Group inference of high-dimensional single-index models
JOURNAL OF NONPARAMETRIC STATISTICS(2024)
摘要
For the supervised and semi-supervised settings, a group inference method is proposed for regression parameters in high-dimensional semi-parametric single-index models with an unknown random link function. The inference procedure is based on least squares, which can be extended to other general convex loss functions. The proposed test statistics are weighted quadratic forms of the regression parameter estimates, in which the weight could be a non-random matrix or the sample covariance matrix of the covariates. The proposed method could detect dense but weak signals and deal with high correlation of covariates inside the group. A 'contaminated test statistic' is established in the semi-supervised regime to decrease the variance. The asymptotic properties of the resulting estimators are established. The finite-sample behaviour of the proposed method is evaluated through extensive simulation studies. Applications to two genomic datasets are provided.
更多查看译文
关键词
Group inference,high dimension,hypothesis testing,single-index models,semi-supervised learning
AI 理解论文
溯源树
样例
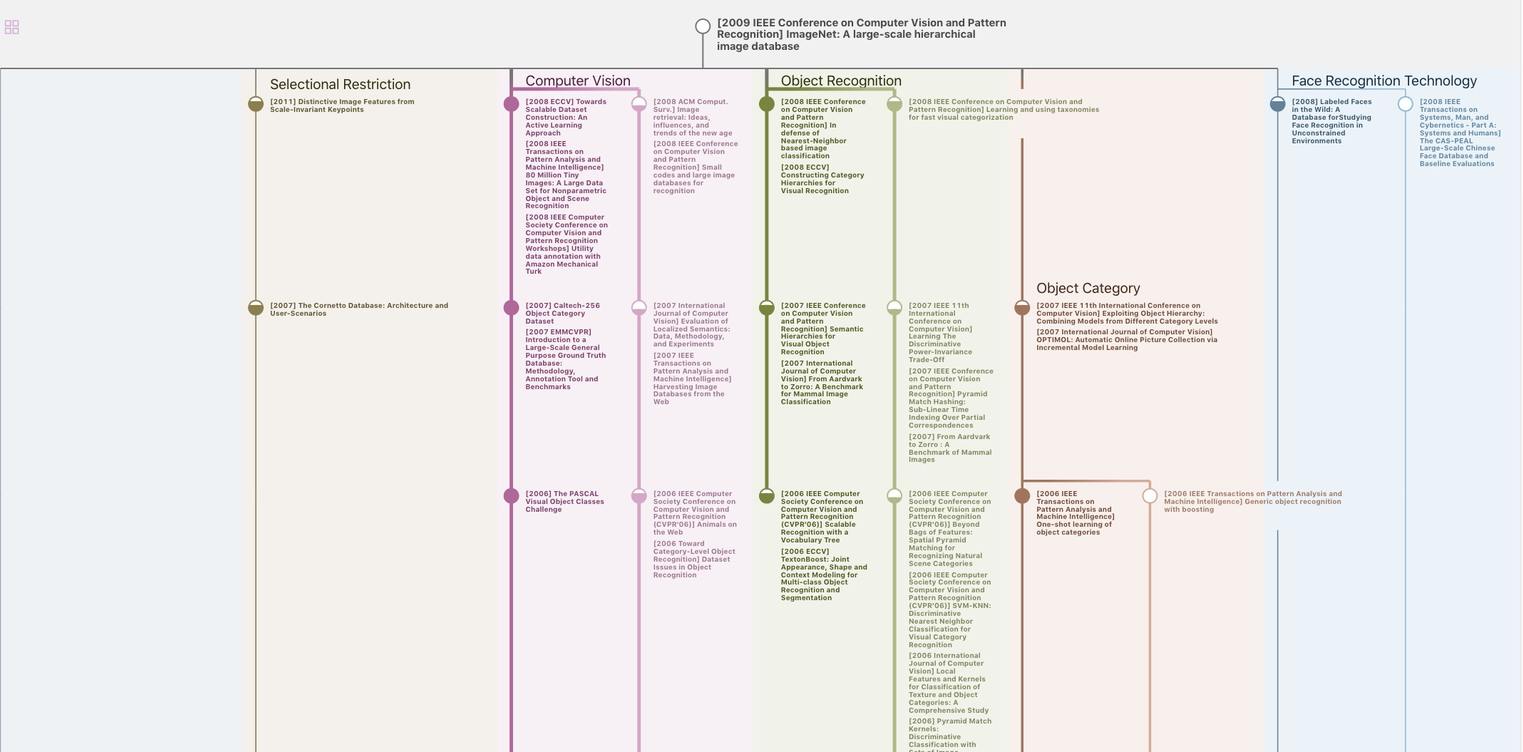
生成溯源树,研究论文发展脉络
Chat Paper
正在生成论文摘要