Genomic Selection Optimization in Blueberry: Data-driven Methods for Marker and Training Population Design.
PLANT GENOME(2024)
摘要
Genomic prediction is a modern approach that uses genome-wide markers to predict the genetic merit of unphenotyped individuals. With the potential to reduce the breeding cycles and increase the selection accuracy, this tool has been designed to rank genotypes and maximize genetic gains. Despite this importance, its practical implementation in breeding programs requires critical allocation of resources for its application in a predictive framework. In this study, we integrated genetic and data-driven methods to allocate resources for phenotyping and genotyping tailored to genomic prediction. To this end, we used a historical blueberry (Vaccinium corymbosun L.) breeding dataset containing more than 3000 individuals, genotyped using probe-based target sequencing and phenotyped for three fruit quality traits over several years. Our contribution in this study is threefold: (i) for the genotyping resource allocation, the use of genetic data-driven methods to select an optimal set of markers slightly improved prediction results for all the traits; (ii) for the long-term implication, we carried out a simulation study and emphasized that data-driven method results in a slight improvement in genetic gain over 30 cycles than random marker sampling; and (iii) for the phenotyping resource allocation, we compared different optimization algorithms to select training population, showing that it can be leveraged to increase predictive performances. Altogether, we provided a data-oriented decision-making approach for breeders by demonstrating that critical breeding decisions associated with resource allocation for genomic prediction can be tackled through a combination of statistics and genetic methods. Optimization provides realistic approach for marker density reduction without trade-off in predictive ability. Simulation revealed superior long-term genetic gain with the application of data-driven method. Training breeding population can be optimized using data driven methods for genomic selection. Implementation of genomic selection in a practical breeding program relies on evaluating multiple traits at the field level and obtaining DNA information in large populations. Costly and time-consuming, both actions can be optimized using statistical and genetic parameters. In this paper, we suggest some parameters that could be investigated to guide the selection of molecular markers and orient the identification of key individuals to be included in genomic prediction. Our results apply to blueberry demonstrated that genomic selection can be optimized, and the use of data-driven methods has the potential to reduce cost and time in the long term.
更多查看译文
AI 理解论文
溯源树
样例
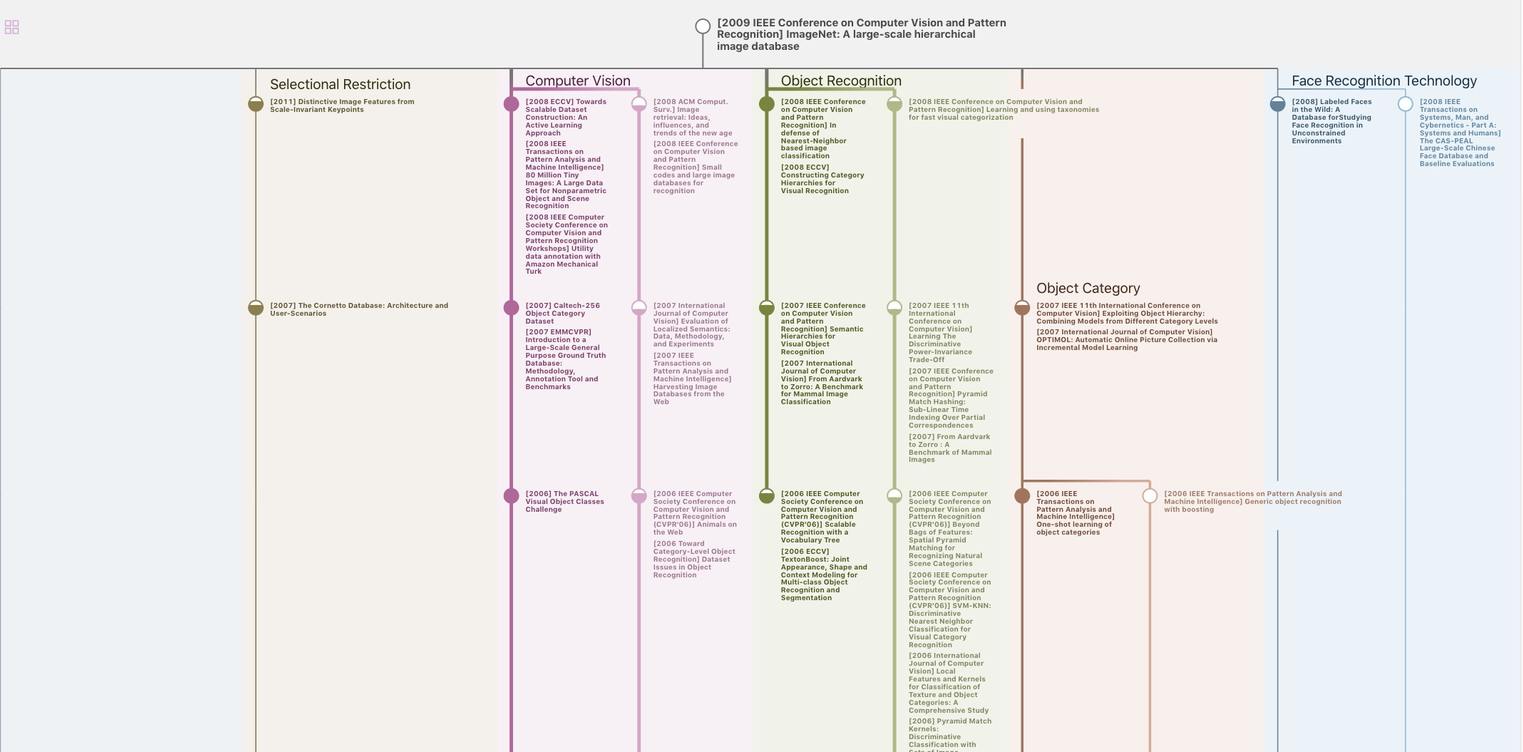
生成溯源树,研究论文发展脉络
Chat Paper
正在生成论文摘要