Capturing Exposure Disparities with Chemical Transport Models: Evaluating the Suitability of Downscaling Using Land Use Regression
ENVIRONMENTAL SCIENCE & TECHNOLOGY(2024)
摘要
High resolution exposure surfaces are essential to capture disparities in exposure to traffic-related air pollution in urban areas. In this study, we develop an approach to downscale Chemical Transport Model (CTM) simulations to a hyperlocal level (similar to 100m) in the Greater Toronto Area (GTA) under three scenarios where emissions from cars, trucks and buses are zeroed out, thus capturing the burden of each transportation mode. This proposed approach statistically fuses CTMs with Land-Use Regression using machine learning techniques. With this proposed downscaling approach, changes in air pollutant concentrations under different scenarios are appropriately captured by downscaling factors that are trained to reflect the spatial distribution of emission reductions. Our validation analysis shows that high-resolution models resulted in better performance than coarse models when compared with observations at reference stations. We used this downscaling approach to assess disparities in exposure to nitrogen dioxide (NO2) for populations composed of renters, low-income households, recent immigrants, and visible minorities. Individuals in all four categories were disproportionately exposed to the burden of cars, trucks, and buses. We conducted this analysis at spatial resolutions of 12, 4, 1 km, and 100 m and observed that disparities were significantly underestimated when using coarse spatial resolutions. This reinforces the need for high-spatial resolution exposure surfaces for environmental justice analyses.
更多查看译文
关键词
air pollution,environmental justice,machinelearning,traffic emissions,nitrogen dioxide,land use regression,chemical transport models,downscaling
AI 理解论文
溯源树
样例
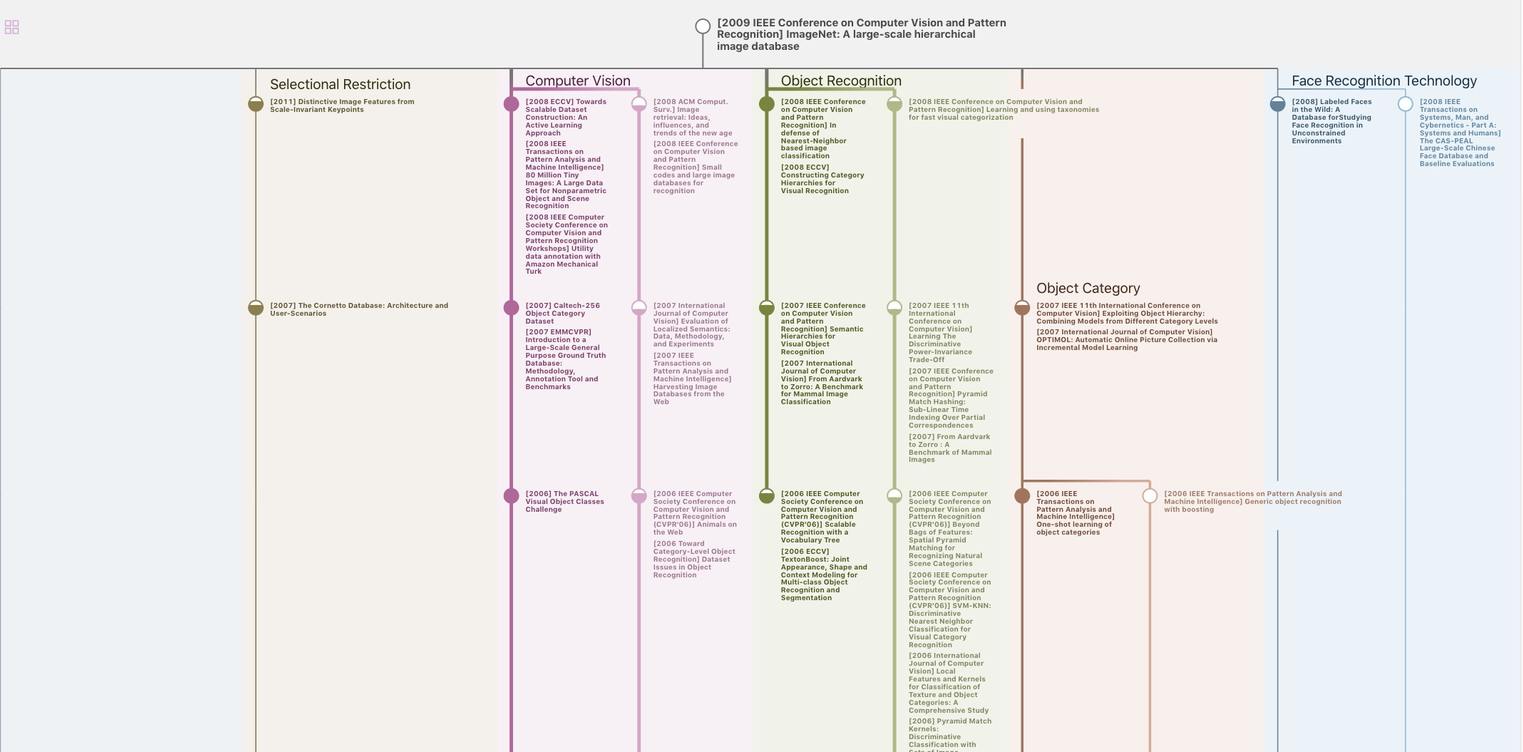
生成溯源树,研究论文发展脉络
Chat Paper
正在生成论文摘要