Training on the Fly: On-device Self-supervised Learning Aboard Nano-drones Within 20 Mw
IEEE Transactions on Computer-Aided Design of Integrated Circuits and Systems(2024)
摘要
Miniaturized cyber-physical systems (CPSes) powered by tiny machine learning (TinyML), such as nano-drones, are becoming an increasingly attractive technology. Their small form factor (i.e., 10cm diameter) ensures vast applicability, ranging from the exploration of narrow disaster scenarios to safe human-robot interaction. Simple electronics make these CPSes inexpensive, but strongly limit the computational, memory, and sensing resources available on board. In real-world applications, these limitations are further exacerbated by domain shift. This fundamental machine learning problem implies that model perception performance drops when moving from the training domain to a different deployment one. To cope with and mitigate this general problem, we present a novel on-device fine-tuning approach that relies only on the limited ultra-low power resources available aboard nano-drones. Then, to overcome the lack of ground-truth training labels aboard our CPS, we also employ a self-supervised method based on ego-motion consistency. Albeit our work builds on top of a specific real-world vision-based human pose estimation task, it is widely applicable for many embedded TinyML use cases. Our 512-image on-device training procedure is fully deployed aboard an ultra-low power GWT GAP9 System-on-Chip and requires only 1MB of memory while consuming as low as 19mW or running in just 510ms (at 38mW). Finally, we demonstrate the benefits of our on-device learning approach by field-testing our closed-loop CPS, showing a reduction in horizontal position error of up to 26 state-of-the-art baseline. In the most challenging never-seen-before environment, our on-device learning procedure makes the difference between succeeding or failing the mission.
更多查看译文
关键词
On-device Learning,Self-supervised Learning,Embedded ML,TinyML,Resource-constrained CPS
AI 理解论文
溯源树
样例
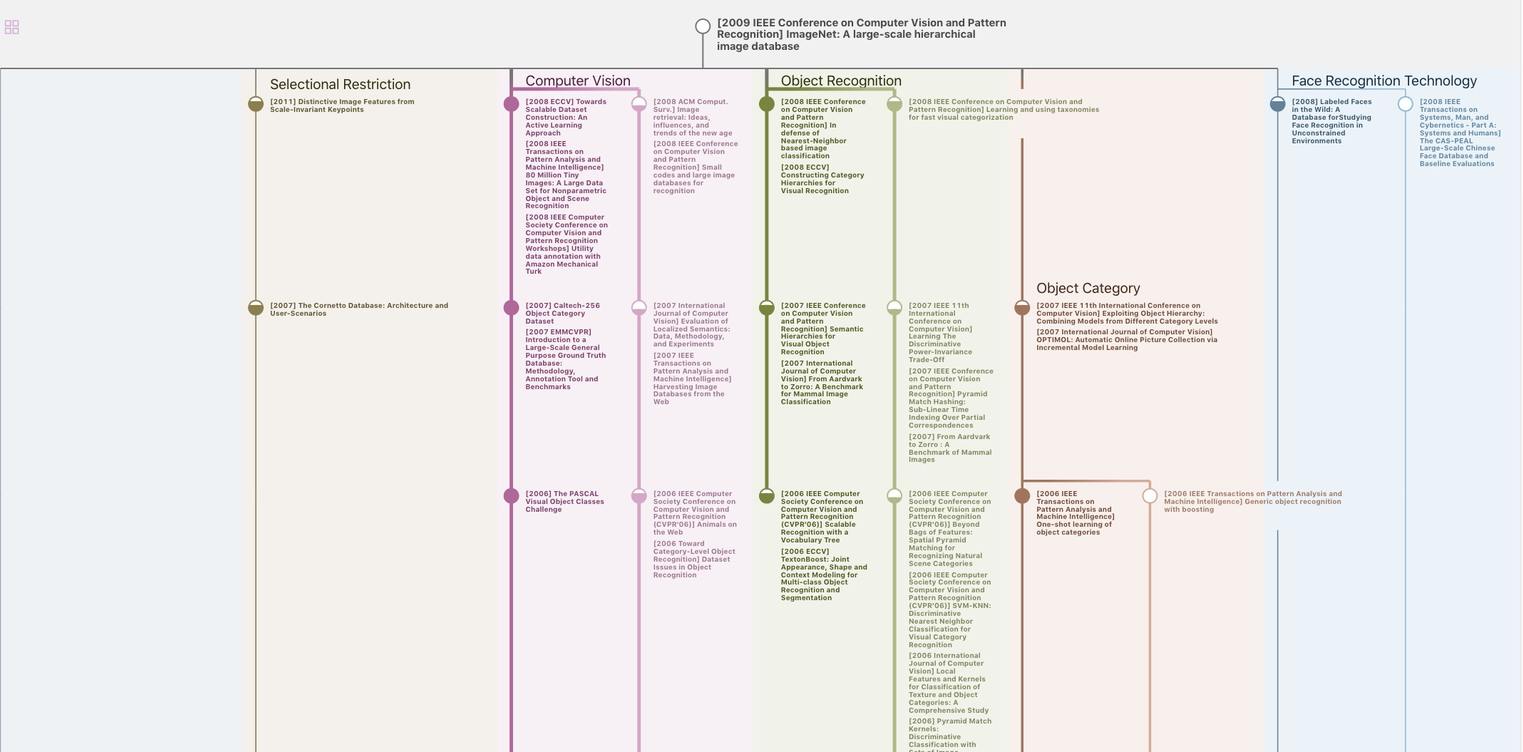
生成溯源树,研究论文发展脉络
Chat Paper
正在生成论文摘要