DSAI-09 APPLYING CONSENSUS QUALITY OF CARE MEASURES FOR BRAIN METASTASES (BMETS-QC) TO PATIENT EHR DATA, A PILOT STUDY
Neuro-Oncology Advances(2024)
UVA Cancer Center | UVA Health
Abstract
Optimal management of patients with brain metastases requires coordinated, interprofessional care, that is well-documented in their electronic health record (EHR). There are unquantified inconsistencies and gaps in the care for this complex medical diagnosis. We explored the feasibility of applying a peer-reviewed set of 29 quality of care (QOC) consensus measures to commercially available aggregated, limited EHR data. These measures address care planning, treatment timing, assessment of QoL, referrals to palliative care and hospice, and survival. Patients with BMETS were selected (N=43) from a an EHR data warehouse sourced from IQVIA’s collaboration with the Guardian Research Network (GRN). Data extraction followed curation rules developed by the study team and performed by GRN. Patients’ EHR data was manually reviewed by trained staff. Both structured data and unstructured text notes were reviewed and coded. Dates of diagnosis, medical services, and death were masked by indexing to birth date. For each indicator, a patient’s EHR data was coded as done (+), not done(-), or inconclusive (missing). Of 29 indicators, 23 could be ascertained in > 85% of cases. Instances of lower ascertainment occurred for patients lost to follow-up, missing dates of service, no information on planned or actual dose, or missing prognostic scores. BMETS-QC processes ascertained as not performed (-) mostly involved a lack of documented patient-centered care (i.e., shared decision-making, patient education, and psychosocial support). Finally, 12 indicators required the use of unstructured data and required data curation with manual review or NLP. Most BMETS-QC indicators appear feasible to collect in EHR data with curation processes. An important finding is that providers may fail to document key elements of patient-centered care or timely prognostic information. To handle missing data, patients must have their BMETS care recorded within a single EHR system.
MoreTranslated text
求助PDF
上传PDF
View via Publisher
AI Read Science
AI Summary
AI Summary is the key point extracted automatically understanding the full text of the paper, including the background, methods, results, conclusions, icons and other key content, so that you can get the outline of the paper at a glance.
Example
Background
Key content
Introduction
Methods
Results
Related work
Fund
Key content
- Pretraining has recently greatly promoted the development of natural language processing (NLP)
- We show that M6 outperforms the baselines in multimodal downstream tasks, and the large M6 with 10 parameters can reach a better performance
- We propose a method called M6 that is able to process information of multiple modalities and perform both single-modal and cross-modal understanding and generation
- The model is scaled to large model with 10 billion parameters with sophisticated deployment, and the 10 -parameter M6-large is the largest pretrained model in Chinese
- Experimental results show that our proposed M6 outperforms the baseline in a number of downstream tasks concerning both single modality and multiple modalities We will continue the pretraining of extremely large models by increasing data to explore the limit of its performance
Upload PDF to Generate Summary
Must-Reading Tree
Example
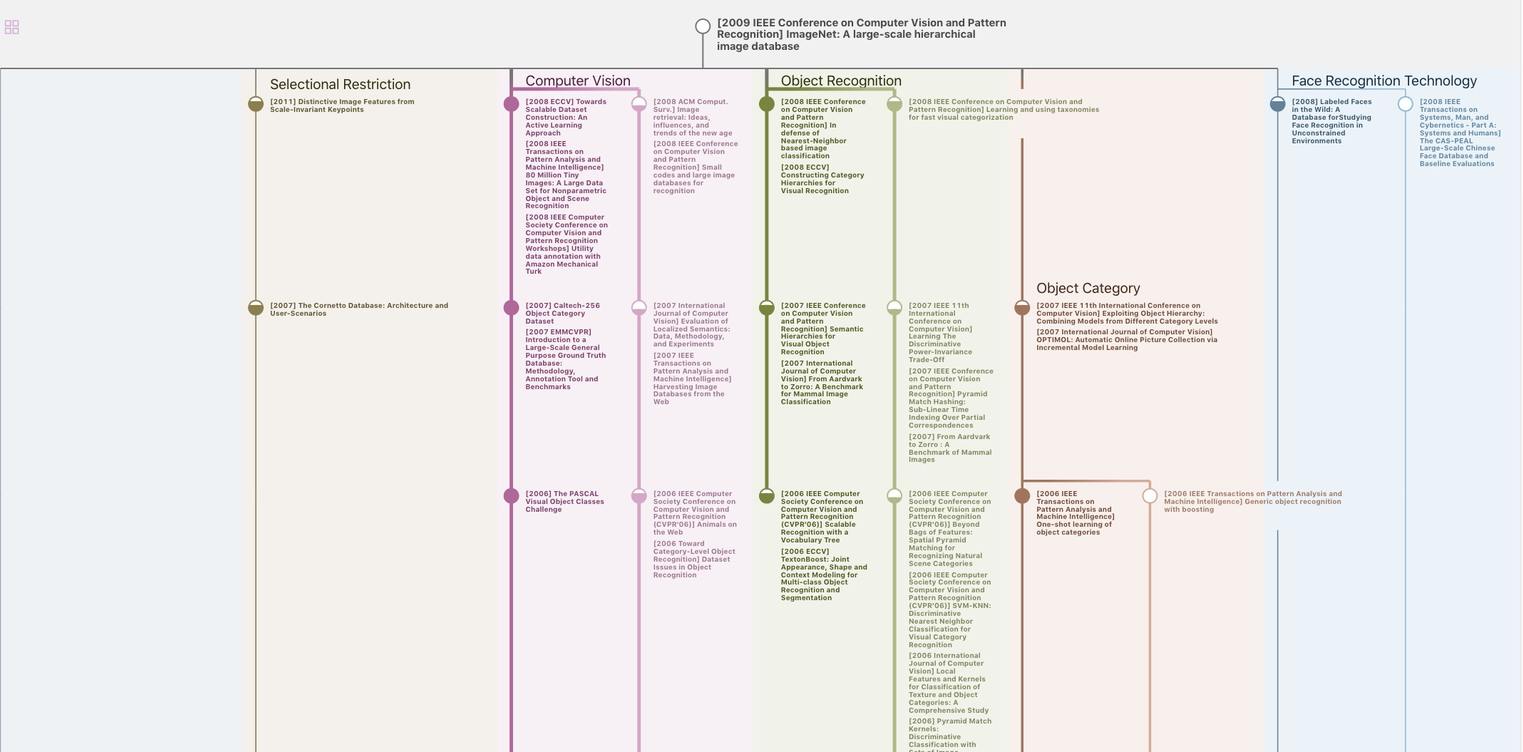
Generate MRT to find the research sequence of this paper
Data Disclaimer
The page data are from open Internet sources, cooperative publishers and automatic analysis results through AI technology. We do not make any commitments and guarantees for the validity, accuracy, correctness, reliability, completeness and timeliness of the page data. If you have any questions, please contact us by email: report@aminer.cn
Chat Paper
GPU is busy, summary generation fails
Rerequest