Convolutional Neural Network Advances in Demosaicing for Fluorescent Cancer Imaging with Color-Near-infrared Sensors
Journal of Biomedical Optics(2024)
Abstract
Significance:Single-chip imaging devices featuring vertically stacked photodiodes and pixelated spectral filters are advancing multi-dye imaging methods for cancer surgeries, though this innovation comes with a compromise in spatial resolution. To mitigate this drawback, we developed a deep convolutional neural network (CNN) aimed at demosaicing the color and near-infrared (NIR) channels, with its performance validated on both pre-clinical and clinical datasets. Aim:We introduce an optimized deep CNN designed for demosaicing both color and NIR images obtained using a hexachromatic imaging sensor. Approach:A residual CNN was fine-tuned and trained on a dataset of color images and subsequently assessed on a series of dual-channel, color, and NIR images to demonstrate its enhanced performance compared with traditional bilinear interpolation. Results:Our optimized CNN for demosaicing color and NIR images achieves a reduction in the mean square error by 37% for color and 40% for NIR, respectively, and enhances the structural dissimilarity index by 37% across both imaging modalities in pre-clinical data. In clinical datasets, the network improves the mean square error by 35% in color images and 42% in NIR images while enhancing the structural dissimilarity index by 39% in both imaging modalities. Conclusions:We showcase enhancements in image resolution for both color and NIR modalities through the use of an optimized CNN tailored for a hexachromatic image sensor. With the ongoing advancements in graphics card computational power, our approach delivers significant improvements in resolution that are feasible for real-time execution in surgical environments.
MoreTranslated text
Key words
near-infrared imaging,image-guided surgery,cancer surgery,bioinspired sensors,demosaicing,convolutional neural network
求助PDF
上传PDF
View via Publisher
AI Read Science
Must-Reading Tree
Example
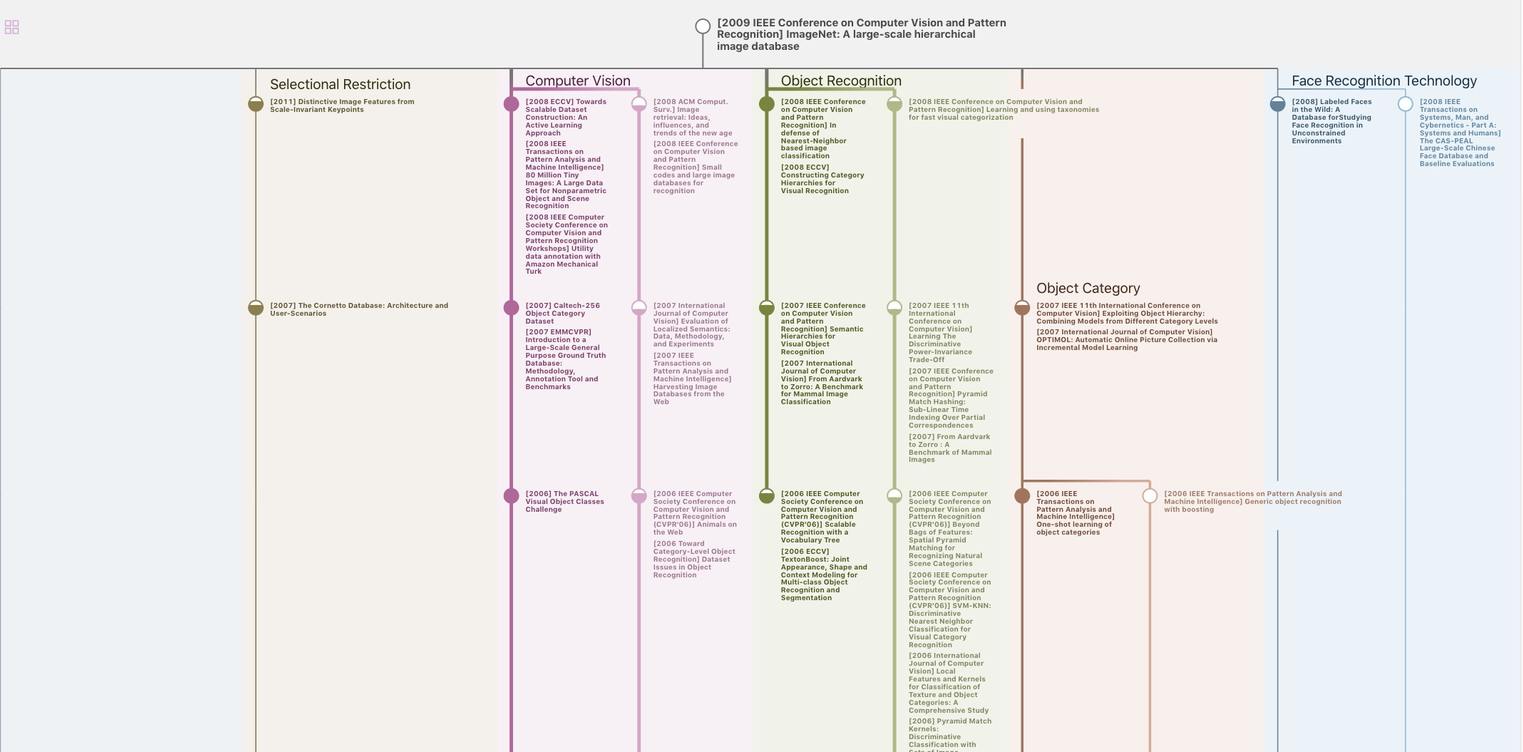
Generate MRT to find the research sequence of this paper
Data Disclaimer
The page data are from open Internet sources, cooperative publishers and automatic analysis results through AI technology. We do not make any commitments and guarantees for the validity, accuracy, correctness, reliability, completeness and timeliness of the page data. If you have any questions, please contact us by email: report@aminer.cn
Chat Paper
Summary is being generated by the instructions you defined