A Hybrid CNN-LSTM Network with Attention Mechanism for Myoelectric Control in Upper Limb Exoskeletons.
International Conference on Ubiquitous Robots and Ambient Intelligence(2024)
摘要
This paper introduces a novel attention-based sequence-to-sequence network for predicting upper-limb ex-oskeleton joint angles, enhancing the control of assistive tech-nologies for individuals with upper limb impairments. By integrating EMG and IMU signals, our model facilitates real-time decoding of user intentions, generating precise movement trajectories for a 3-DoF cable-driven upper-limb exoskeleton. The implementation of an attention mechanism within an encoder-decoder architecture allows for the dynamic prioritization of the most pertinent EMG features and historical angular positions. Our model significantly outperforms existing EMG-based hand motion prediction methods in terms of prediction accuracy and responsiveness as demonstrated in evaluations. This approach not only offers a tailored response to varying sequence lengths and compensates for sensor unreliability but also introduces a level of generalization and adaptability previously unattainable in robotic rehabilitation and assistive devices. The implementation of the attention mechanism allows for adaptive learning, focusing on the most relevant signals for each user, thereby enhancing the system's ability to learn and predict complex movement trajectories.
更多查看译文
关键词
Upper Limb,Attention Mechanism,Upper Limb Exoskeleton,Hybrid CNN-LSTM,Hybrid CNN-LSTM Network,Prediction Accuracy,Sequence Length,Assistive Technology,Joint Angles,Inertial Measurement Unit,Angular Position,EMG Signals,Rehabilitation Robots,Upper Limb Impairment,Neural Network,Model Performance,Convolutional Neural Network,Feature Maps,Ability Of The Model,Spinal Cord Injury,Exosuit,EMG Data,Inertial Measurement Unit Data,Longer Sequences,Capability Of Model,Gravity Compensation,sEMG Signals,Joint Position,Hidden State,Context Vector
AI 理解论文
溯源树
样例
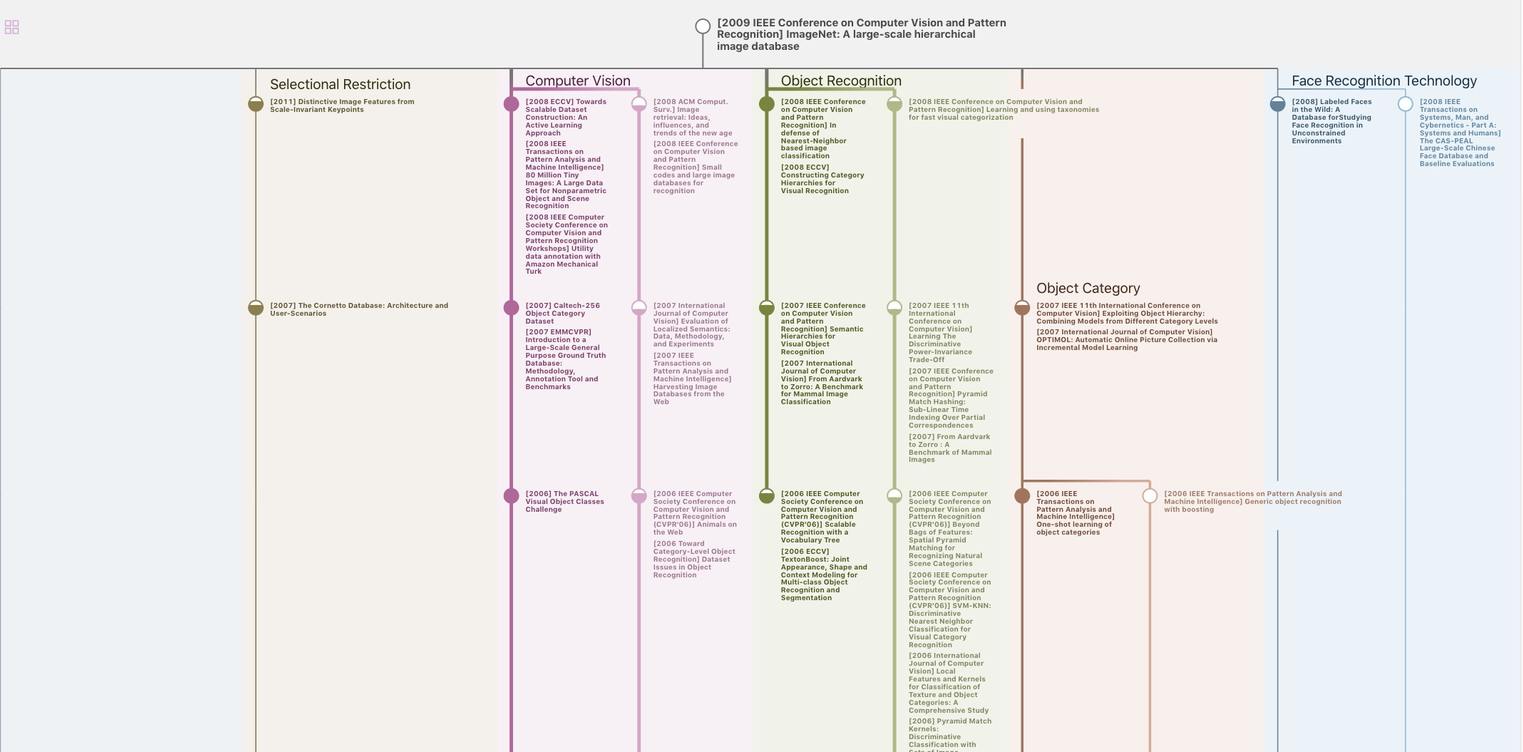
生成溯源树,研究论文发展脉络
Chat Paper
正在生成论文摘要