Grow Your Limits: Continuous Improvement with Real-World RL for Robotic Locomotion
ICRA 2024(2024)
摘要
Deep reinforcement learning can enable robots to autonomously acquire complex behaviors such as legged locomotion. However, RL in the real world is complicated by constraints on efficiency, safety, and overall training stability, which limits its practical applicability. We present APRL, a policy regularization framework that modulates the robot’s exploration throughout training, striking a balance between flexible improvement potential and focused, efficient exploration. APRL enables a quadrupedal robot to efficiently learn to walk entirely in the real world within minutes and continue to improve with more training where prior work saturates in performance. We demonstrate that continued training with APRL results in a policy that is substantially more capable of navigating challenging situations and adapts to changes in dynamics. Videos and code to reproduce our results are available at: https://sites.google.com/berkeley.edu/aprl
更多查看译文
关键词
Reinforcement Learning,Deep Learning Methods
AI 理解论文
溯源树
样例
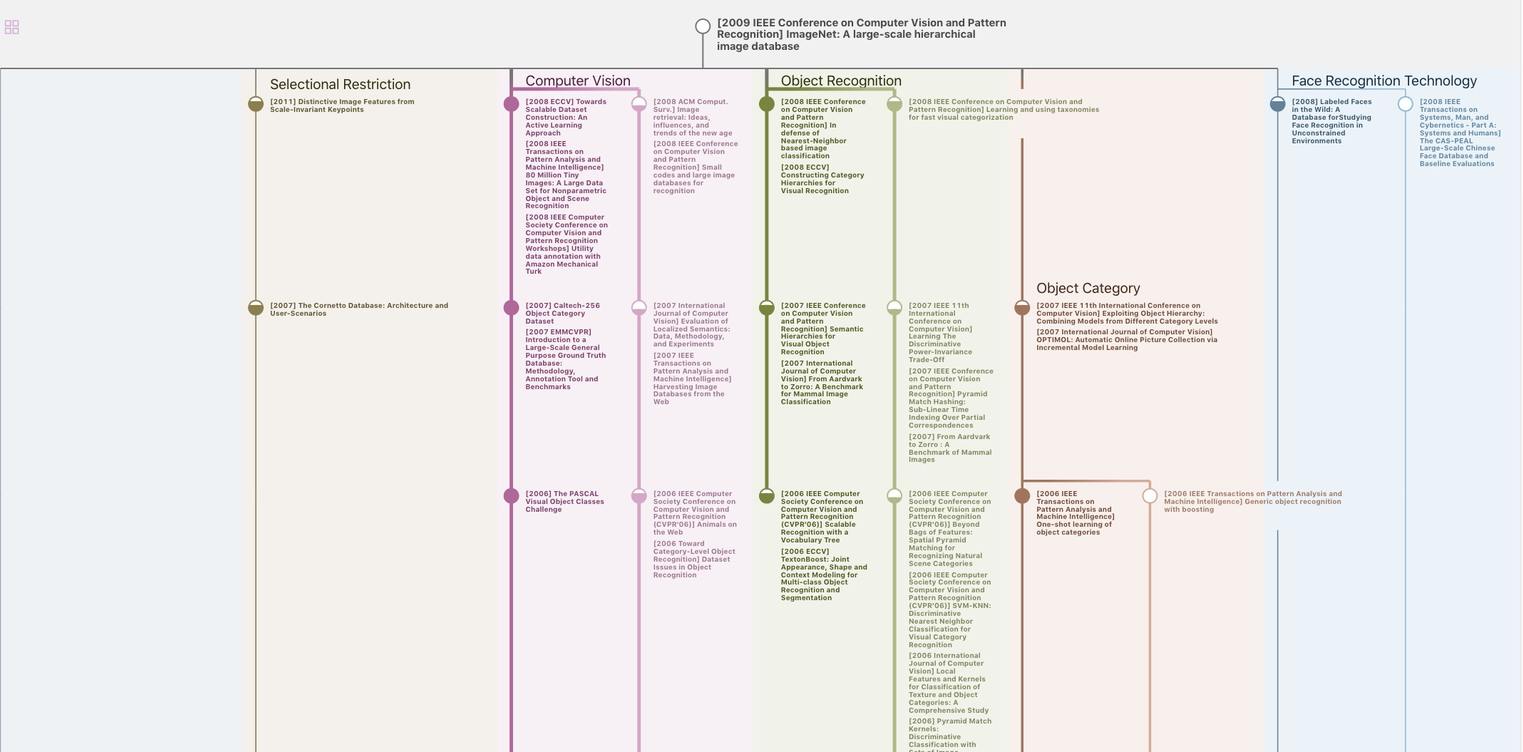
生成溯源树,研究论文发展脉络
Chat Paper
正在生成论文摘要