An Improved Imitation Learning-based Online Scheduling Strategy for Microgrids with Renewable Energy and Energy Storage
2024 IEEE 2nd International Conference on Power Science and Technology (ICPST)(2024)
摘要
The development of data-driven techniques, particularly imitation learning (IL), has provided new avenues for online optimization of microgrids. However, the sequential decision-making nature of microgrid online optimization, along with clear spatiotemporal coupling characteristics, results in the accumulation of errors during the optimization process, affecting the optimality and accuracy of scheduling results. This paper proposes a novel strategy that combines expert decision classification and the bidirectional long-short term memory (Bi-LSTM) algorithm to improve the online scheduling results of microgrids. Specifically, during the off-line training phase, the expert decision data are classified based on the energy storage scheduling state, followed by the utilization of Bi-LSTM algorithm to train more refined models for each expert decision category. In the online decision-making process, a category filtering model is first used to identify the expert decision category to which a given scenario belongs, and the corresponding model is then adopted to provide more accurate scheduling results for the given scenario. That is, this paper innovatively introduces classification into the learning process of scheduling strategy to reduce single-step errors and cascade cumulative errors. The simulation results show that compared with pure IL method, mean-square error (MSE) and mean absolute error (MAE) of our approach are reduced by 9.13 and 1.29 respectively and R-Squared (R2) is increased to 0.96, which effectively reduces cascading errors and enhances the accuracy of online scheduling.
更多查看译文
关键词
online scheduling,microgrid,cascading errors,classification,Bi-LSTM
AI 理解论文
溯源树
样例
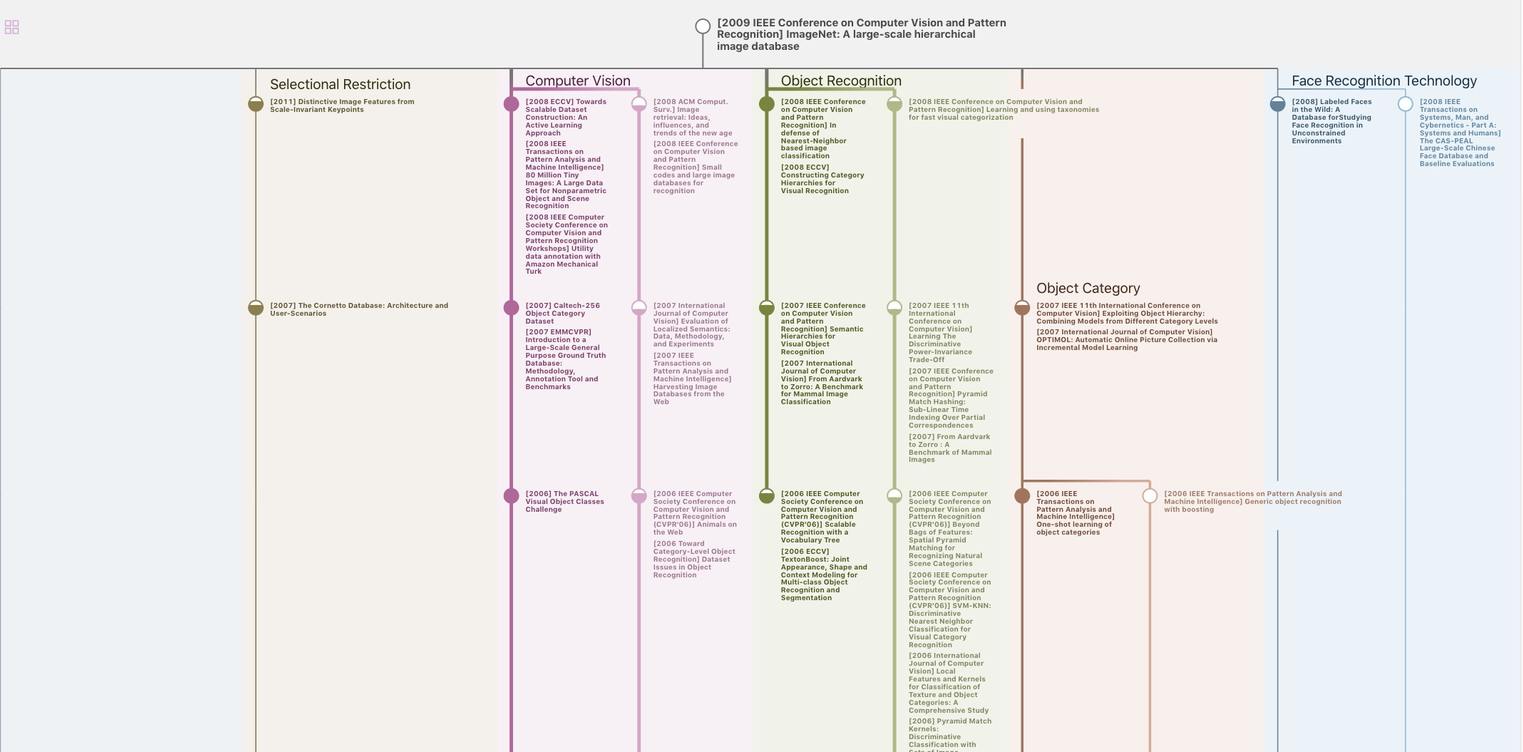
生成溯源树,研究论文发展脉络
Chat Paper
正在生成论文摘要