Online Fair Allocation with Best-of-Many-Worlds Guarantees
arxiv(2024)
摘要
We investigate the online fair allocation problem with sequentially arriving
items under various input models, with the goal of balancing fairness and
efficiency. We propose the unconstrained PACE (Pacing According to Current
Estimated utility) algorithm, a parameter-free allocation dynamic that requires
no prior knowledge of the input while using only integral allocations. PACE
attains near-optimal convergence or approximation guarantees under stationary,
stochastic-but-nonstationary, and adversarial input types, thereby achieving
the first best-of-many-worlds guarantee in online fair allocation. Beyond
theoretical bounds, PACE is highly simple, efficient, and decentralized, and is
thus likely to perform well on a broad range of real-world inputs. Numerical
results support the conclusion that PACE works well under a variety of input
models. We find that PACE performs very well on two real-world datasets even
under the true temporal arrivals in the data, which are highly nonstationary.
更多查看译文
AI 理解论文
溯源树
样例
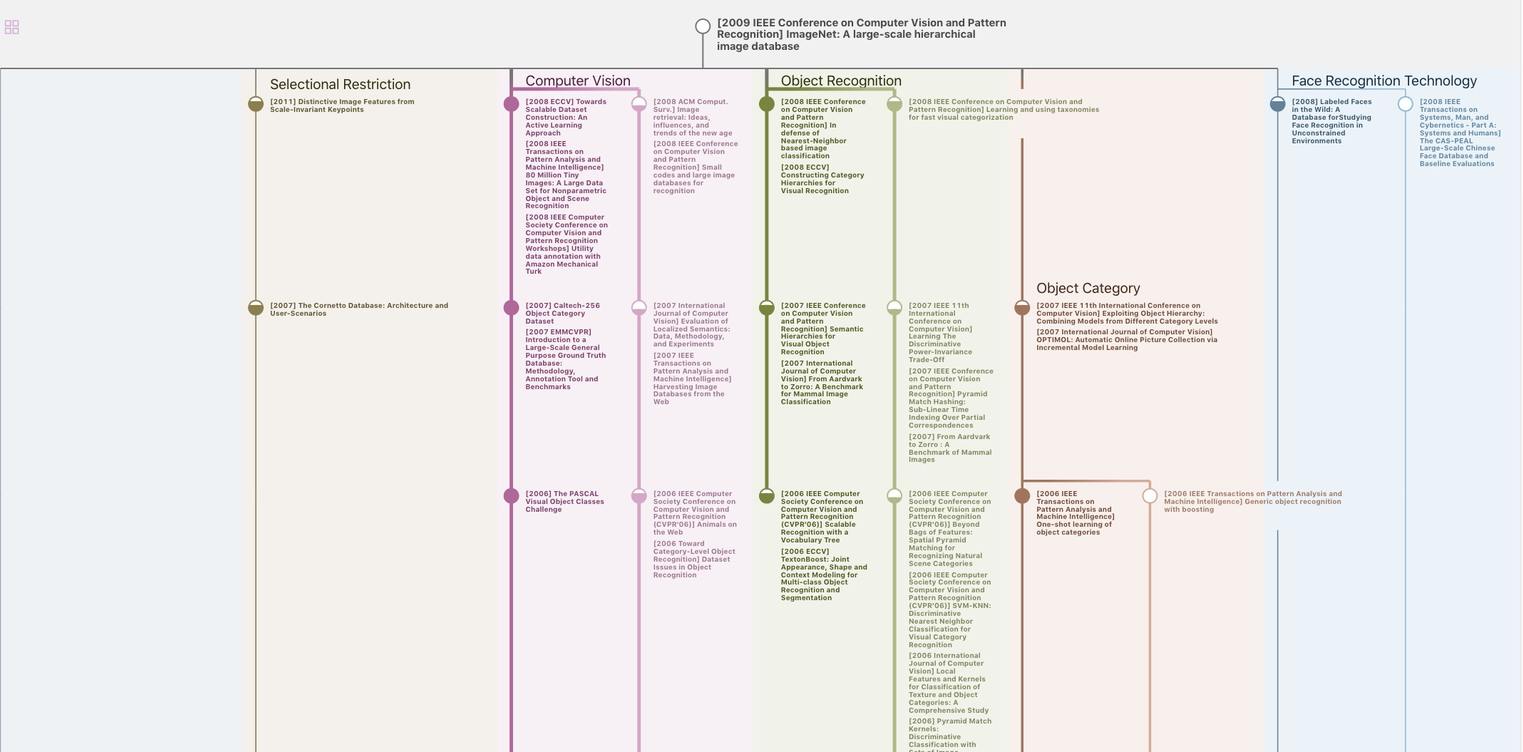
生成溯源树,研究论文发展脉络
Chat Paper
正在生成论文摘要