Machine Learning-Aided Design of LaCe(Fe,Mn,Si)13H-type Magnetocaloric Materials for Room-Temperature Applications
JOURNAL OF ALLOYS AND COMPOUNDS(2024)
摘要
La(Fe,Si)13 magnetocaloric materials have attracted extensive attention in recent years due to their excellent magnetocaloric properties and low price. In this study, we established and compared several machine models and used a support vector machine for predicting the influences of different element contents on the Curie temperature. Based on the results predicted by the machine learning model, a series of fully hydrogenated materials (La1.1-xCexFe13-y-zMnySizHF, y approximate to 0.3-0.5x, z=1.1, 1.3, 1.5) with Curie temperatures between 290 and 310 K were designed. After material screening, a material with an element composition of La0.66Ce0.44Fe11.4Mn0.1Si1.5HF was proven to have good room temperature magnetocaloric properties, with a Curie temperature of 301 K, magnetic entropy change of 11.3 J/kgK under a 0-2 T magnetic field, and a relative cooling power of 119.3 J/kg, which performs well in the existing La(Fe,Si)13-type materials that can be applied near room temperature. This work not only deepens researchers' understanding of machine learning-aided design of materials with specific application environment restrictions but also greatly accelerates the design of near-room temperature La(Fe,Si)13 magnetocaloric materials.
更多查看译文
关键词
Machine learning,Magnetocaloric effect,La-Fe-Si alloy,Microstructure and phase transition
AI 理解论文
溯源树
样例
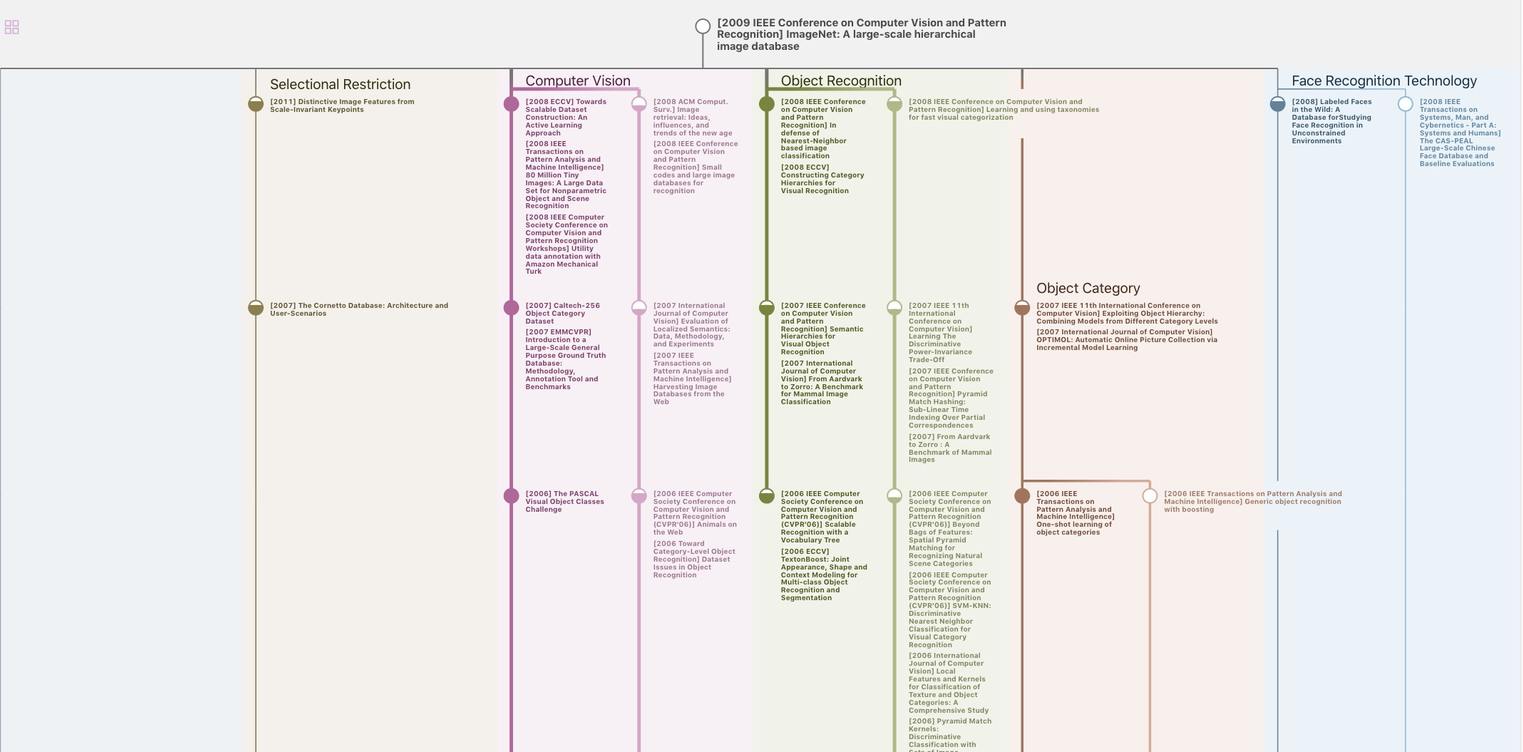
生成溯源树,研究论文发展脉络
Chat Paper
正在生成论文摘要