Similarity measurement of symbolic sequence based on complexity estimate and dynamic time warping
Nonlinear Dynamics(2024)
摘要
Symbolic Aggregate approXimation (SAX) represents a classic approach for transforming time series data into symbolic representations, achieving dimensionality reduction and providing a distance measurement method between symbolic sequences. However, classic SAX technique primarily focuses on the average value of each segment and overlooks other critical features in time series. This limitation may lead to incorrect recognition of shape features in time series. In this paper, the first-order difference based SAX called DIFF-SAX and permutation entropy based SAX called PE-SAX are proposed as novel improved SAX methods to overcome the limitations of classic SAX. After that, we introduce dynamic time warping (DTW) algorithm into our improved SAX methods and put forward novel algorithms to measure the similarity between improved SAX representations. Subsequently, the core algorithms in this article, DTW-based DIFF-SAX (DIFF-SAX-DTW) and DTW based PE-SAX (PE-SAX-DTW), are proposed. Our proposed algorithms not only achieve dimension reduction but also overcome a main drawback of classic SAX: it is unable to accurately distinguish the time series with similar mean values. Additionally, the introduction of DTW algorithm makes it possible to achieve the “feature to feature” optimal warping alignment and measure the similarity between symbolic sequences with unequal length. Finally, by applying existing methods and our proposed similarity measurements to the classification problem of real-life datasets, the effectiveness and superiority of our proposed algorithms are demonstrated.
更多查看译文
关键词
Complex time-series,Similarity measure,Classification,Symbolic aggregate approximation,Dynamic time warping
AI 理解论文
溯源树
样例
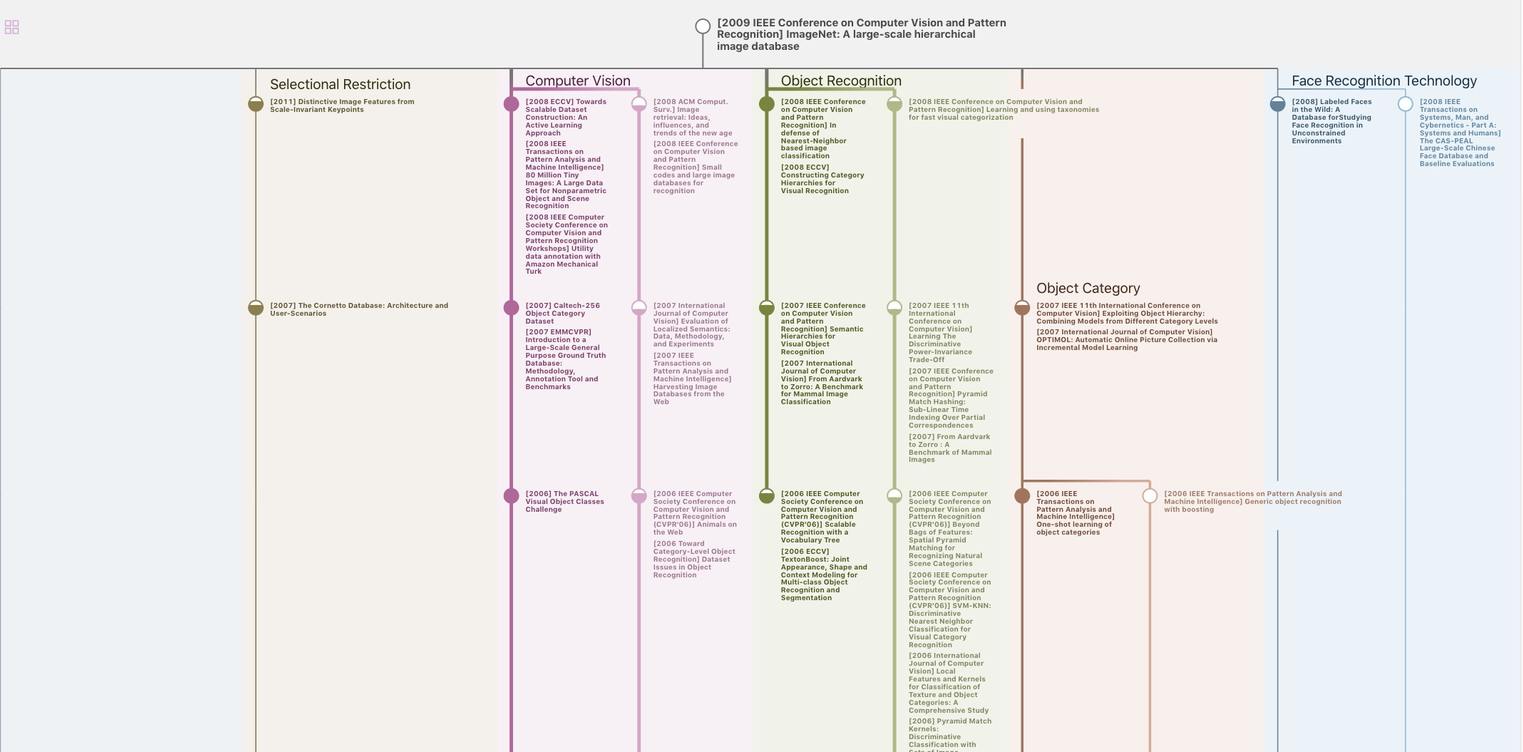
生成溯源树,研究论文发展脉络
Chat Paper
正在生成论文摘要