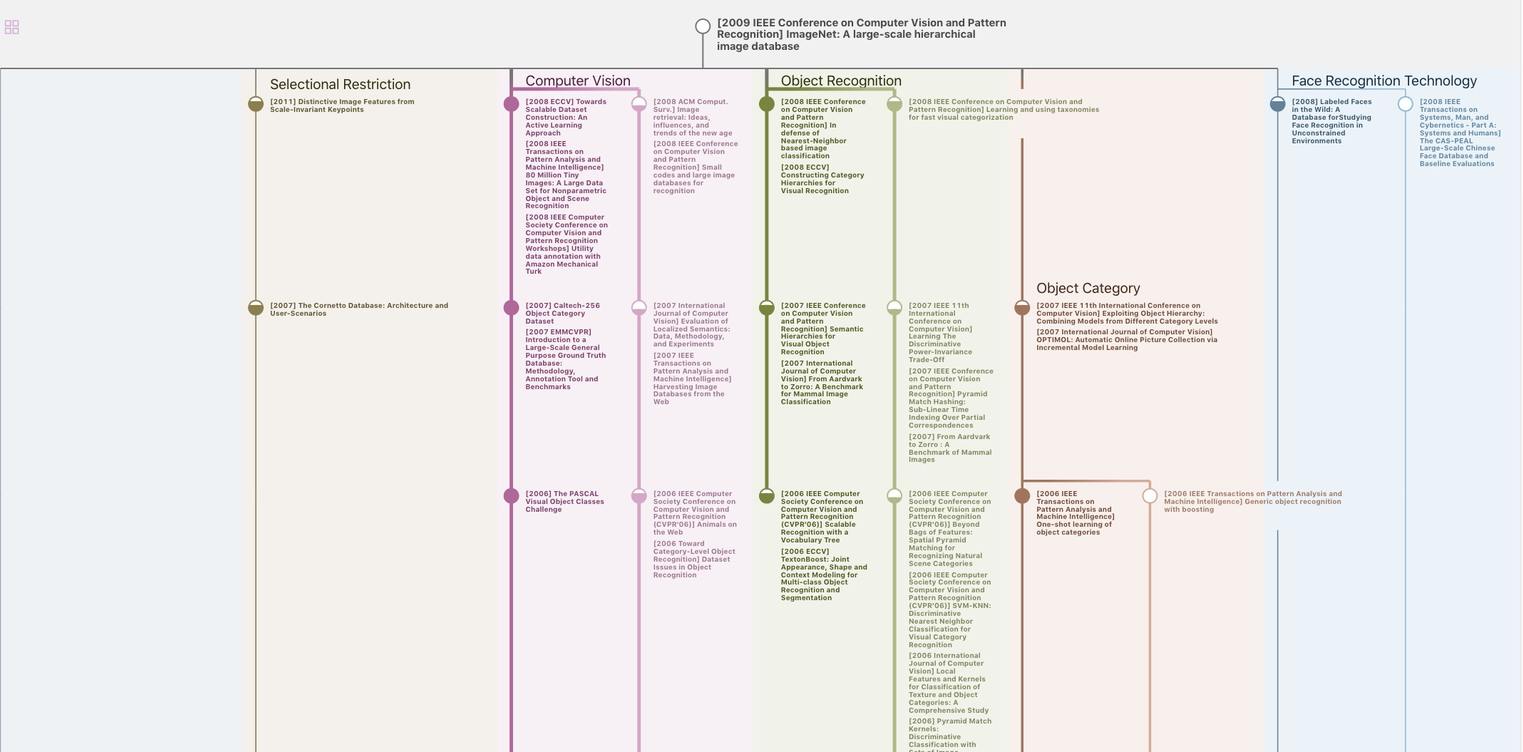
Generate MRT to find the research sequence of this paper
Data Disclaimer
The page data are from open Internet sources, cooperative publishers and automatic analysis results through AI technology. We do not make any commitments and guarantees for the validity, accuracy, correctness, reliability, completeness and timeliness of the page data. If you have any questions, please contact us by email: report@aminer.cn
Chat Paper
去 AI 文献库 对话