A New Representation of Universal Successor Features for Enhancing the Generalization of Target-Driven Visual Navigation
IEEE Robotics and Automation Letters(2024)
摘要
Target-driven visual navigation is a long-standing objective in the field of robotics. Deep reinforcement learning methods have demonstrated their effectiveness in developing target-driven visual navigation policies, yet they often struggle with generalization. Although extended reinforcement learning methods based on successor features enhance cross-target generalization, they falter in unseen environments. To tackle this issue, we put forth an approach that builds upon successor features and introduces two novel designs: the parsimonious dynamics and the adaptive policy. Specifically, we employ state features that predict successor features to create latent parsimonious dynamics models. Furthermore, learning a set of rules or dynamics within the latent state facilitates the identification of informative features for prediction and the acquisition of their corresponding successor features. The adaptive policy is to prevent the agent getting trapped by repeatedly taking the same action repeatedly even though it doesn't lead to any progress. Comprehensive results from the interactive AI2-THOR and the Habitat simulator show that agents trained using this method achieve state-of-the-art capabilities in generalization across target, scene, and domain. Additionally, we propose a continuous learning experiment that utilizes pre-trained models to achieve superior generalization.
更多查看译文
关键词
Autonomous agents,target-driven visual navigation,reinforcement learning,generalization
AI 理解论文
溯源树
样例
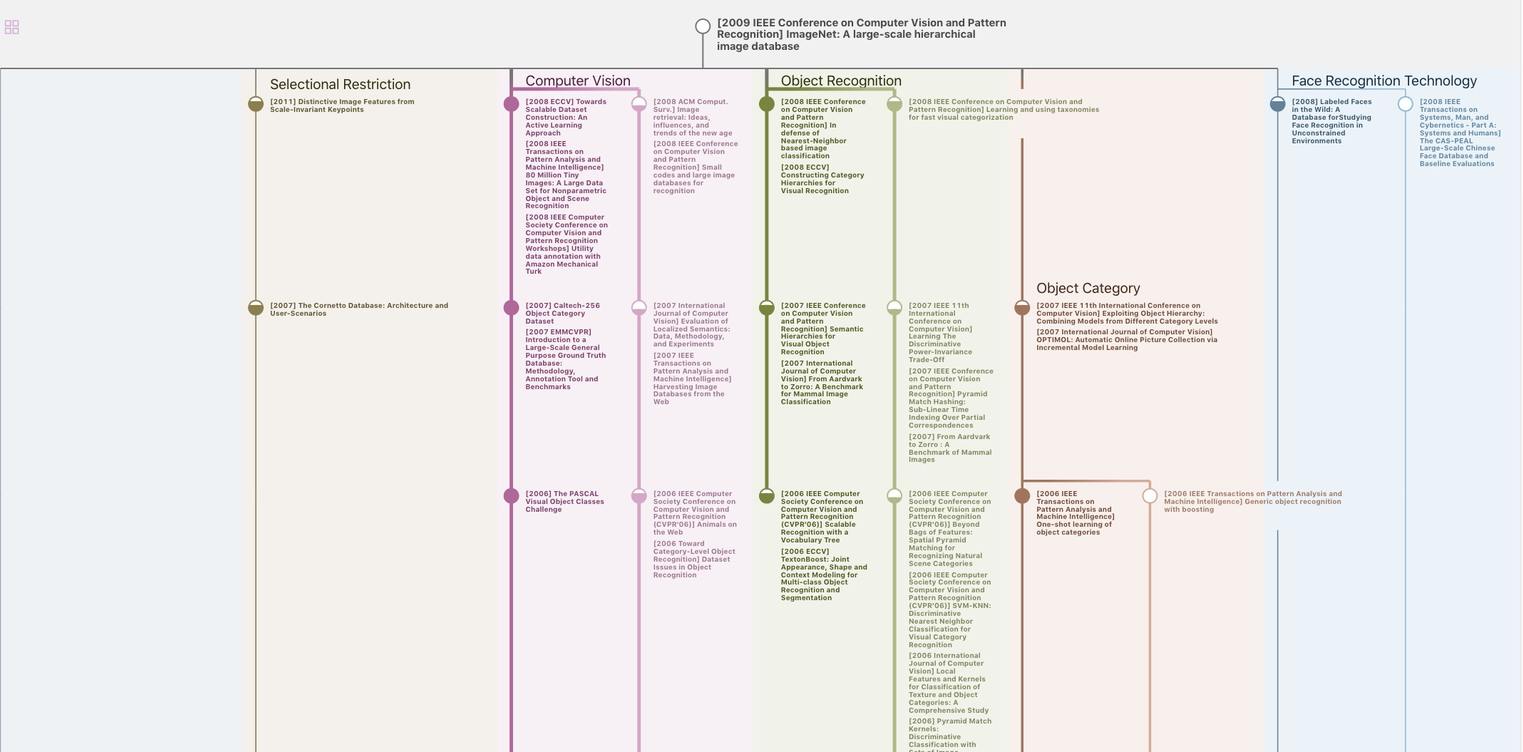
生成溯源树,研究论文发展脉络
Chat Paper
正在生成论文摘要