Unleashing the Power of Data Tsunami: A Comprehensive Survey on Data Assessment and Selection for Instruction Tuning of Language Models
arxiv(2024)
Abstract
Instruction tuning plays a critical role in aligning large language models
(LLMs) with human preference. Despite the vast amount of open instruction
datasets, naively training a LLM on all existing instructions may not be
optimal and practical. To pinpoint the most beneficial datapoints, data
assessment and selection methods have been proposed in the fields of natural
language processing (NLP) and deep learning. However, under the context of
instruction tuning, there still exists a gap in knowledge on what kind of data
evaluation metrics can be employed and how they can be integrated into the
selection mechanism. To bridge this gap, we present a comprehensive review on
existing literature of data assessment and selection especially for instruction
tuning of LLMs. We systematically categorize all applicable methods into
quality-based, diversity-based, and importance-based ones where a unified,
fine-grained taxonomy is structured. For each category, representative methods
are elaborated to describe the landscape of relevant research. In addition,
comparison between latest methods is conducted on their officially reported
results to provide in-depth discussions on their limitations. Finally, we
summarize the open challenges and propose the promosing avenues for future
studies. All related contents are available at
https://github.com/yuleiqin/fantastic-data-engineering.
MoreTranslated text
AI Read Science
Must-Reading Tree
Example
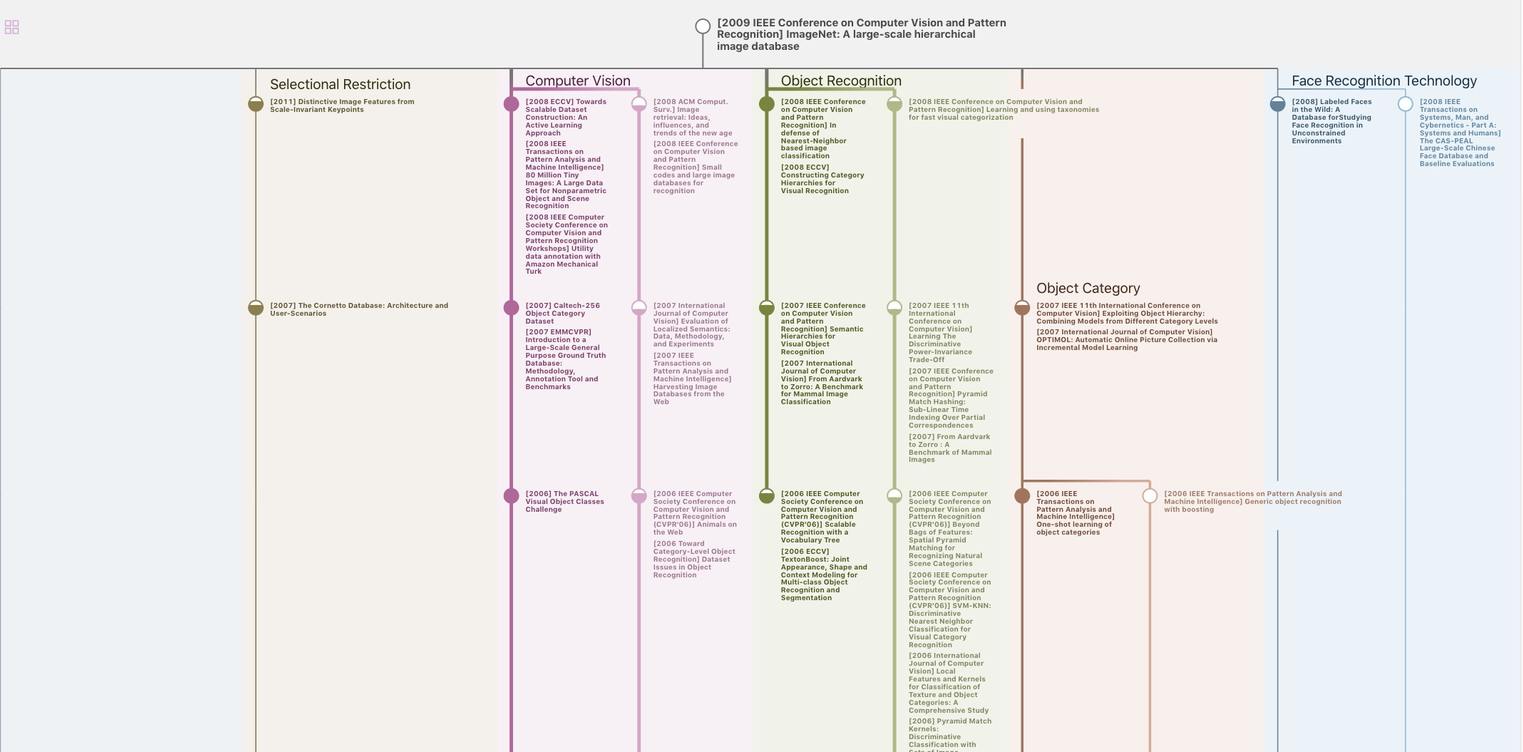
Generate MRT to find the research sequence of this paper
Chat Paper
Summary is being generated by the instructions you defined