The accuracy and quality of image-based artificial intelligence for muscle-invasive bladder cancer prediction
Insights into Imaging(2024)
摘要
To evaluate the diagnostic performance of image-based artificial intelligence (AI) studies in predicting muscle-invasive bladder cancer (MIBC). (2) To assess the reporting quality and methodological quality of these studies by Checklist for Artificial Intelligence in Medical Imaging (CLAIM), Radiomics Quality Score (RQS), and Prediction model Risk of Bias Assessment Tool (PROBAST). We searched Medline, Embase, Web of Science, and The Cochrane Library databases up to October 30, 2023. The eligible studies were evaluated using CLAIM, RQS, and PROBAST. Pooled sensitivity, specificity, and the diagnostic performances of these models for MIBC were also calculated. Twenty-one studies containing 4256 patients were included, of which 17 studies were employed for the quantitative statistical analysis. The CLAIM study adherence rate ranged from 52.5
更多查看译文
关键词
Magnetic resonance imaging,Urinary bladder neoplasms,Neoplasm staging,Muscle-invasive bladder neoplasms,Artificial intelligence
AI 理解论文
溯源树
样例
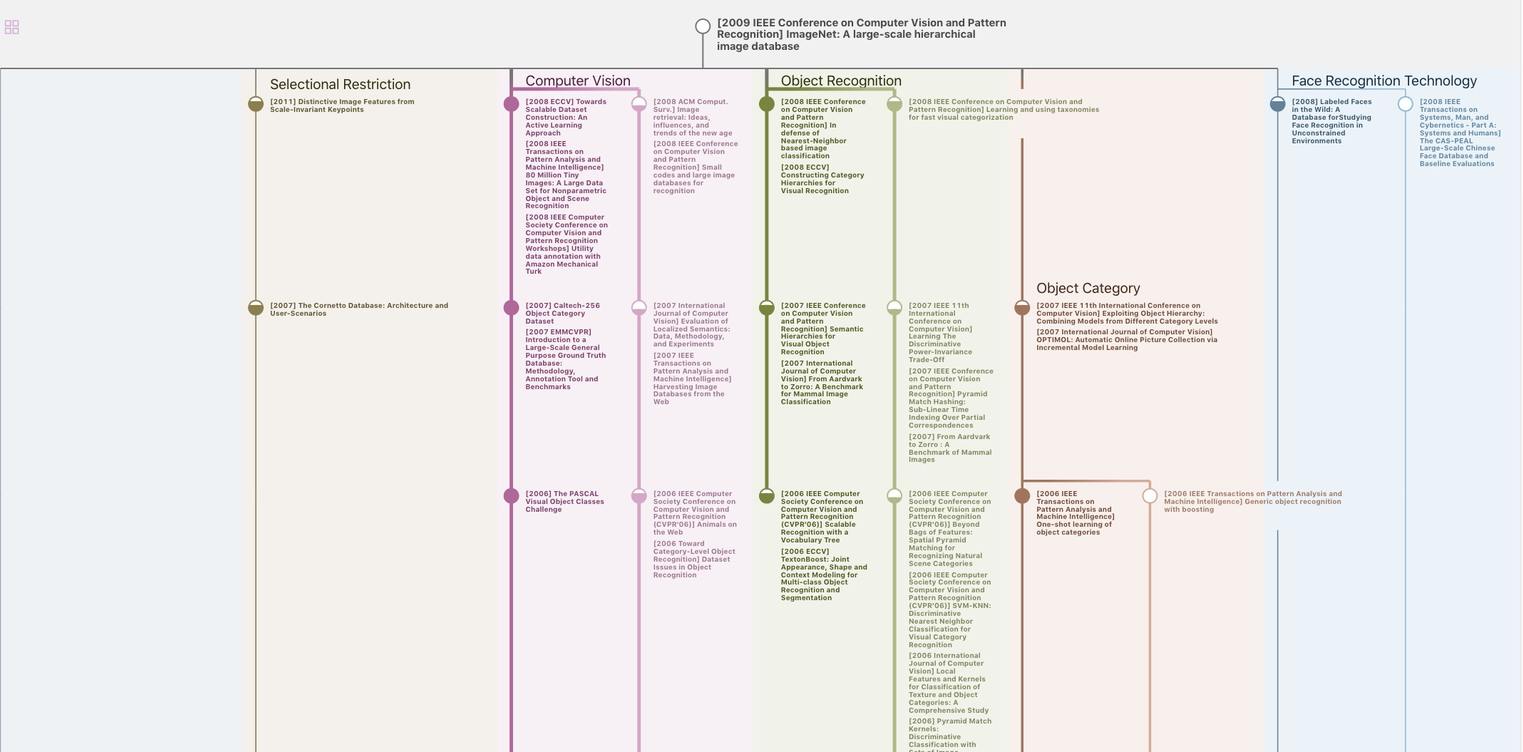
生成溯源树,研究论文发展脉络
Chat Paper
正在生成论文摘要