Harnessing genome prediction in Brassica napus through a nested association mapping population
biorxiv(2024)
摘要
Genome prediction (GP) significantly enhances genetic gain by improving selection efficiency and shortening crop breeding cycles. Using a nested association mapping (NAM) population a set of diverse scenarios were assessed to evaluate GP for vital agronomic traits in B. napus. GP accuracy was examined by employing different models, marker sets, population sizes, marker densities, and incorporating genome-wide association (GWAS) markers. Eight models, including linear and semi-parametric approaches, were tested. The choice of model minimally impacted GP accuracy across traits. Notably, two models, rrBLUP and RKHS, consistently yielded the highest prediction accuracies. Employing a training population of 1500 lines or more resulted in increased prediction accuracies. Inclusion of single nucleotide absence polymorphism (SNaP) markers significantly improved prediction accuracy, with gains of up to 15%. Utilizing the Brassica 60K Illumina SNP array, our study effectively revealed the genetic potential of the B. napus NAM panel. It provided estimates of genomic predictions for crucial agronomic traits through varied prediction scenarios, shedding light on achievable genetic gains. These insights, coupled with marker application, can advance the breeding cycle acceleration in B. napus.
### Competing Interest Statement
The authors have declared no competing interest.
更多查看译文
AI 理解论文
溯源树
样例
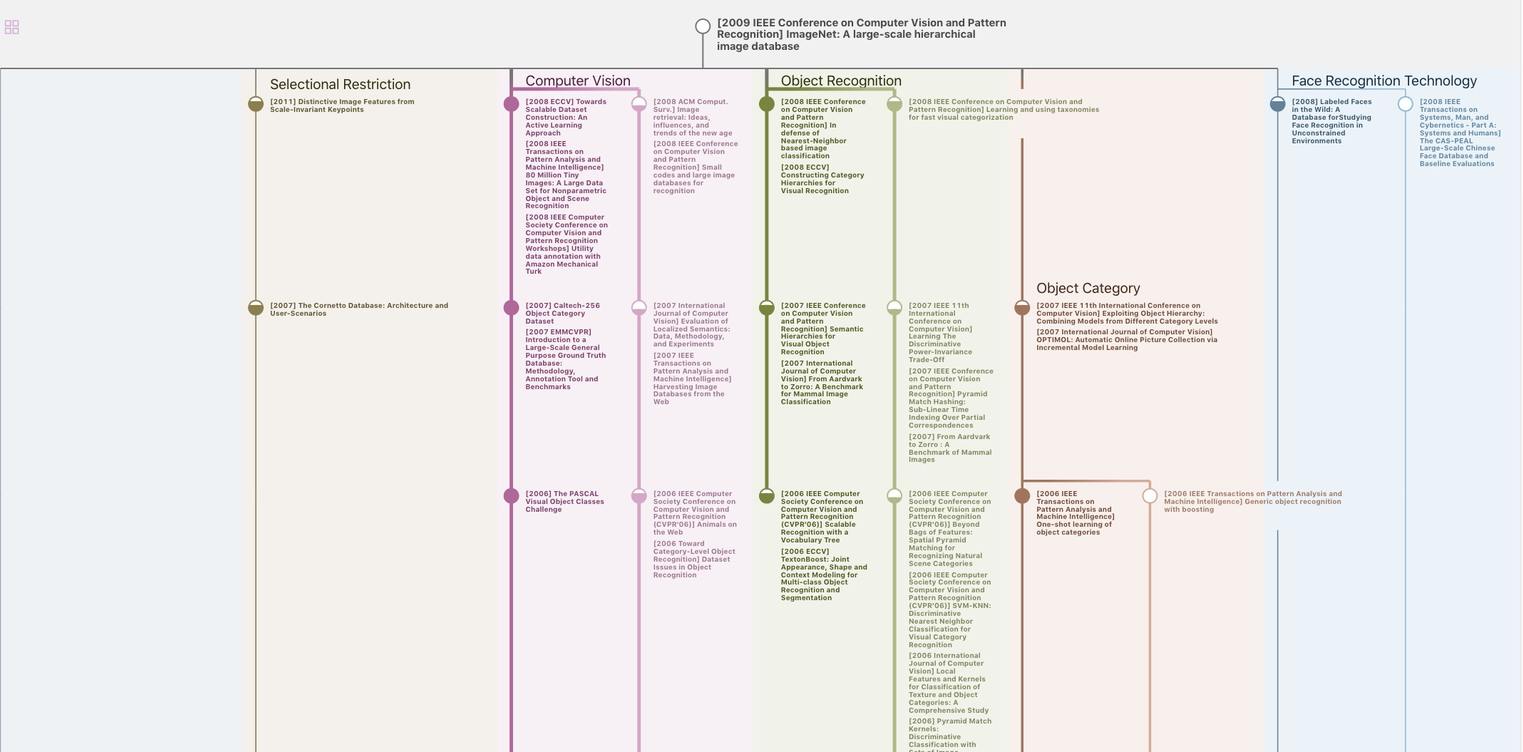
生成溯源树,研究论文发展脉络
Chat Paper
正在生成论文摘要