Generating Labeled Samples Based on Improved Cdcgan for Hyperspectral Data Augmentation: A Case Study of Drought Stress Identification of Strawberry Leaves
COMPUTERS AND ELECTRONICS IN AGRICULTURE(2024)
关键词
cDCGAN,Spectral generation,Data augmentation,Hyperspectral data,Drought stressed leaves
AI 理解论文
溯源树
样例
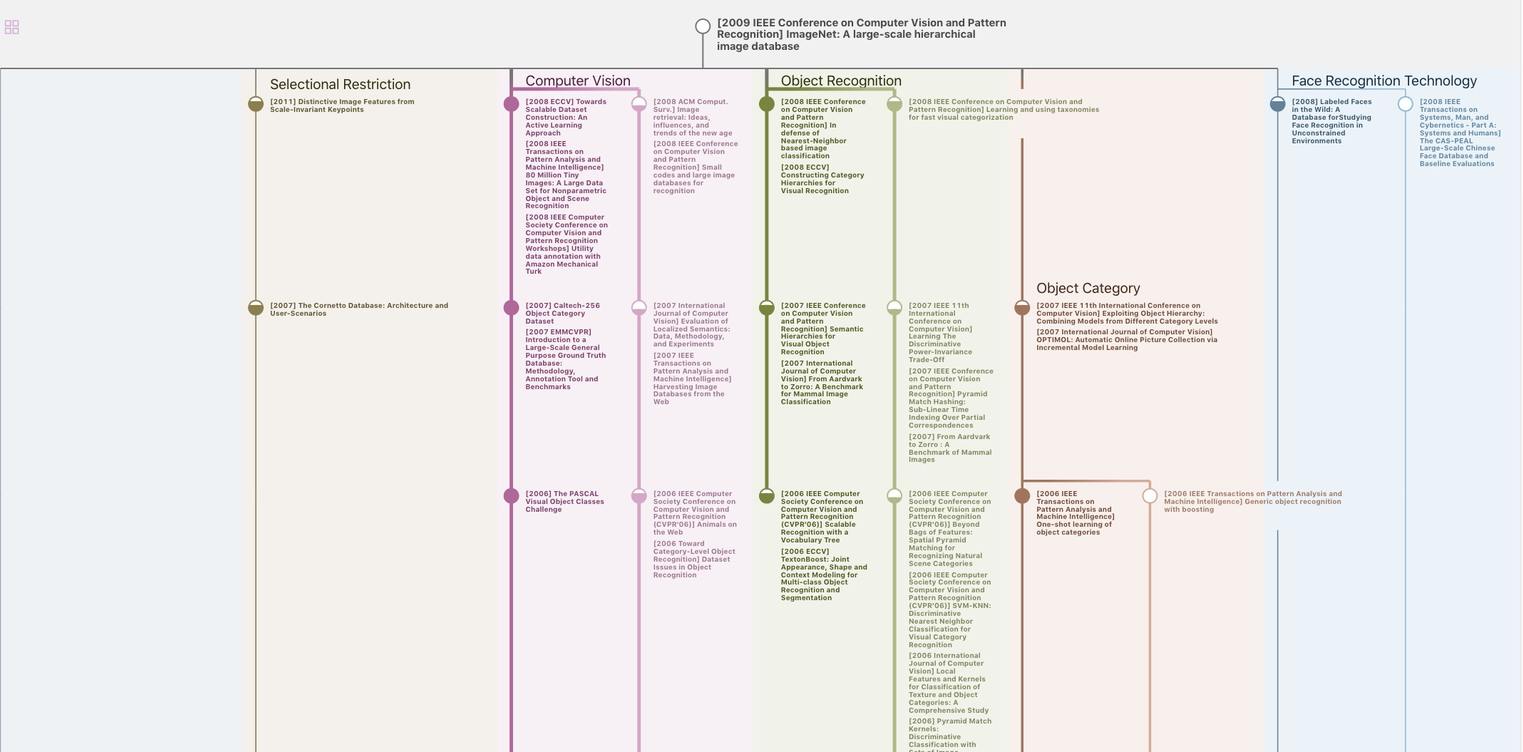
生成溯源树,研究论文发展脉络
Chat Paper
正在生成论文摘要