Many-objective Artificial Hummingbird Algorithm: an Effective Many-Objective Algorithm for Engineering Design Problems
JOURNAL OF COMPUTATIONAL DESIGN AND ENGINEERING(2024)
摘要
Many-objective optimization presents unique challenges in balancing diversity and convergence of solutions. Traditional approaches struggle with this balance, leading to suboptimal solution distributions in the objective space especially at higher number of objectives. This necessitates the need for innovative strategies to adeptly manage these complexities. This study introduces a Many-Objective Artificial Hummingbird Algorithm (MaOAHA), an advanced evolutionary algorithm designed to overcome the limitations of existing many-objective optimization methods. The objectives are to improve convergence rates, maintain solution diversity, and achieve a uniform distribution in the objective space. MaOAHA implements information feedback mechanism (IFM), reference point-based selection and association, non-dominated sorting, and niche preservation. The IFM utilizes historical data from previous generations to inform the update process, thereby improving the algorithm's the exploration and exploitation capabilities. Reference point-based selection, along with non-dominated sorting, ensures solutions are both close to the Pareto front and evenly spread in the objective space. Niche preservation and density estimation strategies are employed to maintain diversity and prevent overcrowding. The comprehensive experimental analysis benchmarks MaOAHA against four leading algorithms viz. Many-Objective Gradient-Based Optimizer, Many-Objective Particle Swarm Optimizer, Reference Vector Guided Evolutionary Algorithm, and Nondominated Sorting Genetic Algorithm III. The DTLZ1-DTLZ7 benchmark sets with four, six, and eight objectives and five real-world problems (RWMaOP1-RWMaOP5) are considered for performance assessment of the selected algorithms. The results demonstrate that internal parameter-free MaOAHA significantly outperforms its counterparts, achieving better generational distance by up to 52.38%, inverse generational distance by up to 38.09%, spacing by up to 56%, spread by up to 71.42%, hypervolume by up to 44%, and runtime by up to 52%. These metrics affirm the MaOAHA's capability to enhance the decision-making processes through its adept balance of convergence, diversity, and uniformity. Graphical Abstract
更多查看译文
关键词
many-objective optimization,multi-objective optimization,diversity preservation,artificial hummingbird algorithm,non-dominated sorting
AI 理解论文
溯源树
样例
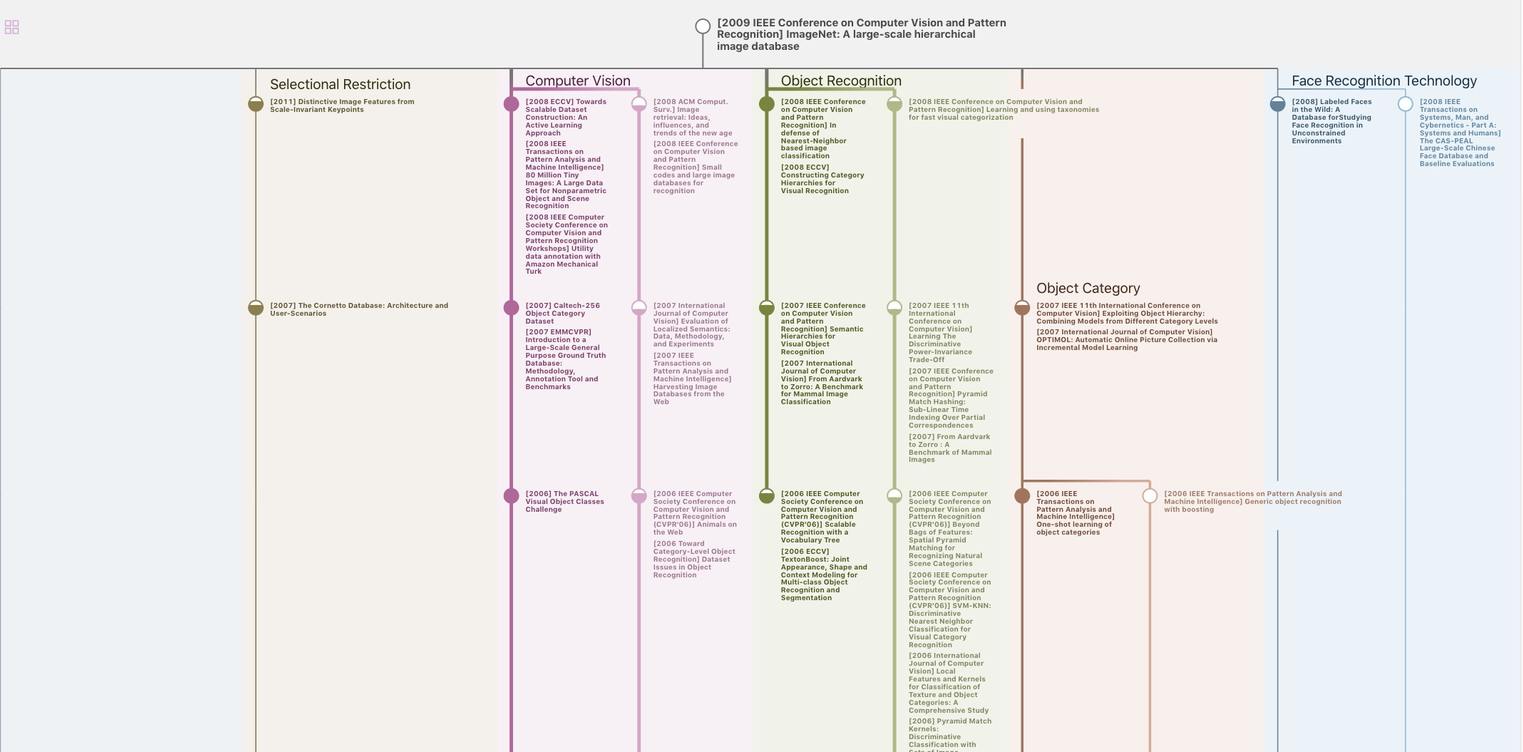
生成溯源树,研究论文发展脉络
Chat Paper
正在生成论文摘要