Deep Learning Architecture with Shunted Transformer and 3D Deformable Convolution for Voxel-Level Dose Prediction of Head and Neck Tumors
Physical and Engineering Sciences in Medicine(2024)
摘要
Intensity-modulated radiation therapy (IMRT) has been widely used in treating head and neck tumors. However, due to the complex anatomical structures in the head and neck region, it is challenging for the plan optimizer to rapidly generate clinically acceptable IMRT treatment plans. A novel deep learning multi-scale Transformer (MST) model was developed in the current study aiming to accelerate the IMRT planning for head and neck tumors while generating more precise prediction of the voxel-level dose distribution. The proposed end-to-end MST model employs the shunted Transformer to capture multi-scale features and learn a global dependency, and utilizes 3D deformable convolution bottleneck blocks to extract shape-aware feature and compensate the loss of spatial information in the patch merging layers. Moreover, data augmentation and self-knowledge distillation are used to further improve the prediction performance of the model. The MST model was trained and evaluated on the OpenKBP Challenge dataset. Its prediction accuracy was compared with three previous dose prediction models: C3D, TrDosePred, and TSNet. The predicted dose distributions of our proposed MST model in the tumor region are closest to the original clinical dose distribution. The MST model achieves the dose score of 2.23 Gy and the DVH score of 1.34 Gy on the test dataset, outperforming the other three models by 8 D_99 , 1.54 D_95 , 1.87 D_1 , 1.87 D_mean , 1.89 D_0.1cc , respectively, superior to the other three models. The quantitative results demonstrated that the proposed MST model achieved more accurate voxel-level dose prediction than the previous models for head and neck tumors. The MST model has a great potential to be applied to other disease sites to further improve the quality and efficiency of radiotherapy planning.
更多查看译文
关键词
Deep learning,Dose prediction,Head and neck tumors,Multi-scale transformer model
AI 理解论文
溯源树
样例
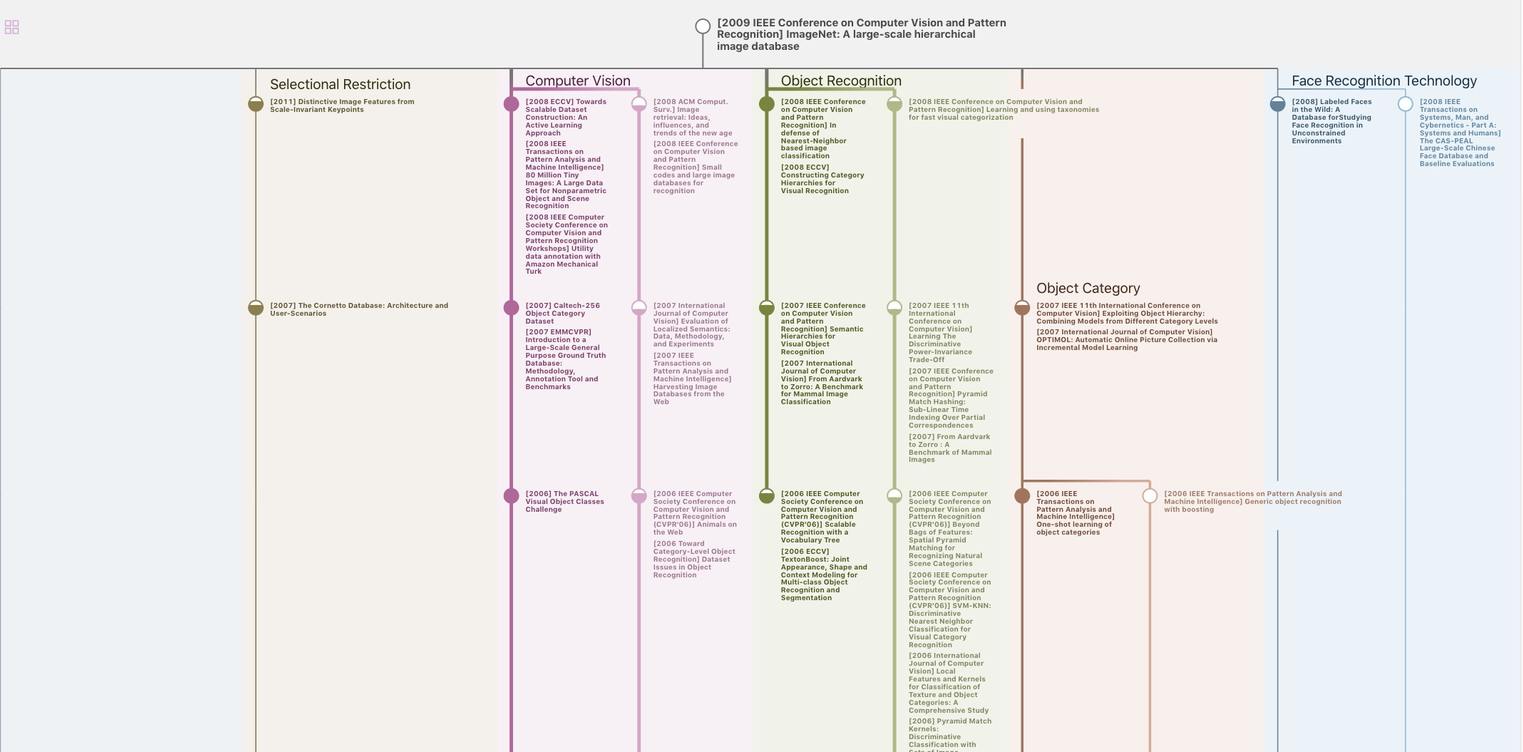
生成溯源树,研究论文发展脉络
Chat Paper
正在生成论文摘要