Multi-output Machine Learning for Addressing the Trade-off Between Water Permeability and Wetting Resistance in Membrane Distillation
DESALINATION(2024)
摘要
Membrane distillation (MD) has gained extensive attention for the desalination of hypersaline brine. Nevertheless, the performance of MD is often hampered by the inherent trade-off between water permeability and wetting resistance. Elucidating the fundamental basis for this trade-off is crucial for the development of membrane that minimize this limitation. To address this, we trained a multi-output machine learning (ML) model that incorporates various factors, including membrane properties, operational conditions, and solution composition, to predict the performance of MD process. Our model exhibited the best performances for prediction of permeability and wetting resistance, indicted by R2 of 0.901 and 0.873, respectively. The Shapley Additive exPlanations (SHAP) method were employed to interpret the model and extracted significant insights into the contributions of various factors to the trade-off between permeability and wetting resistance, which revealed the surprising importance of the LEP and porosity, as a route for minimizing the trade-off effect. Subsequently, multi-target optimization was performed to address the current permeability-wetting trade-off limitation and the optimal combination of LEP and porosity tailored to different application requirements were provided. This study presents a novel multi-output ML model for predicting the performance of MD process and offers mechanistic insights into the wetting resistance-water permeability trade-off, which represents a paradigm shift for the design of high-performance MD process.
更多查看译文
关键词
Membrane distillation,Machine learning,SHAP value,Trade-off
AI 理解论文
溯源树
样例
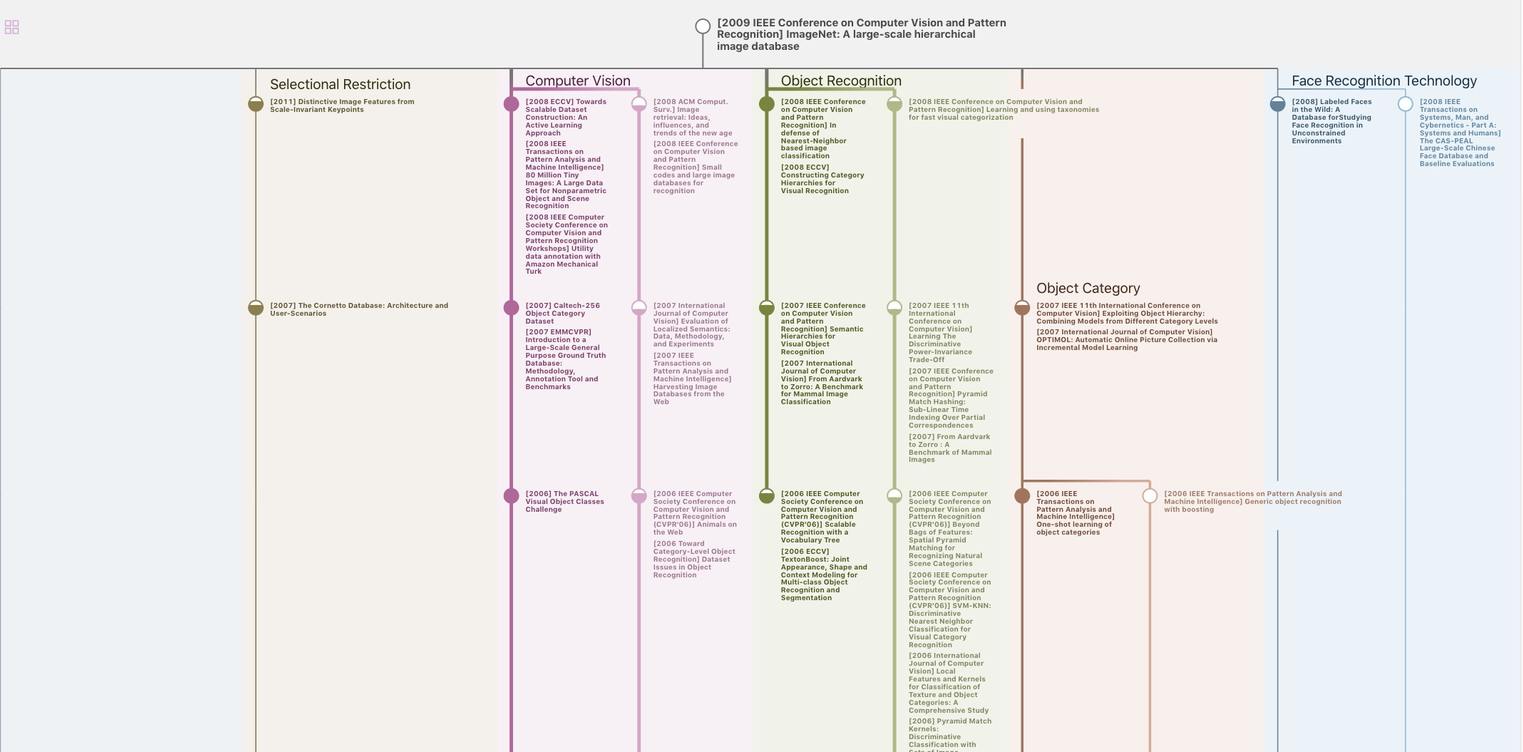
生成溯源树,研究论文发展脉络
Chat Paper
正在生成论文摘要