Predictive models for secondary epilepsy within 1 year in patients with acute ischemic stroke: a multicenter retrospective study
medrxiv(2024)
摘要
Objective:Post-stroke epilepsy (PSE) is a significant complication that has a negative impact on the prognosis and quality of life of ischemic stroke patients. We collected medical records from 4 hospitals in Chongqing and created an interpretable machine learning model for prediction.Methods: We collected medical records, imaging reports, and laboratory tests from 21459 patients with a diagnosis of ischemic stroke . We conducted traditional univariable and multivariable statistics analyses to compare and identify important features. Then the data was divided into a 70% training set and a 30% testing set. We employed the Synthetic Minority Oversampling Technique combined with Edited Nearest Neighbors method to resample an imbalanced dataset in the training set. Nine commonly used methods were used to build machine learning models, and relevant prediction metrics were compared to select the best-performing model. Finally, we used SHAP(SHapley Additive exPlanations) for model interpretability analysis, assessing the contribution and clinical significance of different features to the prediction.Results: In the traditional regression analysis, complications such as hydrocephalus, cerebral hernia, uremia, deep vein thrombosis; significant brain regions included the involvement of the cortical regions including frontal lobe, parietal lobe, occipital lobe, temporal lobe, subcortical region of basal ganglia, thalamus and so on contributed to PSE. General features such as age, gender, and the National Institutes of Health Stroke Scale score, as well as laboratory indicators including WBC count, D-dimer, lactate, HbA1c and so on were associated with a higher likelihood of PSE. Patients with conditions such as fatty liver, coronary heart disease, hyperlipidemia, and low HDL had a higher likelihood of developing PSE. The machine learning models, particularly tree models such as Random Forest, XGBoost, and LightGBM, demonstrated good predictive performance with an AUC of 0.99.Conclusion:The model built on a large dataset can effectively predict the likelihood of PSE, with tree-based models performing the best. The NIHSS score , WBC count and d-dimer were found to have the greatest impact.
### Competing Interest Statement
The authors have declared no competing interest.
### Funding Statement
The research is funded by Based on artificial intelligence and multiple omics technology set up a system of auxiliary cardiovascular disease diagnosis and treatment(2023CDJYGRH-ZD06);by Emergency medicine Key laboratory of Chongqing Joint Fund for Talent Innovation and development(2024RCCX10),by Brain-like intelligence research Key laboratory of chongqing Education Commission(BIR2019004)
### Author Declarations
I confirm all relevant ethical guidelines have been followed, and any necessary IRB and/or ethics committee approvals have been obtained.
Yes
The details of the IRB/oversight body that provided approval or exemption for the research described are given below:
The study protocol was approved by the Ethics Committees of Chongqing University Center Hospital, Chongqing University Qianjiang Central Hospital, Bishan District People's Hospital, and Yubei Traditional Chinese Medicine Hospital.
I confirm that all necessary patient/participant consent has been obtained and the appropriate institutional forms have been archived, and that any patient/participant/sample identifiers included were not known to anyone (e.g., hospital staff, patients or participants themselves) outside the research group so cannot be used to identify individuals.
Yes
I understand that all clinical trials and any other prospective interventional studies must be registered with an ICMJE-approved registry, such as ClinicalTrials.gov. I confirm that any such study reported in the manuscript has been registered and the trial registration ID is provided (note: if posting a prospective study registered retrospectively, please provide a statement in the trial ID field explaining why the study was not registered in advance).
Yes
I have followed all appropriate research reporting guidelines, such as any relevant EQUATOR Network research reporting checklist(s) and other pertinent material, if applicable.
Yes
The codes,models,analysis results was uploaded at https://github.com/conanan/lasso-ml . The full dataset can be provided for researchers if needed by the corresponding author.
更多查看译文
AI 理解论文
溯源树
样例
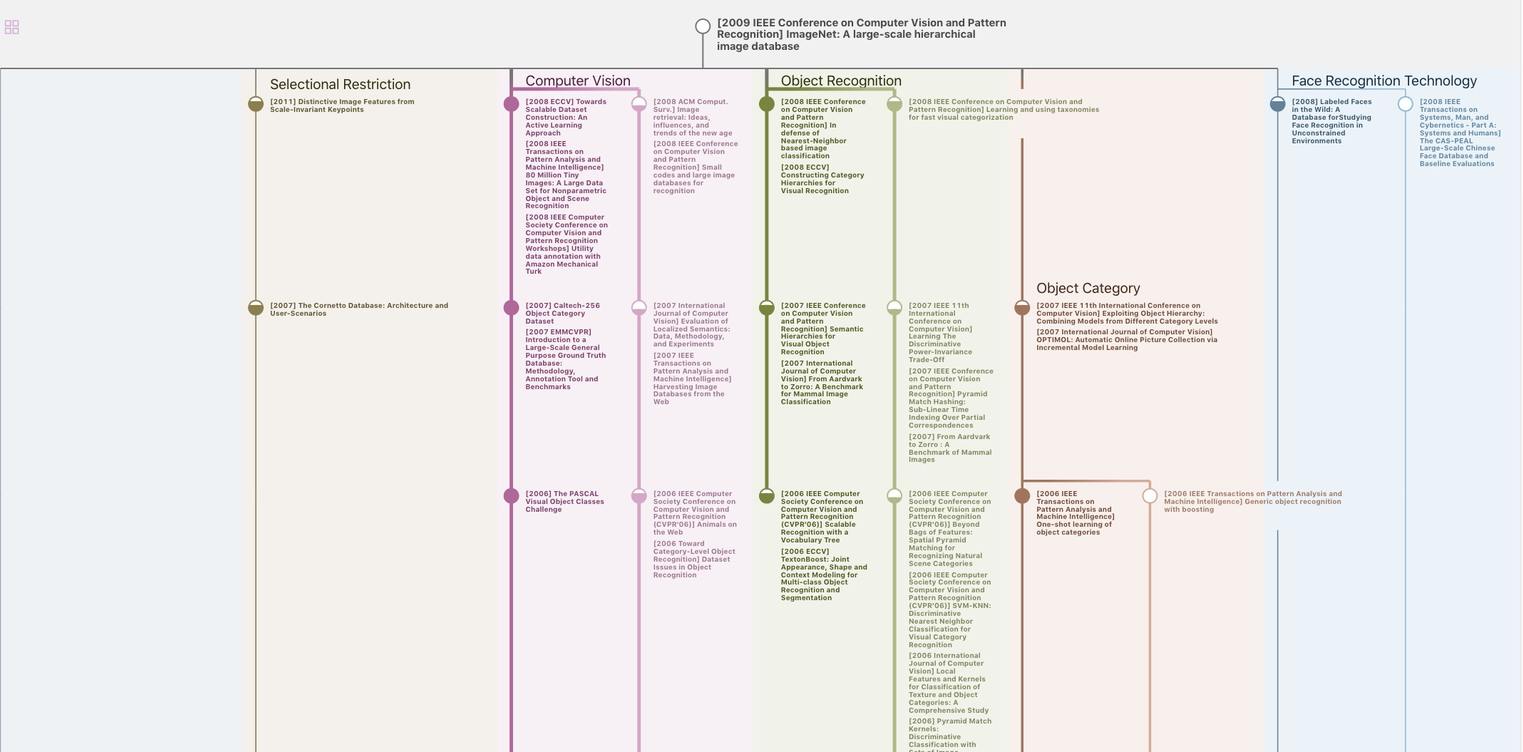
生成溯源树,研究论文发展脉络
Chat Paper
正在生成论文摘要